Data Driven Analysis: Insights for Strategic Decisions
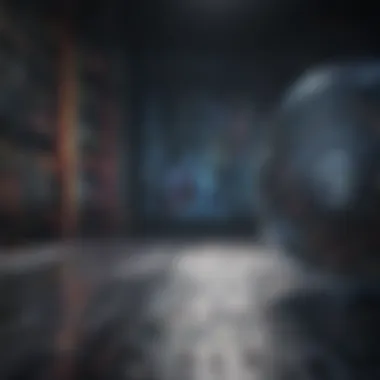
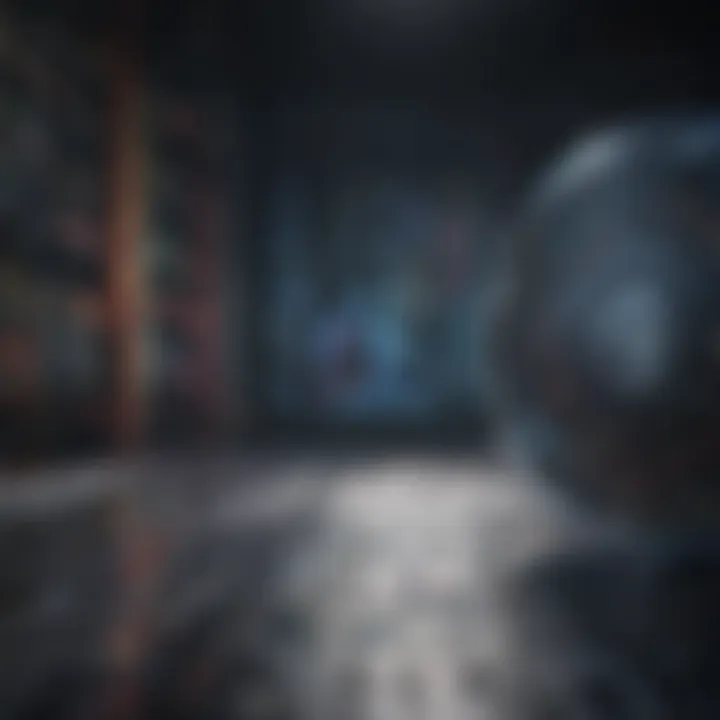
Intro
In the age of information, understanding how to properly sift through mountains of data is not just a skill; it’s a necessity. This necessity transcends mere academic discourse and seeps into the very fabric of corporate and scientific decision-making. Data-driven analysis, at its core, is the practice of utilizing data to inform conclusions and guide decisions. It’s an intricate dance between numbers and insights, a blend of methodology and instinct that showcases the power of informed choices.
As businesses and researchers alike dive deeper into this realm, the significance of harnessing accurate data becomes ever more pronounced. We live in an era where bad data can steer organizations in the wrong direction, leading to decisions that are less than optimal. Therefore, establishing a robust understanding of what data really means and how best to analyze it is crucial. In this exploration, we will dissect the foundational concepts guiding data analysis and untangle the complex web of current trends shaping the field.
Let’s begin our journey by breaking down the Key Concepts that drive data-driven analysis.
Intro to Data Driven Analysis
In today's fast-paced environment, data-driven analysis serves as the backbone of decision-making processes across several fields. It harnesses the power of data to unlock insights that can significantly enhance business strategies, scientific research, and public policy. By relying on a multitude of data sources, organizations can make informed choices, minimizing guesswork and maximizing outcomes.
Data-driven analysis is not just about having access to large amounts of information; it’s about transforming raw data into meaningful insights. These insights guide strategic planning and operational efficiency, making it crucial for professionals and researchers alike. In a world inundated with information, being data-savvy is no longer a luxury; it's a necessity.
Defining Data Driven Analysis
At its core, data-driven analysis refers to methodologies that emphasize using data to influence decisions. It integrates quantitative and qualitative data to establish patterns, predict outcomes, and support strategic choices. However, it's important to distinguish it from mere data collection and reporting. While collection aids in gathering facts, analysis interprets those facts, forming a narrative from numbers and trends.
The discipline encompasses several methodologies including, but not limited to, statistical analysis, machine learning techniques, and predictive analytics. Each methodology enables organizations to create models that can anticipate future trends, effectively translating insights into actionable strategies.
This reliance on solid empirical data results not only in better decision-making but also cultivates a culture of evidence-based practices. Decisions backed by data tend to be more reliable than those made through instinct or anecdotal evidence. As businesses face increasingly complex challenges, understanding the merits and means of data-driven analysis becomes paramount in distinguished sectors ranging from healthcare to finance.
"In the era of information, those who analyze better win the race."
"In the era of information, those who analyze better win the race."
The role of data in decision-making is both significant and multifaceted. With the advent of technology, more sophisticated methods are becoming available, leading to deeper insights and better outcomes. As we continue to explore the nuances of data-driven analysis throughout this article, we will uncover the methodologies, applications, and ethical considerations that define this vital field.
Historical Context of Data Analysis
The historical context of data analysis is crucial for understanding how we arrived at contemporary practices of making decisions based on insights gleaned from data. By examining the past, we can appreciate how analytical techniques have evolved and recognize the foundations upon which modern methodologies stand. Knowledge of this evolution not only positions us better in the field but also allows us to leverage past lessons to refine future analytical strategies.
The journey of data analysis can be traced back centuries, where the earliest anecdotes of data gathering date back to ancient civilizations, where societies collected census information for taxation or military purposes. These initial forays into data collection laid the groundwork for more structured approaches, leading us to the modern methodologies employed today.
Evolution of Analytical Techniques
Analytical techniques have undergone significant changes, and understanding this evolution reveals essential insights into contemporary data analysis. Initially, data was primarily observed and recorded manually, making computations cumbersome and prone to human error. Early forms of statistical analysis involved simple aggregations of numbers, which meant that insights were often limited and unclear.
As the Industrial Revolution took hold, a shift began. With the advent of the printing press and later the telegraph, data collection methods became more sophisticated, enabling faster and more accurate recording of information across various sectors. Heron of Alexandria's contributions in the first century, for instance, introduced early mathematical principles that would support these emerging techniques.
In the 20th century, the introduction of computers marked a turning point. The ability to process vast amounts of data in mere seconds transformed analytical capabilities. Statistical methods, sociological trends, and predictive modeling became central to how decisions were made. The development of methods like regression analysis and time series forecasting began to significantly contribute to various fields ranging from economics to healthcare.
