Fraud Detection Techniques: An In-Depth Exploration
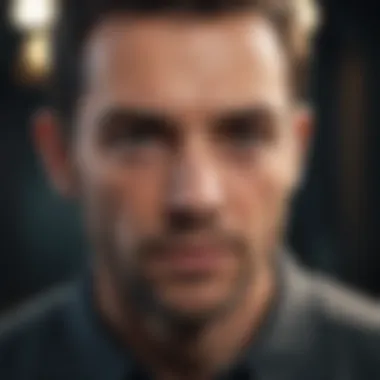
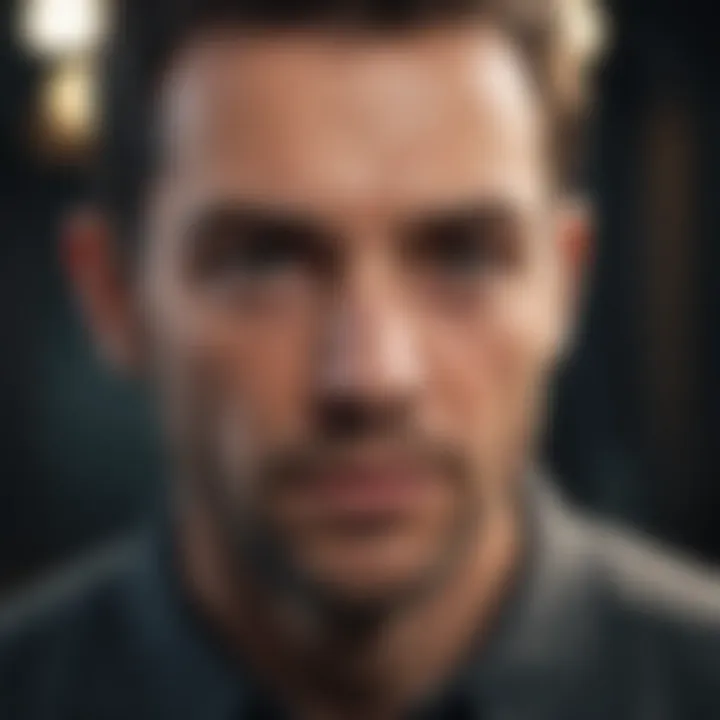
Intro
Understanding fraud detection techniques is crucial in today’s rapidly evolving digital landscape. Fraud impacts various sectors including finance, healthcare, and e-commerce. Detecting fraudulent activity can save organizations significant amounts of money and protect their reputations. This article examines the different methods and technologies available to combat fraud, offering insights into both traditional approaches and advanced technological developments.
Key Concepts
Definition of the Main Idea
Fraud detection refers to the processes and techniques used to identify fraudulent activities. It involves analyzing data to uncover patterns and irregularities that may indicate deception. With the growth of technology, fraud detection has become more sophisticated. Organizations now use a blend of human insight and automated systems to increase the accuracy and speed of detection.
Overview of Scientific Principles
Several scientific principles guide fraud detection techniques. Data analysis plays a central role, utilizing statistical methods and algorithms. For instance, anomaly detection algorithms help identify that activity deviates from normal patterns. Machine learning is increasingly prominent, enabling systems to learn from data and improve their accuracy over time.
Current Research Trends
Recent Studies and Findings
Recent studies have highlighted the effectiveness of machine learning in identifying fraudulent transactions. Researchers found that models like random forests and neural networks outperform traditional rule-based systems. These studies underline the importance of adapting to evolving fraud tactics through continuous research and innovation.
Significant Breakthroughs in the Field
A key breakthrough is the integration of real-time analytics in fraud detection systems. This advancement allows organizations to respond to suspected fraud instantaneously. Companies are leveraging big data analytics and artificial intelligence to monitor transactions, enhancing the capability to detect fraud as it occurs.
The future of fraud detection lies in the integration of advanced technology and innovative methodologies, continuously evolving to address the complex landscape of fraud.
The future of fraud detection lies in the integration of advanced technology and innovative methodologies, continuously evolving to address the complex landscape of fraud.
By understanding these key concepts and current research trends, one can appreciate the importance of effective fraud detection mechanisms in safeguarding businesses and consumers alike.
