RNA Sequencing Normalization Techniques and Effects
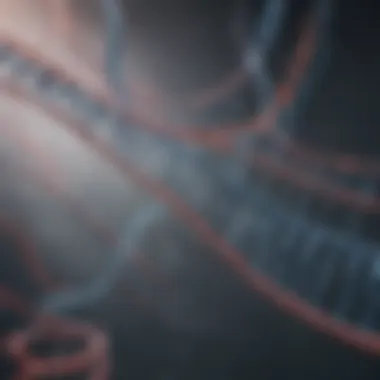
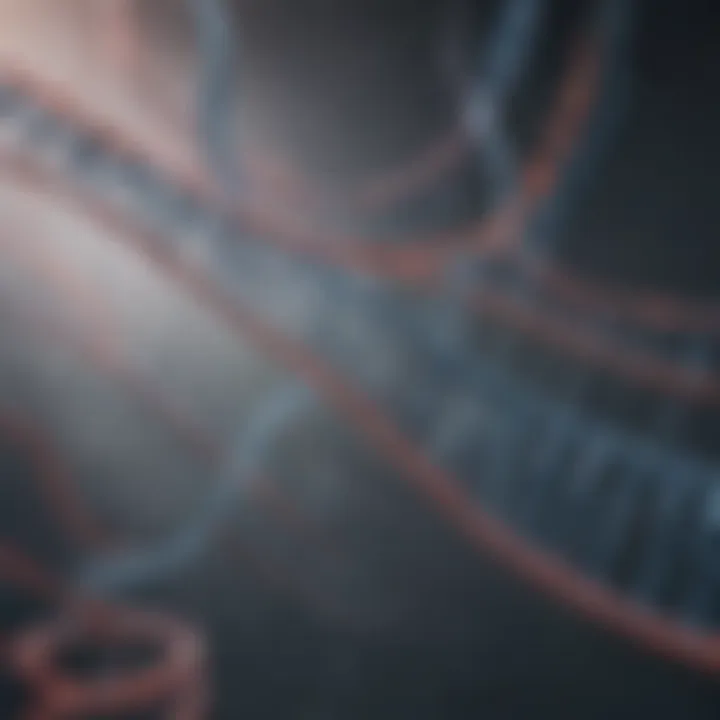
Intro
In the ever-advancing field of genomic research, RNA sequencing serves as a foundational pillar for understanding gene expression and its intricacies. When researchers engage in RNA sequencing studies, the results can be prone to discrepancies resulting from various technical and biological factors. Thus, the normalization of RNA sequencing data emerges as a pivotal step.
Normalization is the process of adjusting data to enable meaningful comparisons across different samples. A well-implemented normalization strategy can dramatically influence the interpretation of gene expression levels, leading to sound conclusions within biological contexts. Whether you are a novice diving into the world of RNA-seq or a seasoned researcher, grasping the fundamentals of normalization techniques is essential.
This article delves into the various methodologies employed in RNA sequencing normalization, highlighting their principles, potential limitations, and implications that resonate through the broader scope of biological research. By weaving a narrative that marries theory with practical applications, we aim to provide clarity around this often intricate topic, equipping you with the knowledge to navigate the complexities of RNA sequencing analysis.
Prolusion to RNA Sequencing
In the realm of molecular biology, RNA sequencing—or RNA-seq—has become an indispensable tool for comprehensively examining gene expression. It provides a window into the intricate workings of cellular machinery, revealing insights about which genes are turned on and off under various conditions. This multifaceted approach holds significance not only for basic research but also for clinical applications, where understanding gene expression can guide therapeutic strategies.
The emergence of next-generation sequencing technologies has drastically enhanced the capacity to analyze RNA samples. These advancements allow for deeper dives into transcriptomics, shedding light on the complexity of gene regulation and alternative splicing events. As researchers venture deeper into the world of RNA-seq, it’s crucial to appreciate normalization—an aspect that can make or break the validity of expression data. Without proper normalization methodologies, interpreting results can be like trying to navigate a maze without a map.
Overview of RNA Sequencing Technologies
RNA sequencing technologies have evolved tremendously over the past decade. Initially, traditional methods such as microarrays were used to assess gene expression, but they faced limitations like low sensitivity and the inability to detect novel transcripts. The advent of high-throughput RNA-seq changed that landscape altogether.
What are the core technologies in RNA sequencing? Here’s a brief rundown:
- Illumina Sequencing: This method, popular for its accuracy and scalability, involves fragmenting the RNA and attaching adapters, allowing for efficient amplification and sequencing of multiple fragments simultaneously.
- Ion Torrent: Using semiconductor technology, Ion Torrent measures changes in pH that occur when nucleotides are incorporated into the DNA strand, offering a rapid and cost-effective sequencing solution.
- Pacific Biosciences: Renowned for its long-read capabilities, this technology presents advantages in resolving complex genomic structures and detecting isoforms that short-read technologies might miss.
In a nutshell, these technologies have democratized high-throughput sequencing, allowing labs of all sizes to generate vast amounts of genetic data. However, this increase in data volume comes with the caveat of necessitating robust normalization techniques to ensure reliable analysis of the results.