Key Evolutionary Milestones:
- Manual Counting to Automated Data Processing: A fundamental change from slow, manual entries to fast automated systems.
- Introduction of Statistical Software: Tools like SPSS in the late 1960s democratized statistical analysis, allowing more professionals to wield data effectively without needing extensive mathematical backgrounds.
- Machine Learning and Big Data: The last two decades ushered in machine learning techniques that are common today. Algorithms can now learn from data and predict outcomes, marking a monumental leap in data analysis.
Understanding these historical contexts and the evolutionary steps taken not only illuminates the data analytics landscape but also emphasizes the significance of learning from what has come before. Today's analysts must grasp these principles to effectively wield data's power in decision-making processes.
"Without historical context, we could be adrift in a sea of data, unable to discern the meaningful patterns that drive our decisions."
"Without historical context, we could be adrift in a sea of data, unable to discern the meaningful patterns that drive our decisions."
Through this historical lens, we see that data analysis is not merely a modern fad, but a practice steeped in relevance and necessity, tightly woven into the fabric of societal advancement.
The Importance of Data Quality
Data quality is not merely a buzzword in the realm of data-driven analysis; it stands as the cornerstone upon which valuable insights are built. High-quality data can spell the difference between success and failure in decision-making for organizations, researchers, and educators alike. When data is accurate, clean, and reliable, it empowers entities to derive meaningful conclusions that can lead to innovative strategies and successful outcomes. However, when the underlying data is flawed, no amount of sophisticated analysis can salvage the insights drawn from it.
Focusing on data quality ensures that the conclusions drawn from data-driven analysis are substantiated and trustworthy. Characteristics such as accuracy, completeness, consistency, reliability, and relevance shape the quality of data. By maintaining high-quality standards, organizations can leverage their data to its fullest potential, ultimately enhancing efficiency and driving progress. Poor quality data can lead to misguided strategies, waste of resources, and missed opportunities, emphasizing the need for a stringent quality assurance process throughout the lifecycle of data management.
Ensuring Accuracy and Reliability
The foundation of any analytical endeavor lies in data accuracy and reliability. But what do these terms actually mean in practice? Accuracy refers to how close a given data point is to the true value, while reliability describes the consistency of data when measured multiple times.
To ensure accuracy, organizations often employ several methodologies, including:
- Regular audits of data sources: An inspection of both primary and secondary data sources helps to catch errors before they propagate through the analysis process.
- Validation checks during data input: Implementing robust checks at the time of data entry can prevent inaccuracies from being captured in the first place.
- Utilization of trusted tools: Tools that emphasize integrity and security can enhance the overall reliability of captured data.
"High-quality data is the bedrock of insightful analytics; without it, analysis is little more than educated guesswork."
"High-quality data is the bedrock of insightful analytics; without it, analysis is little more than educated guesswork."
Moreover, reliability comes into play by establishing a framework for collecting and processing data that can produce consistent results over time. This can include developing standard operating procedures for data collection and using consistent metrics when analyzing and presenting data findings. The emphasis on both accuracy and reliability cannot be overstated as they directly influence the credibility of the decisions made based on analyzed data.
Common Challenges in Data Quality
Despite the emphasis on data quality, organizations often face numerous obstacles that complicate its maintenance. Some challenges include:
- Diversity in data formats: When data is gathered from different sources, discrepancies in formatting can lead to difficulties in analysis. For instance, date formats may vary, which complicates comparison and trend analysis.
- Human error in data handling: Whether it’s inputting wrong values or misinterpreting data, human errors can significantly tarnish data quality. Even a single incorrect entry can skew results.
- Volume of data: The sheer amount of data generated today can overwhelm systems and personnel tasked with ensuring its quality. Mismanagement in handling large data sets can lead to overlooked errors or outdated information.
- Data retention policies: Inflexible policies on data retention may lead to the maintenance of outdated or irrelevant data, which can misdirect analytical efforts.
By acknowledging these challenges, organizations stand a better chance of developing efficient strategies to mitigate their impact. Addressing data quality is not a one-time fix but a continuous journey requiring diligence, learning, and adaptability.
Types of Data in Analysis
Understanding the types of data in analysis forms the backbone of effective data-driven decision-making. At its core, each category of data provides unique insights and perspectives that inform strategic choices across various fields such as business, healthcare, and education. The significance of distinguishing between these categories cannot be overstated; it allows analysts to employ the most suitable methodologies and tools for extracting valuable insights. This section will explore the two primary classifications of data, providing a detailed understanding of their implications in practical applications.
Quantitative vs Qualitative Data
Quantitative data pertains to information that can be quantified. It usually involves numbers and can be measured straightforwardly. Think sales figures, website traffic, or even the number of attendees at an event. This type of data allows for statistical analysis, enabling comparisons and drawing trends that are often represented through graphs, charts, or tables. For example, a company may analyze sales over a specific time period and conclude that their revenue has increased due to a marketing campaign.
Conversely, qualitative data is descriptive and tends to capture the characteristics of a phenomenon rather than measurable quantities. This might include customer feedback, interviews, or open-ended survey responses. Qualitative data shines in understanding human behavior, attitudes, and motivations. When a business gathers insights from customer reviews, they may discover underlying sentiments that aren’t readily quantifiable. This insight could guide product development or customer service improvements.
Using both types of data harmoniously offers a fuller picture. As the saying goes, "you can't see the forest for the trees"; hence, mixing the quantitative rigor with qualitative richness can lead to more informed and balanced decisions.
Structured vs Unstructured Data
Structured data is organized and easily searchable, typically stored in relational databases. This data adheres to a predefined model, making it straightforward to process and analyze. For instance, customer databases with fields like names, addresses, and transaction histories are structured. Organizations leverage structured data for quantitative analysis, facilitating tasks such as generating reports and deriving statistical insights.
On the other hand, unstructured data lacks a specific format or organization. This category includes vast amounts of data such as emails, videos, social media posts, or even transcripts from interviews. While unstructured data is often rich in context and insight, its chaotic nature makes it challenging to analyze systematically.