Understanding Fraud
Fraud represents a significant threat across various sectors. Understanding what constitutes fraud is essential for developing effective detection methods. By grasping the nuances of fraudulent activities, organizations can better anticipate risks and devise strategies to combat them. This section will delve into the fundamental aspects of fraud, focusing on its definition and the diverse forms it takes. The knowledge garnered here forms the basis for the subsequent exploration of detection techniques.
Definition of Fraud
Fraud can be classified as an intentional deceiving act meant to secure an unfair or unlawful gain. It typically involves misrepresentation, concealment of facts, or distortion of truth, leading victims to mistakenly believe in the legitimacy of a situation or entity. This deceit can manifest in various contexts, including financial transactions, identity claims, and digital environments. A precise understanding of fraud is vital for any organization aiming to develop a robust fraud detection framework. It enables proactive measures, enhancing overall security and trust within corresponding systems.
Types of Fraud
Fraud can be categorized into several distinct types, each presenting unique challenges and risks. Below are some notable forms:
Financial Fraud
Financial fraud encompasses a broad spectrum of dishonest acts aimed at securing monetary gain through deceit. This category includes investment scams, Ponzi schemes, and accounting misrepresentations. Its key characteristic is the perpetrator's manipulation of financial data or principles, exploiting grey areas for personal benefit. Within this article, financial fraud is emphasized due to its widespread occurrence and the substantial economic impact it can have.
The unique feature of finacial fraud lies in its ability to affect investors and institutions simultaneously, leading to devastating results for both sides. The advantages of identifying and addressing this type of fraud early can prevent significant losses and restore confidence.
Identity Theft
Identity theft occurs when an individual unlawfully uses someone else's personal data. This often includes the hijacking of social security numbers, bank account credentials, and credit card information. The primary characteristic of identity theft is the unauthorized appropriation of personal identifiers, which typically leads to financial catastrophe or reputational harm for the victim. This article focuses on identity theft due to its growing prevalence in a digitalized world.
Unique to identity theft is its potential for pervasive damage, extending far beyond financial losses, as victims may face long-term consequences in terms of credit ratings and psychological stress. The need for rigorous detection mechanisms for identity theft is paramount.
Insurance Fraud
Insurance fraud involves deceiving an insurer to receive undeserved benefits or funds. Common examples include false claims for damages or exaggerating losses. The defining feature of insurance fraud is its dual impact: it drives up costs for policyholders while diminishing the integrity of the insurance industry. This article highlights insurance fraud as a vital topic as it demonstrates the critical nature of accurate claims processing and risk assessment.
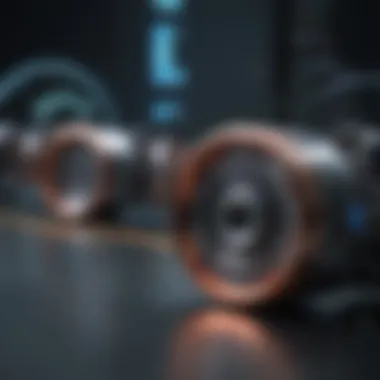
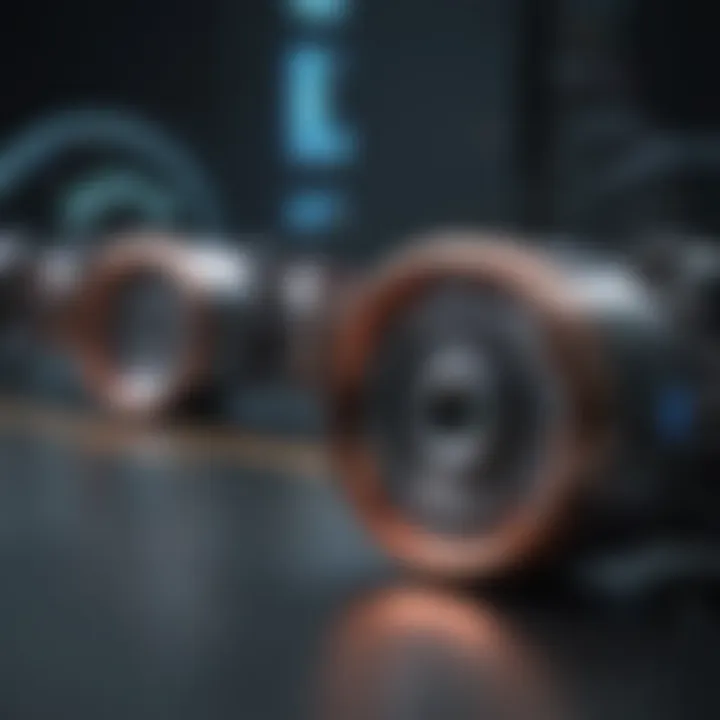
What makes insurance fraud particularly noteworthy is the sophisticated tactics often used to perpetrate it. Consequently, understanding and preventing this fraud is essential for maintaining industry credibility and protecting consumer interests.