Significance of Normalization in RNA-seq
Normalization is akin to leveling the playing field in RNA-seq experiments. Without it, the results can be skewed by various biases—be it technical variations or differences in sample composition. Think of normalization as the equalizer that allows for the proper comparison of samples, particularly when renal gene expression levels might fluctuate due to biological variance or lab handling.
The process helps to combat several influencing factors:
- Library Size Variability: Different samples might be sequenced with varying depths, affecting the overall read counts. Normalization accounts for these discrepancies.
- Batch Effects: When samples are processed in different batches, variations can arise that are unrelated to biological differences. Normalization can help mitigate these effects.
- Gene Length Disparity: Genes with longer sequences naturally yield more reads. Proper normalization techniques can adjust for these variations in nucleotide counts, ensuring that expression levels are comparable.
“Normalization is not merely a procedural step; it’s foundational for meaningful biological interpretation.”
“Normalization is not merely a procedural step; it’s foundational for meaningful biological interpretation.”
Normalization Methods in RNA Sequencing
Normalization methods in RNA sequencing are a fundamental aspect of bioinformatics that ensure the reliability and comparability of gene expression data. When dealing with RNA-seq, the data can be influenced by many external factors. These factors can lead to discrepancies that obscure the true biological signals the researchers are trying to discern. Normalization corrects these biases, enabling more accurate interpretations of gene expression. By understanding these methodologies, researchers can enhance the integrity of their experimental outcomes.
Intro to Normalization
Normalization refers to the process of adjusting values measured on different scales to a common scale. In RNA sequencing, it's essential because the raw read counts generated often reflect not only the biological conditions being studied but also technical variations. For instance, differences in library preparation or sequencing depth can skew the data. Therefore, normalization techniques help mitigate these influences and provide clearer views into the underlying biological processes. By stabilizing the data, researchers can better compare gene expression levels across various conditions and experiment setups.
Quantile Normalization
Quantile normalization is a highly regarded method used to make the distribution of gene expression levels the same across samples. The idea is that, during analysis, every sample should have a similar distribution of expression values, allowing for variance stemming from biological differences rather than technical artifacts. With this method, rank-based values replace raw data, which can lead to powerful adjustments. However, while quantile normalization imposes uniform distribution, it may not account for biological variability adequately, potentially leading to the loss of biologically relevant expression differences.
TMM (Trimmed Mean of M-values)
The Trimmed Mean of M-values, or TMM, is a normalization technique designed to address compositional biases when comparing RNA-seq samples. This method focuses on calculating normalization factors by trimming away the most extreme values and leveraging only those that matter. Essentially, it evaluates ratios of counts, adjusting for differences in library sizes while minimizing the influence of outliers. Notably, TMM is particularly well-suited for studies involving datasets with large variations in gene expression levels across samples. Adaptable and robust, TMM stands as a favored choice within the RNA-seq community.
RPKM and TPM Normalization
RPKM and TPM are two widely employed normalization approaches that focus on gene expression levels. RPKM, or Reads Per Kilobase of transcript per Million mapped reads, adjusts for both gene length and sequencing depth, providing a standardized way to represent expression levels. However, it can be limited in situations where there are low count conditions. TPM, or Transcripts Per Million, also adjusts for gene length but does so in a way that every sample sums up to a total of one million reads. This is pivotal because it overcomes some limitations of RPKM, especially for comparisons between different genes. Both these methods have merits, yet the choice between them depends on specific research contexts.
Size Factor Normalization
Size factor normalization is utilized to adjust for differences in sequencing depth across samples. This method calculates a size factor for each sample based on the geometric mean of counts from all genes. The goal showcases how well new reads account for differences in library sizes. It’s a common approach in workflows utilizing software like DESeq2, which provides statistical power while controlling for varying library sizes. Size factor normalization is crucial, especially in studies comparing multiple samples, as it allows straightforward comparisons without being compromised by technical variations.
Normalization is not merely about adjusting numbers; it's about holding the integrity of biological variances while offsetting technical noise.
Normalization is not merely about adjusting numbers; it's about holding the integrity of biological variances while offsetting technical noise.
By understanding and correctly applying these normalization methods, researchers can significantly enhance the quality of data obtained from RNA sequencing experiments. Each technique has its strengths, and the choice of method should align with the specific goals and characteristics of the experimental design.
Statistical Considerations in Normalization
In the realm of RNA sequencing, normalization sits at the heart of accurate gene expression analysis. The significance of statistical considerations can’t be overstated; they ensure that data is not only interpretable but also reliable when drawing biological conclusions. By addressing fundamental aspects like distributions of expression levels, bias, and variability, researchers can avoid pitfalls that might skew their results. This section will explore these critical statistical elements and their implications for RNA-seq normalization.