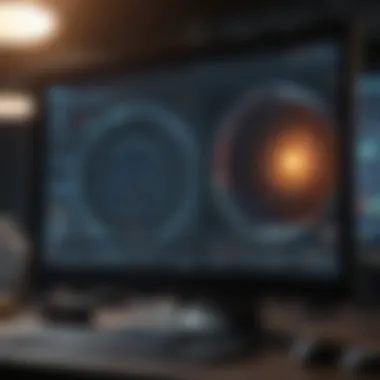
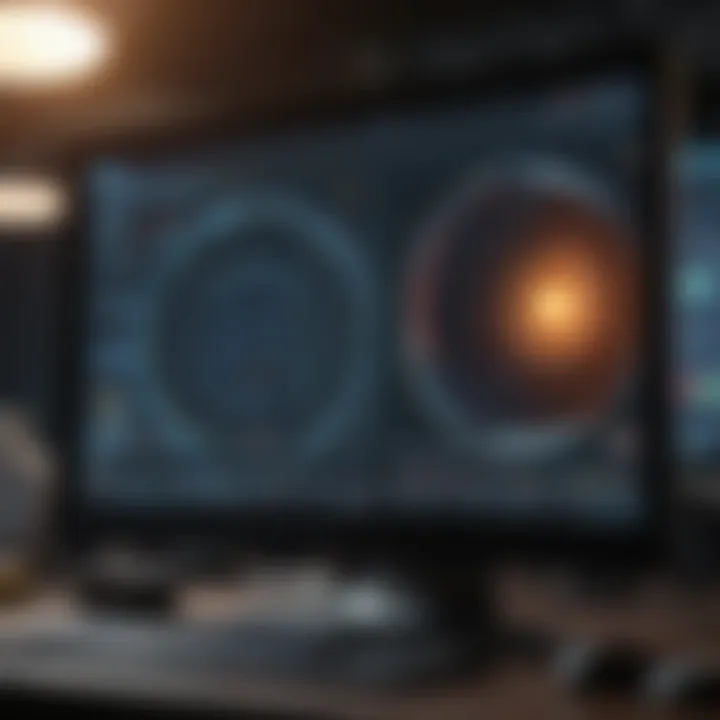
To illustrate, a news agency might collect vast amounts of unstructured data from social media regarding public sentiment on current events. The challenge lies in extracting meaningful insights from this complex landscape. Techniques such as natural language processing can help to turn this data into actionable insights, but it's not without its difficulties. As data analysts venture into the unstructured space, they need to employ innovative methodologies that can unravel the hidden narratives within this raw information.
In summary, understanding the nuances between quantitative and qualitative, as well as structured and unstructured data types is crucial in data-driven analysis. Each has its benefits and considerations, shaping how insights are derived and decisions made. Recognizing these differences empowers organizations to deploy the appropriate analytical strategies, enhancing their overall decision-making capabilities.
Methodologies in Data Driven Analysis
In the rapidly evolving landscape of data-driven analysis, methodologies form the backbone of effective decision-making processes. The various methods used not only define how data is interpreted but also dictate the strategic choices that organizations ultimately make. Understanding these methodologies thus becomes paramount.
The choice of methodology impacts a range of facets, from the accuracy of insights to the breadth of applications. Analytical methods can differ substantially in terms of complexity, execution time, and required expertise. Grasping these distinctions enables professionals to select the most suitable approach for their particular context. Here’s why methodologies play a crucial role:
- Clarity in Analysis: Different methodologies bring clarity and structure to the analysis process. They set out a roadmap to uncover insights, making complex data more digestible.
- Enhancement of Decision Quality: A robust methodology helps improve the quality of decisions significantly. By employing established techniques, data analytics can lead to more reliable outcomes.
- Scalability of Insights: Data methodologies also allow for scaling the insights generated. Techniques can be adapted for different sizes of datasets, making them versatile across various sectors.
Statistical Methods
Statistical methods serve as a linchpin in the realm of data analysis. They involve the application of statistical principles to analyze data, interpret patterns, and derive meaningful conclusions. Techniques such as regression analysis, hypothesis testing, and ANOVA are quintessential in discovering trends or determining relationships within data.
The importance of these methods lies in their ability to provide evidence-based insights. They help in quantifying uncertainties, allowing decision-makers to gauge risks effectively. Furthermore, statistical methods also facilitate:
- Validation of Data: They assess the validity of data sets, ensuring that conclusions drawn are based on sound interpretation rather than mere assumptions.
- Informed Strategic Planning: Organizations can set strategic priorities backed by data, enhancing the quality and effectiveness of their planning efforts.
Statistical methods not only serve as analytical tools but also shape the narrative around organizational performance and strategic goals.
Machine Learning Techniques
As we shift towards more complex analytical demands, machine learning techniques come into play. These algorithms take the reins of the analysis by learning from data, identifying patterns, and making predictions automatically. Machine learning has become increasingly essential due to its potential to process large volumes of data more efficiently than traditional methods.
The key advantages of machine learning in data analysis include:
- Increased Efficiency: By automating various analytical processes, resources can be redirected towards interpreting and applying insights rather than getting bogged down in data processing.
- Adaptability to New Data: These techniques can easily adapt to new data, continually improving accuracy and relevance in predictions.
- Complex Pattern Recognition: Unlike conventional statistical methods, machine learning can delve deeper into complex datasets to unearth patterns that may not be immediately obvious.
Through machine learning, organizations are better equipped to harness the power of their data, leading to more accurate forecasts and strategies built on real-time insights.
Predictive Analytics
Predictive analytics represents the apex of data-driven methodologies. It employs statistical algorithms and machine learning techniques to analyze past data for understanding future outcomes. By leveraging historical data, businesses can anticipate trends, identify potential challenges, and seize opportunities before they fully materialize.
The utilization of predictive analytics offers several compelling benefits:
- Proactive Problem-Solving: Organizations can address potential pitfalls beforehand, which turns reactive strategies into proactive ones.
- Resource Optimization: Through foresight provided by predictive analytics, businesses can optimize resources more effectively, reducing wastage and increasing ROI.
- Enhanced Customer Insights: Predictive models allow for better understanding of customer behavior, leading to refined marketing strategies and improved customer satisfaction.
In essence, predictive analytics encapsulates the future of data-driven decision-making, turning insights into actionable strategies for success.
"The right methodology not only generates insights but also inspires the actions that bring about change."
"The right methodology not only generates insights but also inspires the actions that bring about change."
These methodologies together create a comprehensive toolkit for analysts and decision-makers. In navigating today's data-rich environments, understanding and implementing these various methods can mean the difference between thriving in competition and merely surviving.