Cyber Fraud
Cyber fraud is a modern form that targets online transactions and personal information. It includes phishing scams, online auction fraud, and various cybercrimes performed over the internet. The central attribute of cyber fraud is its reliance on technology and the anonymity it provides to criminals. This article includes cyber fraud as a focal point, as it exemplifies the transformation of fraudulent tactics in our increasingly digital landscape.
A significant feature of cyber fraud is its constantly evolving nature. Cybercriminals adapt quickly to new security measures, which poses ongoing challenges for detection methods. Addressing this challenge effectively requires adaptable and advanced technological solutions.
Importance of Fraud Detection
Fraud detection holds critical significance in today’s complex financial landscape. Understanding the myriad of ways fraud can impact organizations and individuals alike is paramount. In this urgency-driven environment, where criminal tactics are constantly evolving, robust fraud detection mechanisms are not merely beneficial; they are essential for protecting assets and maintaining trust.
Economic Impact
The economic repercussions of fraud can be staggering. According to the Association of Certified Fraud Examiners, organizations lose an estimated five percent of their revenue to fraud annually. This statistic underscores the necessity of effective fraud detection strategies. Businesses can potentially save millions by identifying and tackling fraudulent activities promptly. Furthermore, fraud leads to increased operational costs and can adversely affect cash flow, which is crucial for any organization's survival.
The ripple effect of fraud extends beyond individual organizations. It can undermine entire sectors and result in increased prices for consumers. When companies face losses from fraud, they often pass these costs onto their clients, leading to a rise in prices for goods and services.
Reputation Management
Trust is a cornerstone of any successful business. When fraud occurs, it can severely damage a company's reputation. Rebuilding trust after a fraud incident requires significant time and resources. Customers expect transparency and reliability, and when this is compromised, they may choose to take their business elsewhere.
Effective fraud detection helps safeguard an organization’s reputation. Companies that demonstrate a commitment to protecting their clients and stakeholders against fraud are more likely to retain customer loyalty. Moreover, a strong reputation in fraud prevention can serve as a unique selling proposition in competitive markets, attracting clients who prioritize security.
Regulatory Compliance
In many industries, adherence to regulations regarding fraud prevention is not just advisable; it is obligatory. Governments across the globe have established various regulations that necessitate rigorous fraud detection protocols. For instance, financial institutions are required to implement Anti-Money Laundering measures and comply with Know Your Customer regulations. Noncompliance can lead to severe penalties, including substantial fines and even loss of business licenses.
Maintaining compliance with regulatory requirements is vital. It not only protects companies from legal consequences but also fosters a culture of integrity and accountability. Organizations effectively using fraud detection tools can ensure they meet the necessary standards while achieving operational efficiencies.
"Fraud detection is not just about catching fraud; it's about creating an environment where fraud cannot thrive."
"Fraud detection is not just about catching fraud; it's about creating an environment where fraud cannot thrive."
Traditional Fraud Detection Techniques
In the realm of fraud detection, traditional techniques lay the groundwork for understanding and combating deceitful actions. Though newer technologies have emerged, these classic methods remain significant. They provide a framework and context within which modern advancements operate. Understanding these techniques is critical for comprehending the evolution of fraud prevention.
Manual Auditing
Manual auditing involves the thorough examination of financial records and transactions by trained professionals. This technique is crucial for identifying discrepancies that automated systems might overlook. Auditors evaluate patterns in financial behaviors and pinpoint irregularities. They can track transactions back to their origins, ensuring a higher degree of accuracy.
The effectiveness of manual auditing varies depending on the auditor's expertise and the methods used. This limitation can result in significant gaps—particularly in large organizations with extensive data. However, when combined with modern techniques, such as data analysis, manual audits can provide in-depth insights. Understanding this balance is key for organizations that aim to combat fraud effectively.