Tools and Technologies for Data Analysis
When navigating the landscape of data-driven analysis, understanding the tools and technologies available is paramount. These resources serve as the backbone for analysts and decision-makers alike, transforming raw data into actionable insights. In today’s fast-paced environment, having the right technology is not merely an advantage; it's a necessity.
Data analysis tools have the power to drastically improve efficiency, accuracy, and the overall decision-making process in any organization. For one, they simplify complex tasks, allowing teams to focus on interpreting data instead of getting bogged down by its intricacies. From data cleansing to visualization, the right software can greatly enhance productivity and outcomes.
Aside from mere functionality, these technologies also provide scale. As organizations grow and the volume of data expands, scalable solutions ensure that analytical capabilities keep pace with demands. Additionally, they often incorporate collaborative features, permitting teams across various departments to work together seamlessly on projects, breaking down silos that traditionally hinder fluidity in operations.
Popular Software Solutions
There’s a cornucopia of data analysis tools out there, each with its unique capabilities and strengths. Familiarity with some of the widely used software is crucial for professionals in the field. Here’s a brief overview of a few standout options:
- Tableau: This visualization tool is known for its ease of use, enabling users to create stunning, interactive representations of data without needing to write extensive code.
- Microsoft Power BI: Particularly favored by businesses that already utilize Microsoft products, Power BI integrates seamlessly into their ecosystems, allowing for real-time insights and robust reporting.
- R and Python: While not traditional software like the others, these programming languages are foundational in data analysis, used for statistical computing and machine learning tasks.
- SAS: This software provides advanced analytics, multivariate analysis, and business intelligence capabilities, commonly used in larger enterprises for complex predictive modeling.
These tools empower professionals to conduct thorough analyses, glean insights, and drive data-informed decisions with confidence.
Emerging Technologies in Data Analysis
The data analysis field is ever-evolving, with new technologies constantly emerging to reshape how data is handled and interpreted. Staying attuned to these advancements not only keeps analysts informed but can significantly influence an organization’s competitive edge. Some of the emerging technologies include:
- Artificial Intelligence (AI): AI is gradually taking the reins in data analysis through self-learning algorithms that improve accuracy over time, making predictions based on seemingly unrelated datasets.
- Big Data Technologies: Tools like Hadoop and Spark are designed to handle massive datasets, allowing businesses to analyze complex data patterns previously thought too intricate to decipher.
- Natural Language Processing (NLP): NLP enhances data analysis by allowing machines to understand, interpret, and contextualize human language, enabling insightful sentiment analysis from vast textual datasets.
With these technologies, organizations can harness data at a scale and speed previously unimagined, giving them an edge in their respective markets.
With these technologies, organizations can harness data at a scale and speed previously unimagined, giving them an edge in their respective markets.
Increasing familiarity with these tools and technologies is essential for professionals aiming to remain relevant in the rapidly changing world of data-driven analysis. Consequently, investing time in training and experimentation can profoundly influence future outcomes and breakthroughs.
Applications of Data Driven Analysis
In today’s fast-paced world, data-driven analysis plays a pivotal role across numerous sectors. As we navigate through immense volumes of data, understanding its applications becomes crucial. This concept transforms raw data into insightful information that guides strategic decisions, enhancing overall effectiveness and efficiency in various domains. Organizations harness these insights to streamline operations, improve customer satisfaction, innovate products, and ultimately boost their bottom line. The vast applications of such analysis serve not just companies, but also extend to fields like healthcare, education, and scientific research.
Case Studies in Business
The commercial world thrives on competition, and in this arena, being data savvy is no longer optional. Businesses that effectively utilize data analysis see substantial benefits. Case studies abound showcasing how organizations have adapted data-driven strategies to gain a competitive edge.
For instance, consider how Netflix analyzes viewing patterns. By examining massive datasets regarding user behavior, Netflix can tailor recommendations, significantly improving user experience and subscriber retention. This strategy doesn’t merely increase customer satisfaction; it drives profits as well, as more users stick around for the next binge-worthy show.
Moreover, companies like Amazon employ sophisticated algorithms to scrutinize customer preferences, which influences everything from marketing strategies to inventory management. They meticulously study purchasing trends, enabling them to make logistic decisions that enhance delivery efficiency. The cumulative effect of these insights not only optimizes operational functions but also tailors marketing efforts.
Insights derived from customer interactions significantly shape product offerings and pricing strategies, creating a cycle where both revenue and customer satisfaction rise.
Insights derived from customer interactions significantly shape product offerings and pricing strategies, creating a cycle where both revenue and customer satisfaction rise.
Scientific Research Dynamics
The realm of scientific research relies heavily on data-driven analysis. This methodology allows researchers to extract meaningful conclusions from complex datasets. In an age where research is increasingly collaborative and data-intensive, mastering these techniques is vital.
Take, for example, the field of genomics. Researchers harness data analysis to reveal intricate patterns within genetic information, leading to breakthroughs in personalized medicine. By analyzing large amounts of genomic data, scientists can identify specific genetic mutations that contribute to various diseases. This ensures treatments are tailored to individual patients, markedly improving prospects for effective care.
Another vivid example comes from climate science. Data-driven analysis gives researchers the ability to model climate trends, forecast future scenarios, and assess risks involved with climate change. Historic data, combined with current metrics, allows scientists to produce environmental models that guide policy decisions globally. This has enormous implications, as it impacts legislation, funding, and global cooperation efforts to combat climate change effectively.