Anomaly Detection Methods
Anomaly detection focuses on identifying deviations from expected behavior within datasets. These deviations may signal fraudulent activities. Common methods of anomaly detection include statistical analyses and clustering techniques. For instance, organizations might analyze their historical transaction data to establish a baseline of normal behavior. Once this baseline is established, any transaction that significantly deviates from that norm is flagged for further investigation.
The strength of anomaly detection lies in its ability to spot incidents before they escalate. However, it requires high-quality data and considerable effort to minimize false positives. Organizations must be careful not to overlook genuine transactions due to overly stringent anomaly definitions.
Know Your Customer (KYC)
Know Your Customer (KYC) is a process established to ensure that businesses identify their clients. This technique is particularly prevalent in the financial industry. It aims to prevent fraud by confirming customer identities through documentation and background checks. By establishing a clear picture of who the customer is, organizations can better detect suspicious activities.
The importance of KYC cannot be overstated. It not only helps prevent financial crime but also builds trust in the financial system. However, maintaining compliance with KYC regulations can be a challenge, as requirements often change. Organizations must stay updated and adapt their practices accordingly.
In summary, traditional fraud detection techniques, while facing modern challenges, form an essential part of a robust fraud prevention strategy. A combination of manual efforts, anomaly detection, and thorough customer verification can enhance an organization's ability to combat fraudulent activities. With the proper integration of these techniques, businesses can create a more secure financial environment.
Modern Fraud Detection Techniques
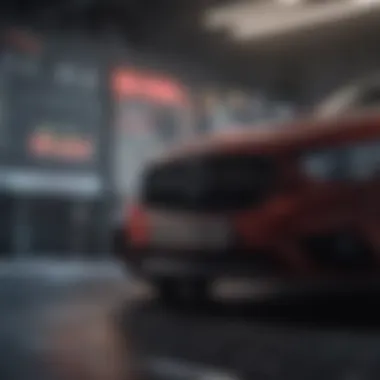
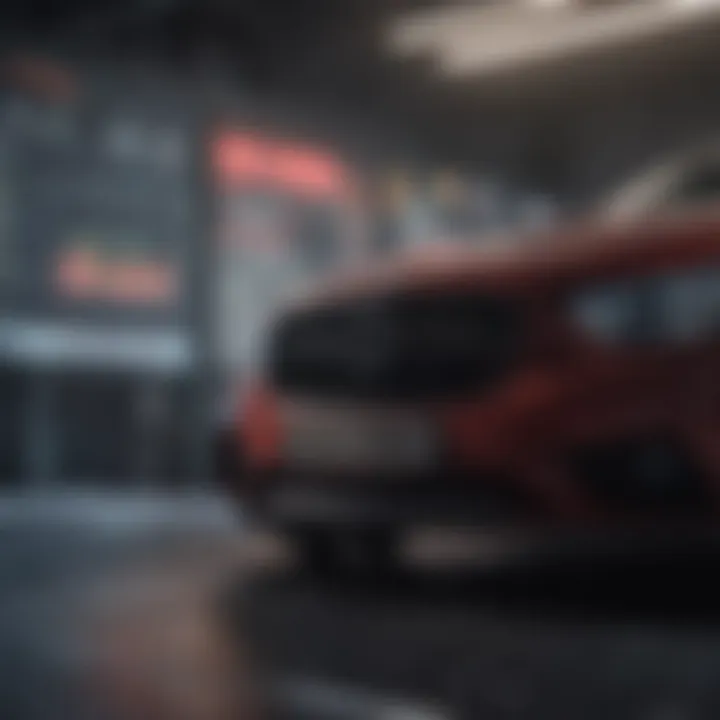
Modern fraud detection techniques leverage advanced technology to tackle increasingly complex fraudulent activities. This shift from traditional methods is essential in our digital age, where fraudsters are becoming more sophisticated. By employing these modern techniques, organizations can enhance their ability to identify and respond to fraud effectively.
Data Analytics and Mining
Data analytics and mining play a crucial role in modern fraud detection. This process involves analyzing large volumes of data to discover patterns and anomalies that may signify fraudulent behavior. The application of these techniques allows organizations to sift through massive datasets quickly, revealing insights that manual methods might miss.