Ethical Considerations in Data Analysis
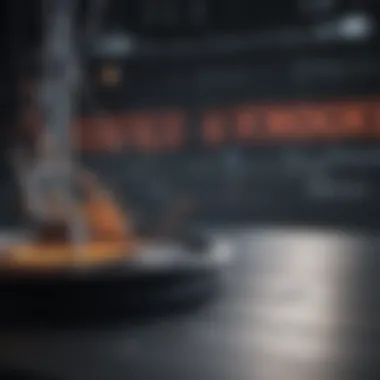
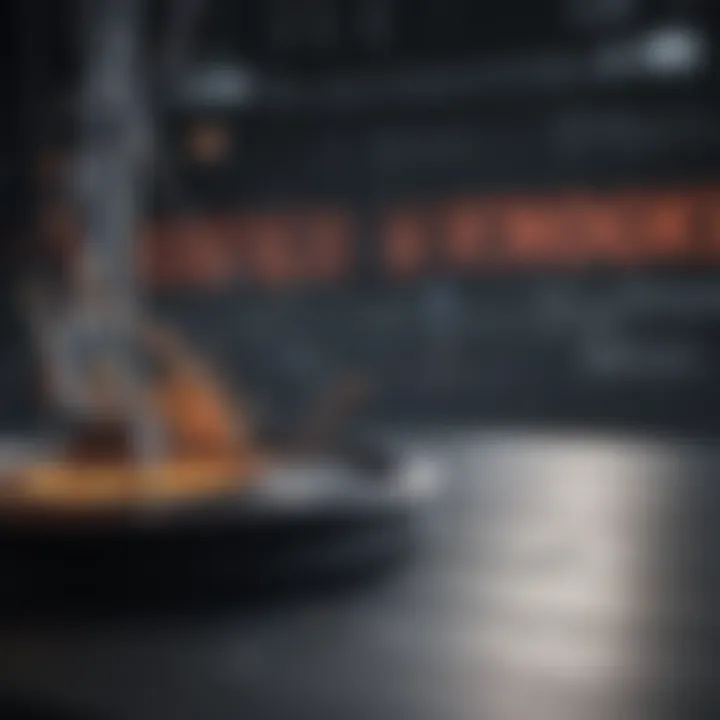
In today’s rapidly evolving landscape, ethical considerations in data analysis stand at the forefront of discussions surrounding responsible practice. This emphasis stems from the profound implications data can have on individual lives and society as a whole. Engaging with data involves not just technical routines; it also demands a view into the ethical landscapes that govern actions and policies linked to data use. These considerations aren't merely an afterthought or regulatory obligation; they ensure that organizations operate within frameworks that uphold societal trust and integrity.
Understanding ethical considerations, particularly in data-driven contexts, is pivotal for anyone—be it a student, researcher, or industry professional. It shapes how data is collected, analyzed, and ultimately utilized. This framework also contributes significantly to the credibility of findings and the efficacy of decisions made based on those findings.
Data Privacy Concerns
One of the most critical aspects of ethical considerations is data privacy. With the world pulsating with vast amounts of data, individuals’ rights to privacy are often at risk. Institutions that process personal data must ensure that there are strong safeguards in place.
Data privacy is particularly salient in sectors such as healthcare and finance, where personal information can lead to severe consequences if mishandled. For instance, a healthcare provider utilizing patient records for analysis needs to anonymize data to prevent identifiable information from being revealed. Furthermore, institutions should be transparent about how data is collected, processed, and shared. This can include:
- Prioritizing consent: Individuals deserve the right to give explicit permission before their data is used.
- Implementing limits on data access: Organizations should restrict who can access sensitive data.
- Establishing clear data retention policies: It’s crucial to determine how long data will be kept and under what circumstances it might be deleted.
Organizations often face scrutiny when their privacy practices fall short, which can have ramifications ranging from financial penalties to loss of public trust. Just take a look at recent data breaches in major corporations; such incidents illustrate how quickly a lack of attention to privacy can escalate into a crisis.
Bias and Fairness in Data Use
Another ethical consideration in data-driven analysis is bias. Bias can manifest in many ways, whether through the data collection process or the algorithms used to analyze that data. These biases often produce skewed results and can lead to unfair outcomes, especially for marginalized groups.
Data-driven decision-making should uphold fairness. For example:
- Diverse teams in data science can help identify and mitigate biases in datasets and algorithms.
- Regular audits of algorithms can be conducted to evaluate their impacts and ensure they don’t perpetuate existing inequalities.
- Inclusive policies should be crafted to reflect the values and needs of a broad spectrum of stakeholders.
Incorporating fairness into data analysis not only improves the accuracy of outcomes but also enhances trust in the decisions made. It's crucial for organizations to recognize that all data tells a story, and that story impacts real people.
"Ethical data practices are not just guidelines; they are essential foundations for sustainable and effective data-driven strategies."
"Ethical data practices are not just guidelines; they are essential foundations for sustainable and effective data-driven strategies."
In summary, the crux of ethical considerations in data analysis relies heavily on ensuring data privacy and addressing biases. As organizations and analysts delve deeper into the data-driven age, the ethical responsibility remains on their shoulders—cognizant that every number represents someone’s story.
Challenges Facing Data Driven Analysis
In today’s data-rich world, the implementation of data driven analysis isn't without its hurdles. Navigating through the vast landscapes of information can be tricky, and it’s crucial to understand these barriers to leverage data effectively. This section digs into the pivotal challenges that one encounters in data driven analysis, focusing on the ramifications these challenges can have on the quality of insights and decision-making processes.
Data Overload
The concept of data overload refers to the overwhelming amount of data available, which can hinder the ability to extract meaningful insights. When businesses or researchers are bombarded with data—whether it's from internal sources, social media platforms, or third-party vendors—it can feel akin to drinking from a fire hydrant. There are several key points to grasp about data overload:
- Information Paralysis: With numerous datasets vying for attention, decision-makers can struggle to discern which data points are relevant for their needs. This can delay or complicate crucial decision-making.
- Noise vs. Signal: In a sea of information, filtering out the noise can be challenging. Distinguishing between significant trends and mere fluctuations requires not just skill but also advanced analytical tools.
- Resource Allocation: Data overload often necessitates additional staffing or technology to manage it effectively, which can lead to increased operational costs.
"Too much information can be just as damaging as too little. It is essential to find a balance and focus on what truly matters."
"Too much information can be just as damaging as too little. It is essential to find a balance and focus on what truly matters."
Interpreting Data Results
Interpreting data results is perhaps one of the most critical aspects of data analysis that can make or break a business decision. While collecting data is relatively straightforward, transforming those raw numbers into actionable insights poses a significant challenge. The process involves several considerations:
- Contextual Understanding: Data doesn't exist in a vacuum. Without an understanding of the context behind the numbers, interpretations can easily lead to misguided conclusions. For example, seasonal sales may appear lower if viewed in isolation, but understanding the market trends and cycles can give a fuller picture.
- Statistical Literacy: A common hurdle many face is the ability to understand statistical significance and implications. Misinterpretation of statistical results can lead to decisions that are uninformed, potentially jeopardizing projects or strategies.
- Communication Skills: Even if the analysis is solid, the results must be communicated effectively to stakeholders. Data visualization tools can aid in presenting findings in digestible formats, but the narrative behind the numbers must still be compelling and clear.