- Efficiency in identifying threats: Analytics not only speeds up the fraud detection process but also increases accuracy. By using historical data, organizations can develop models that help predict potential fraud.
- Dynamic adaptation: Unlike static methods, data analytics can adapt to new fraud trends by constantly learning from new data inputs. This ensures that organizations remain one step ahead of fraudsters.
Machine Learning Algorithms
Machine learning is a pivotal component of modern fraud detection. It utilizes algorithms to analyze data, classify it, and make predictions. This section explores two main types of machine learning used in fraud detection: supervised and unsupervised learning.
Supervised Learning
Supervised learning is characterized by the use of labeled data to train models. This method is particularly potent in detecting known types of fraud. The model learns from historical data, making it capable of identifying similar patterns in new data.
- High precision: Supervised learning often leads to high accuracy in fraud prediction, as the model is trained on specific examples of fraudulent activities.
- Customized solutions: Organizations can tailor models based on their unique data characteristic and the types of fraud they most frequently encounter. However, a limitation is that it requires a significant amount of labeled data to be effective.
Unsupervised Learning
Unsupervised learning, on the other hand, involves training algorithms on data without predefined labels. This approach helps identify unknown patterns or anomalies in transactional data.
- Discovering hidden fraud patterns: It is beneficial for detecting novel fraud tactics that have not been encountered before. By identifying outliers, unsupervised learning can highlight suspicious activities that may require further investigation.
- Less data dependence: One of the advantages is that it does not need extensive labeled datasets like its supervised counterpart. However, the challenge lies in interpreting the results, as there might be a risk of false positives.
Artificial Intelligence Applications
Artificial intelligence (AI) applications in fraud detection encompass various technologies that enhance the detection process. AI integrates both machine learning and rule-based systems, providing a comprehensive approach to fraud prevention.
- Real-time monitoring: AI systems can analyze transactions in real-time, flagging suspicious ones for immediate review.
- Enhanced decision-making: AI systems also assist in making informed decisions by synthesizing data from multiple sources, identifying trends that human analysts may overlook.
Behavioral Analytics
Behavioral analytics plays a crucial role in modern fraud detection methodologies. It focuses on understanding how individuals act in various situations, particularly in the context of financial transactions and interactions. The insights gained from this analysis can significantly enhance security measures across different platforms.
Understanding Customer Behavior
To effectively combat fraud, organizations must first comprehend customer behavior. This involves using advanced data analytics to observe and interpret the behavior of legitimate users. An understanding of normal activities enables detection of potential threats. Specific patterns, such as typical transaction sizes, frequencies, and locations, can be established through historical data. By identifying these parameters, companies can create a baseline that assists in spotting deviations.
Moreover, various factors contribute to customer behavior, including demographic data, purchasing history, and online activity. Integrating data from multiple sources enriches the analysis. For instance, a sudden increase in transaction amounts or changes in purchasing patterns may indicate fraudulent activity, warranting further investigation. Understanding customer motivations, decision-making processes, and the context surrounding their actions is essential for creating effective fraud detection systems.
Detection of Unusual Patterns
Once customer behavior is mapped, organizations can then focus on the detection of unusual patterns. This step is vital as it highlights discrepancies that deviate from normal behavior. By leveraging statistical methods and machine learning, unusual patterns can be flagged in real-time. Patterns of concern might include:
- Transactions occurring at odd hours
- Multiple purchases from diverse locations within a short timeframe
- Rapid alterations in shipping addresses
Detection algorithms can analyze vast amounts of data, pulling critical variables to identify unexpected behaviors that could indicate fraud. For example, if a customer who usually shops in one country suddenly makes multiple purchases from a different country, this anomaly could trigger alerts.
Behavioral analytics thus contributes to a more refined and responsive fraud detection approach, allowing organizations to act swiftly and effectively in mitigating risks. The ongoing evolution in customer behavior offers an ever-expanding dataset for analysts, ensuring that fraud detection remains an adaptive process.
Role of Blockchain in Fraud Prevention
The integration of blockchain technology plays a pivotal role in enhancing fraud prevention strategies. Due to its unique characteristics, such as immutability and decentralized design, blockchain creates a more robust environment for verifying transactions. In this section, we will examine the specific elements that highlight how blockchain contributes to combating fraud, its benefits, and some considerations that arise from its implementation.