In essence, while data driven analysis holds great promise for enhancing decision-making, the challenges of data overload and result interpretation cannot be understated. Being aware of these issues is the first step toward overcoming them, allowing decision-makers to cultivate a data savvy culture within their organizations.
Future Trends in Data Driven Analysis
As we navigate through the intricate world of data, it becomes increasingly clear that future trends in data driven analysis hold notable significance. Understanding these trends is crucial not only for professionals but for anyone looking to remain relevant in today's fast-paced, data-centric environment. This article seeks to outline the specific elements that underline these trends, the benefits they bring, and considerations that professionals must keep in mind.
Integration of AI and Data Science
The marriage between artificial intelligence and data science is quickly becoming one of the most notable trends within this realm. It's hard to throw a rock without hitting some chatter about AI these days. Whether it’s machine learning algorithms or neural networks, AI's role in data analysis is expanding significantly.
Benefits of AI Integration:
- Increased Efficiency: AI algorithms can sift through mountains of data much faster than a human can ever hope to. This means quicker decisions and a more streamlined process.
- Enhanced Accuracy: With AI's ability to detect patterns and anomalies, data accuracy is poised to improve. This reduces the likelihood of human errors that can skew results.
- Predictive Insights: AI offers the capability to forecast trends based on historical data, enabling proactive decision-making.
However, considerations must be taken into account. For instance, relying solely on AI can lead to overfitting, where models become too tailored to historical data and struggle to adapt to new trends. A harmony between human intuition and machine precision is essential for maximizing the potential of AI in data analysis.
Automating Data Insights
Automation is another trend gaining traction, as organizations strive to harness real-time data insights without the extensive labor that analysis typically entails. Automating the data insight process allows companies to stay agile and make informed decisions quicker.
Key Points for Automation of Data Insights:
- Real-time Data Processing: Automation enables businesses to work with data as it comes in rather than relying on periodic updates, promoting proactive responses to changes.
- Reduced Costs: Less manpower needed for analysis translates to significant savings. Fewer resources can be allocated elsewhere effectively.
- Scalability: Automated systems scale easily as your data grows, making them adaptable for business expansion without requiring proportional investment in human resources.
Again, while the trend towards automation sounds attractive, it’s vital to ensure that algorithms used in this automation are continuously monitored and evaluated. Data without context can lead to misguided conclusions, which can compromise the entire operation.
"Data is nothing without its voice. The future of analysis lies in how we empower that voice with intelligent automations and deep learning processes."
"Data is nothing without its voice. The future of analysis lies in how we empower that voice with intelligent automations and deep learning processes."
In summary, as AI integration and the automation of data insights continue to reshape data driven analysis, understanding these trends becomes crucial. Keeping a finger on the pulse of these changes will bolster capabilities, ensuring that professionals are equipped to face future challenges head-on, transforming raw data into actionable insights.
The Role of Visualization in Data Analysis
In an era where data flows like a river, the significance of visualization in data analysis can’t be understated. When interpreting complex datasets, it becomes imperative to ascertain not just the numbers but the stories they unfold. Good visualization transforms raw data into a format that is easy to digest, permitting deeper insights that might be veiled in spreadsheets filled with jumbled figures. The role of visualization entails not just presentation, but also communication of information effectively.
Key considerations emerge in this sphere. First, visualization serves to illuminate patterns, trends, and outliers that might otherwise slip through the cracks of detailed analysis. For instance, a bar graph representing yearly sales can quickly show fluctuations that hours of data work might overlook. Furthermore, capturing an audience's attention is easier with visuals—humans are inherently visual creatures. This pivot allows analysts to bridge comprehension between various stakeholders, simplifying jargon into understandable graphics.
The benefits extend beyond clarity. Effective visualization aids data-driven decision-making by facilitating comparative analysis, making it easier to juxtapose different datasets side-by-side. Just as a painter uses colors to create a striking image, data analysts and scientists employ visual techniques to unearth insights that can guide pivotal decisions.
"Data visualization is the part of data analysis that brings your findings alive."
"Data visualization is the part of data analysis that brings your findings alive."
Effective Data Representation Techniques
Choosing the right representation method plays a fundamental role in successful data visualization. Various techniques have proven effective, and using them judiciously can significantly enhance clarity. Here are some representation techniques:
- Bar Charts: Ideal for displaying discrete data, bar charts effectively illustrate comparisons, such as sales figures across different products.
- Line Graphs: A fundamental tool for tracking changes over time; they beautifully capture trends and fluctuations that might otherwise be obscured.
- Heat Maps: This technique illustrates data density. For example, mapping customer interactions across different regions can reveal hotspots of engagement.
- Pie Charts: Though often critiqued, pie charts can still provide a quick glance at relative proportions, such as market shares among competing companies.
It's crucial to remember that the choice of technique should align with the audience's needs and the data’s nature. Overly complex visuals can overwhelm, while oversimplified ones can mislead.
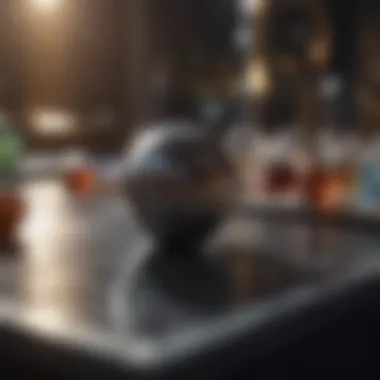
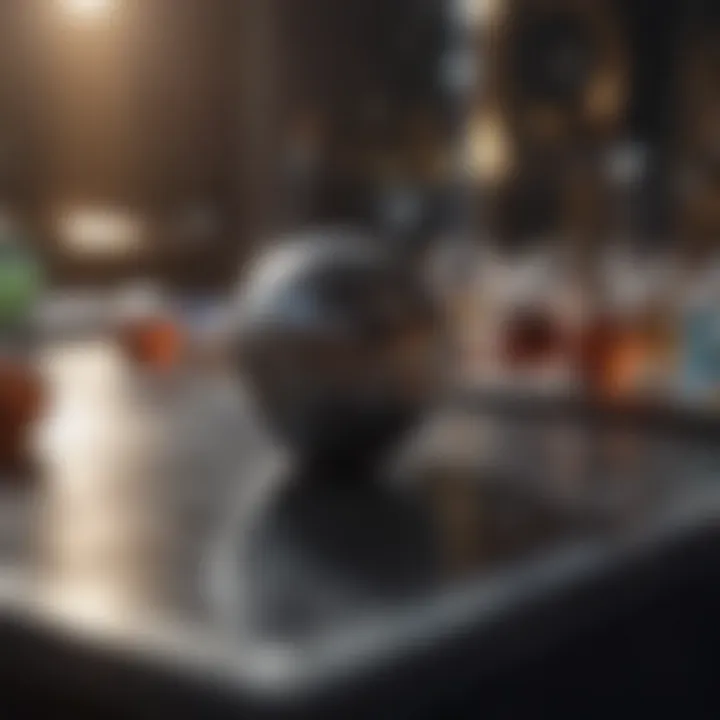
Tools for Data Visualization
The landscape of data visualization is dotted with numerous tools catering to diverse needs. These applications range from the sophisticated to the user-friendly, allowing professionals across fields to articulate insights more fluently. Several tools have gained prominence in this area:
- Tableau: Known for its interactive and shareable dashboards, Tableau facilitates intuitive exploration of data.