Transparency and Traceability
One of the primary advantages of blockchain technology is its transparency. Every transaction recorded on a blockchain is visible to all participants, which fosters an environment of accountability. This openness discourages fraudulent actions, as potential wrongdoers know that their actions are under scrutiny. Furthermore, because every transaction is timestamped and linked to previous transactions, traceability becomes a key asset.
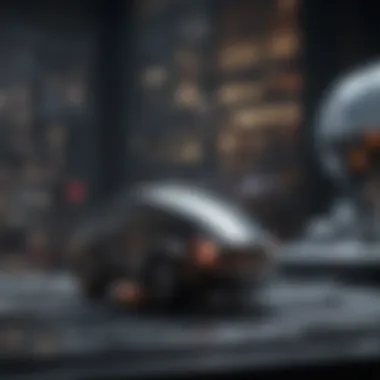
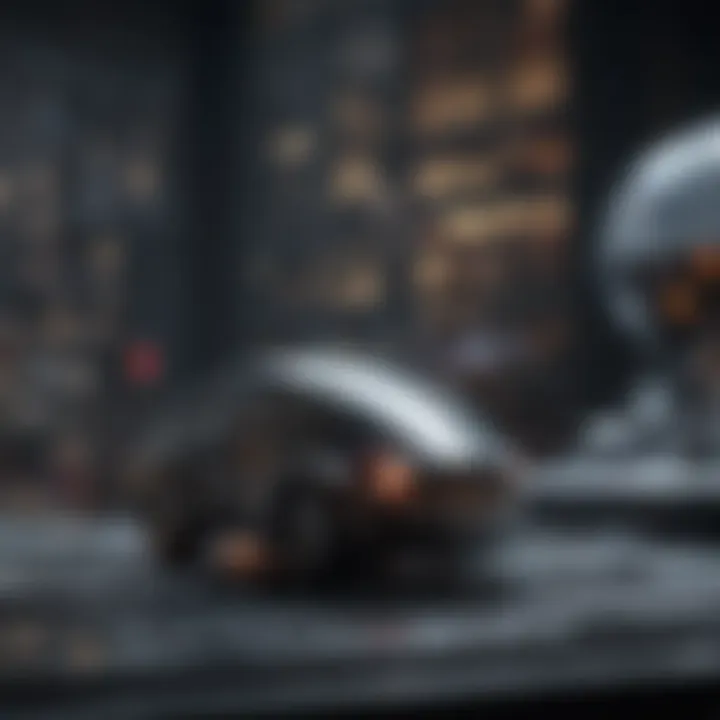
"In a blockchain environment, participants can trace every action back to its origin, making it remarkably difficult to falsify records."
"In a blockchain environment, participants can trace every action back to its origin, making it remarkably difficult to falsify records."
This feature becomes especially useful in industries such as supply chain management, where tracking the provenance of goods can help identify fraud quickly. In financial services, for instance, suspicious transactions can be easily monitored and traced back to their origins, mitigating risks associated with money laundering and unauthorized financial activities.
Smart Contracts
Another critical component of blockchain's role in fraud prevention is the use of smart contracts. These are self-executing contracts with the terms of the agreement directly written into code. When conditions are met, the contract automatically executes the agreed actions. This helps to eliminate the need for intermediaries, reducing the chances of manipulation.
Smart contracts ensure that all parties involved adhere to the stipulated terms, automatically enforcing compliance. This functionality not only minimizes the potential for fraud but also enhances efficiency. For example, in real estate transactions, a smart contract can automatically transfer property ownership once payment is confirmed, reducing delays and opportunities for fraudulent behavior.
Challenges in Fraud Detection
Understanding the challenges in fraud detection offers critical insights into the complexities involved in preventing fraudulent activities. As fraudsters continuously evolve their tactics, the importance of adapting detection methods cannot be overstated. In an increasingly digital landscape, organizations face pressing issues that hinder their ability to combat fraud effectively.
Evolving Fraud Tactics
The first significant challenge is the rapidly changing tactics that fraudsters employ. Traditional methods often prove insufficient as criminals develop more sophisticated and elusive strategies. For instance, techniques such as identity theft have become more intricate, leveraging stolen personal information in ways that can evade standard detection systems. Fraudsters are also using technology to their advantage, utilizing deepfake software, phishing schemes, and social engineering. Organizations must stay ahead of these trends, investing in ongoing training and the latest technology to understand and address these evolving threats.
Data Scarcity and Quality Issues
Another formidable challenge is data scarcity and quality issues. Effective fraud detection depends on having comprehensive and accurate data. However, many organizations struggle with fragmented data sources, making it difficult to obtain a unified view of customer behaviors and transaction patterns. Additionally, poor quality data can lead to false positives or negatives, which can undermine the trust in fraud detection systems. Ensuring data integrity is paramount, which often necessitates investing in robust data management solutions and practices that prioritize accuracy and reliability across the board.
Integration of Systems
System integration presents yet another significant hurdle in the fight against fraud. Many businesses operate through multiple platforms with varied data sources. The lack of integration can create blind spots where fraudulent activities may go unnoticed. Seamless integration of systems helps organizations share data efficiently and increases the overall robustness of fraud detection efforts. However, achieving this level of integration requires significant resources and strategic planning. Organizations must navigate technical challenges and often face resistance from internal stakeholders, making this a complex undertaking.
"Combating fraud requires not just a response to current issues, but also a proactive approach to anticipate future threats."
"Combating fraud requires not just a response to current issues, but also a proactive approach to anticipate future threats."
Future Trends in Fraud Detection
The landscape of fraud detection is evolving constantly, shaped by advancements in technology and changing patterns of fraudulent behavior. Understanding future trends in this area is crucial for stakeholders, including businesses, law enforcement, and consumers. The relevance of these trends lies in their potential to significantly enhance the effectiveness of fraud detection systems.
Organizations must consider the implications of these trends as they refine their strategies. Failing to adapt may expose them to greater risks. The integration of emerging technologies offers numerous benefits, such as improved accuracy in identifying fraud and faster response times.
In addition, staying updated with these trends facilitates better regulatory compliance. Organizations can proactively align their practices with evolving legal requirements, minimizing potential penalties. Thus, comprehending future trends is not merely an academic exercise; it has real-world applications that can safeguard both individuals and institutions.
Predictive Analytics
Predictive analytics is increasingly shaping the fraud detection landscape. By leveraging historical data, organizations can forecast potential fraudulent activities. Statistical algorithms analyze patterns and trends, allowing systems to predict when and how fraud may occur. This data-driven approach results in a more proactive stance against fraud, rather than a mere reactive one.
Evidence shows that predictive models enhance decision-making efficiency. They provide insights into customer behavior and transaction anomalies. For instance, financial institutions deploy predictive analytics to identify unusual spending patterns, enabling them to act swiftly and mitigate risks.
- Key Benefits of Predictive Analytics in Fraud Detection:
- Early Detection: Identifying potential fraud before it occurs can save substantial resources.
- Tailored Responses: Organizations can create targeted strategies to combat specific types of fraud.
- Reduced False Positives: Predictive analytics improves the accuracy of fraud alerts, allowing personnel to focus on genuine threats.
Incorporating predictive analytics not only strengthens the organization’s fraud defense but also improves customer trust. Clients appreciate financial entities that utilize advanced methodologies for protecting their interests.
Collaborative Fraud Detection
Collaborative fraud detection marks a progressive approach towards mitigating fraudulent activities. This method involves the sharing of data and insights among different organizations or sectors. Collaboration can take many forms, including partnerships between financial institutions, governmental agencies, and private enterprises.
Through shared data, organizations can build a more expansive picture of fraudulent trends, facilitating quicker response times and collective intelligence to identify fraudsters more effectively. The pooling of resources can lead to:
- Enhanced Data Quality: Combining multiple data sources can produce a richer dataset for analysis.
- Broader Perspectives: Different organizations bring unique expertise, offering diverse strategies for tackling fraud.
- Increased Public Safety: Collaborative approaches can lead to improved overall security in broader financial and transactional systems.
Adopting collaborative fraud detection requires careful considerations of data privacy and compliance issues. Organizations must navigate legal frameworks while fostering relationships for effective information exchange. However, the potential for a collective impact on reducing fraud is significant.
"Fraud detection is not just about technology; it’s about partnerships and shared responsibility."
"Fraud detection is not just about technology; it’s about partnerships and shared responsibility."