- Microsoft Power BI: This tool integrates seamlessly with Excel, empowering users to develop comprehensive reports with ease.
- D3.js: A powerful JavaScript library that allows for intricate and dynamic visualizations, perfect for web applications.
- Google Data Studio: This is a favorite among those seeking cost-effective solutions, enabling users to create custom reports and dashboards from various sources.
With continuing advancements in technology, the tools available are becoming increasingly sophisticated. Selecting the proper tool can drastically impact the effectiveness of your data storytelling.
Collaborative Approaches to Data Analysis
In today's fast-paced environment, one of the most compelling aspects of data driven analysis is the shift towards collaborative approaches. These methods not only enhance the quality of insight derived from data but also democratize the process of analysis. Effectively combining diverse viewpoints and skill sets, organizations can harness a broader spectrum of expertise. Bringing varied perspectives into analytical projects can lead to richer interpretations and solution-driven outcomes. Whether it’s in tech startups or established corporations, collaboration has become a focal point in elevating decision-making processes.
Interdisciplinary Frameworks
When it comes to collaboration, employing interdisciplinary frameworks can significantly enrich data analysis. These frameworks invite contributions from multiple fields, such as statistics, computer science, psychology, and domain-specific knowledge. For example, a healthcare initiative may involve statisticians to interpret data trends, doctors to contextualize findings, and ethicists to weigh the implications of data use in patient care.
By assembling teams with diverse expertise, organizations can:
- Broaden the scope of inquiry: Different fields provide unique lenses through which to analyze data, ensuring a well-rounded evaluation.
- Encourage creativity: Cross-pollination of ideas can lead to innovative approaches to problem-solving.
- Enhance relevance: Insights become more applicable to real-world challenges when viewed through the eyes of various disciplines.
This holistic approach not only paves the way for more informed decisions but also amplifies the potential for impactful outcomes. As each field contributes its unique methodologies, the collective analysis gains depth and precision.
Team Dynamics in Analytical Projects
Success in collaborative data driven analysis hinges on the team dynamics within analytical projects. The functioning of a team can make or break a data project, influencing everything from idea generation to the implementation of insights.
For effective team collaboration, consider the following:
- Clear Communication: Establish open lines of dialogue among team members to facilitate sharing of ideas and methodologies. Ensuring that everyone is on the same page can prevent misunderstandings and maintain focus on common goals.
- Diverse Skill Sets: Ensure that team members bring different skills to the table. Having a mix of data analysts, subject matter experts, and even software engineers can bolster the analytical process, allowing the team to tackle challenges more effectively.
- Fostering Trust: Building an environment where all voices are valued can lead to greater participation and enthusiasm. Team members who feel supported are more likely to share their insights and challenge the status quo.
"Collaboration is key; the more perspectives we invite, the stronger our analysis becomes."
"Collaboration is key; the more perspectives we invite, the stronger our analysis becomes."
Impact of Data Driven Analysis on Decision Making
In today’s fast-paced environment, organizations are inundated with data from all sides. From customer feedback to market trends, the sheer volume can be overwhelming. However, data driven analysis has become a cornerstone in modern decision making, transforming raw data into actionable insights. The importance of effectively leveraging this analysis cannot be understated. Not only does it provide a clearer picture of current situations, but it also equips decision-makers with the foresight necessary to anticipate future challenges and opportunities.
Transforming Business Strategies
One of the primary benefits of data driven analysis is its ability to refine and reshape business strategies. Companies that harness data effectively can pinpoint the exact needs of their customers, allowing for a tailored approach that elevates customer satisfaction. For instance, consider an online retailer that analyzes purchasing patterns. By understanding what products are frequently bought together, they can create more effective promotional bundles. This is not just guesswork; it's a calculated move informed by data. It paints a vivid picture of consumer behavior, and this knowledge turns mere strategies into effective, data-backed actions.
Moreover, data can reveal areas of inefficiency within an organization. By scrutinizing operational processes, companies can eliminate bottlenecks and save resources. A manufacturing firm that tracks production metrics might discover that a particular machine frequently breaks down during peak hours. Armed with this knowledge, managers can either upgrade equipment or adjust schedules. Therefore, data analysis doesn't merely react to current trends but enables proactive decision-making that can save both time and money.
Enhancing Research Outcomes
In the realm of research, data driven analysis provides a framework that enhances the validity and credibility of findings. Researchers are under constant pressure to deliver results that are not only accurate but also impactful. Using data analytics tools to dissect complex datasets allows for deeper insights, which can lead to groundbreaking conclusions. For example, in a healthcare study, analyzing patient data over years can reveal correlations and patterns that might not be evident through traditional methods.
Furthermore, the synthesis of varied data types—such as qualitative reports from patients and quantitative health metrics—yields a richer narrative. This enriched understanding helps in formulating hypotheses that are more aligned with real-world scenarios. As such, data driven analysis not only supports but actively enriches research outcomes, ensuring that findings are robust and reflective of true conditions.
"Realizing the full potential of data means not just seeing it, but understanding its implications deeply."
"Realizing the full potential of data means not just seeing it, but understanding its implications deeply."
Ultimately, the impact of data driven analysis on decision making is profound. In both business and research contexts, it facilitates informed strategies and fosters innovation. As we delve deeper into a data-centric world, those who incorporate data insights into their decision-making processes stand to gain a significant edge. The pathway forward is not just about collecting data but transforming it into invaluable insights that can shape the very future of organizations and research initiatives alike.
Data Literacy in the Modern World
In today’s fast-paced, digital landscape, data literacy has emerged as a cornerstone of effective decision-making. It encompasses not just the ability to read and interpret data but also to critically engage with it, challenge assumptions, and derive meaningful insights. As organizations increasingly rely on data to inform strategies and drive innovations, understanding the intricacies of data becomes imperative for a wide array of professionals.
The ability to make sense of data affects numerous areas, from shaping business strategies to enhancing educational frameworks. Without a strong foundation in data literacy, individuals risk becoming overwhelmed by the sheer volume of information available. This leads to potentially misguided decisions based on misinterpretations or selective data usage. Moreover, it can sideline important human elements, such as intuition and experience, in favor of blind reliance on data, which can be a slippery slope.
In essence, data literacy serves as a catalyst for improved critical thinking and analytical skills. This understanding is crucial for fostering a work environment where data-driven decision-making thrives.
The Need for Data Proficiency
Data proficiency is not merely a nice-to-have skill anymore; it’s a necessity across sectors. With advancements in technology, the accessibility of data has skyrocketed, giving rise to the increased demand for professionals who can navigate, analyze, and interpret this data effectively. Proficiency means being equipped to handle complex datasets, understand statistical methods, and engage with various analytical tools. This enables individuals to extract value from data and apply insights toward tangible outcomes.
- Improved Decision-Making: Being data literate allows professionals to make informed decisions rather than relying on gut feelings or outdated practices.
- Enhanced Career Opportunities: As the job market evolves, employers are searching for candidates with data skills—those who can demonstrate an ability to utilize data in strategic contexts.
- Facilitated Collaboration: In an environment where teamwork is essential, data proficiency helps disparate teams speak a common language about data, fostering better communication and collaboration across departments.
"In the future, data-savvy individuals will be indispensable to corporate strategies, shaping not just outcomes but also the narrative around data itself."
"In the future, data-savvy individuals will be indispensable to corporate strategies, shaping not just outcomes but also the narrative around data itself."
Educational Initiatives Promoting Data Skills
To meet the growing demand for data literacy, educational institutions and organizations are increasingly prioritizing initiatives aimed at enhancing data skills. These initiatives vary from formal educational programs to informal workshops and online courses, each tailored to meet the specific needs of different audiences.
- University Curricula: Many universities are now offering degree programs and certifications that focus on data science and analytics, preparing students for a career in the data-driven market.
- Workplace Training Programs: Corporates are investing in employee development through tailored training sessions that cover key aspects of data analysis, visualization, and interpretation.
- Online Learning Platforms: Websites like Coursera and edX provide accessible courses on data literacy, targeting a wide audience from beginners to advanced learners.
- Community Workshops: Local libraries and community centers increasingly host free workshops aimed at enhancing data skills among residents, ensuring inclusivity and access for all.
By fostering a culture of data literacy through these initiatives, society can build a robust backbone of knowledgeable individuals ready to transform insights into impactful decisions.
End and Future Outlook
In wrapping up the examination of data driven analysis, it becomes clear how this discipline is not merely a passing trend but a framework that has transformed how decisions are made across various sectors. The emphasis on data allows organizations to ground their strategies in empirical evidence. This isn’t just about big data or advanced analytics; it's about using the right data, effectively and responsibly, to drive meaningful insights that inform actions.
As we look ahead, several specific elements emerge as significant for the future of data driven analysis:
- Adaptability: Organizations must be prepared to adapt as new analytical tools and methodologies arise. This includes staying abreast of advancements in artificial intelligence and machine learning, which are likely to increasingly influence data strategies.
- Ethical Use of Data: With the wealth of information available, ethical considerations must be paramount. Ensuring that data collection and interpretation are fair and just will be critical as the regulatory landscape evolves.
- Skill Development: There will be a rising necessity for data literacy. As companies leverage analytics for decision-making, education systems will need to produce graduates capable of navigating this data-rich environment.
- Collaboration Across Disciplines: The future of data driven analysis will likely favor interdisciplinary approaches that can tackle complex problems effectively. This means that analysts will need to collaborate with domain experts, leading to a more nuanced understanding of data applications.
Ultimately, the integration of data driven approaches into decision making is set to enhance not only business strategies but also scientific advancement. As more professionals embrace this methodology, we can expect widespread improvements in outcomes across varied fields, affirming the impact that diligent analysis can have on our world.
Summary of Key Insights
Throughout this article, various key insights have emerged:
- Data driven analysis is vital in navigating the complexities of modern decision-making, providing organizations with actionable insights based on robust data.
- High-quality data is fundamental; without it, the reliability of analyses significantly diminishes, potentially leading to misguided decisions.
- Emerging tools and technologies continue to revolutionize how data is analyzed and interpreted, expanding the possibilities for organizations.
- Ethical considerations in data usage cannot be overlooked as they are crucial for maintaining public trust and compliance with regulations.
- Future trends point toward the increasing importance of data literacy and collaboration among various disciplines, fostering environments where cutting-edge analysis can thrive.
As organizations continue to adapt to a rapidly changing landscape, the insights drawn from data become even more essential in crafting resilient, innovative strategies.
The Path Forward for Data Driven Approaches
Looking forward, several paths can be outlined for advancing data driven approaches:
- Innovative Training Programs: Companies and educational institutions should invest in creating innovative training programs that not only teach data analysis skills but also cover ethics and the practical application of data in decision-making thus developing a skilled workforce.
- Enhanced Data Visualization: As syntactic complexity in data sets increases, the need for sophisticated data visualization tools that make information digestible for a wider audience will be essential. Clarity here drives improved comprehension and decision-making.
- Integration of AI Tools: The integration of AI-driven tools can streamline data analysis processes, enabling predictability and efficiency—this will be critical for organizations aiming to stay competitive in their respective fields.
- Stronger Data Policies: Establishing comprehensive data governance policies will ensure that organizations handle data responsibly, mitigating risks associated with privacy concerns and ethical dilemmas.
- Community Engagement: Engaging with the public in data initiatives can foster trust and transparency, encouraging collaboration and benefiting from diverse perspectives that enrich the analysis.
Taking these steps will not only solidify the roles of data and analysis in decision-making but also pave the way for more equitable, informed, and efficient operational frameworks.