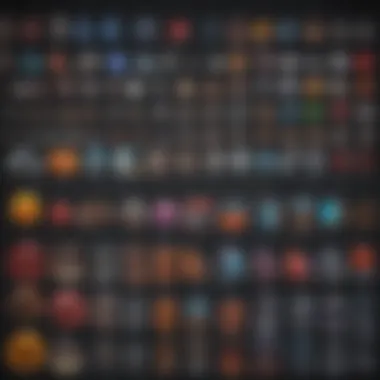
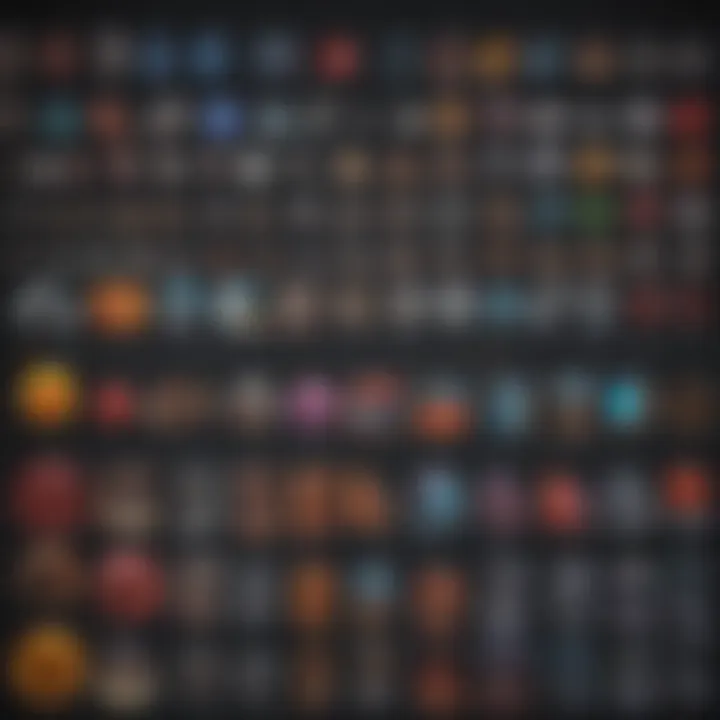
Distributions and Their Impact on Normalization
The essence of effective normalization lies in understanding the distributions of RNA-seq data. Generally, gene expression data do not follow a simple distribution pattern. Rather, they can exhibit complexities such as skewness or multimodality.
- Skewed Distributions: These arise commonly due to lowly expressed genes. If one doesn't account for such skewing, normalization processes might lead to misleading interpretations about gene activity.
- Multimodal Distributions: These may indicate populations of cells or states within the sample, which is vital to recognize, especially in studies involving heterogeneous cell populations.
Failing to accurately assess the underlying distribution can lead the normalization process astray. For instance, if the data is right-skewed, using a method that assumes a Gaussian or normal distribution can distort the results.
It’s essential, therefore, to closely examine the data’s distribution characteristics prior to selecting a normalization method. Visual tools like histograms or density plots can lay bare the nuances in the data distribution, guiding researchers in making informed choices.
Important Note: "A normalization strategy that fails to consider the distribution of the data will yield results as useful as fitting a square peg in a round hole."
Important Note: "A normalization strategy that fails to consider the distribution of the data will yield results as useful as fitting a square peg in a round hole."
Understanding Bias and Variability
In a perfect world, RNA sequencing would yield precise and unbiased data. However, reality presents challenges in the form of inherent bias and variability. Understanding these factors is crucial, as they can distort the interpretative accuracy of gene expression levels.
- Bias can manifest from various sources, including systematical errors that creep into the data collection process or even the biological variability inherent in different samples. Here are some typical examples of bias:
- Variability is another crucial factor to understand. It's the natural fluctuations that occur between samples and within replicates. Not accounting for variability could lead to misinterpretations. A high degree of variability might suggest that a gene does not express significantly between conditions, when in fact the data just reflects sampling noise.
- Library preparation: Different library prep kits might yield varying results, introducing bias.
- Batch effects: Samples processed at different times could show discrepancies due to variations in reagents or environmental conditions.
To address these statistical challenges, tools and methodologies have been developed that help researchers account for bias and capture the true variability within their datasets. Normalization methods must be rigorously evaluated not just for their ability to adjust data but also for how well they address the underlying statistical assumptions they make.
In this landscape, the challenge for researchers is twofold: identify biases and choose the right normalization method that grapples with both bias and variability to produce reproducible results.
Comparative Analysis of Normalization Techniques
In the realm of RNA sequencing, normalization techniques play a pivotal role in refining the data quality and ensuring the reliability of results. Understanding how each methodology stacks up against the others is crucial for researchers aiming to make informed decisions in their analytical workflows. This segment dives into what makes comparative analysis of these techniques not only relevant but essential in advancing RNA-seq studies.
The heart of this comparison lies in several key elements:
- Performance: Different techniques yield varying levels of accuracy, which can significantly influence biological interpretations.
- Applicability: Some methods may suit certain data types better than others, affecting their broader usefulness.
- Complexity and Ease of Use: While some normalization methods can be straightforward, others might involve intricate calculations and significant computational resources.
"Choosing the right normalization technique is akin to laying the groundwork for a sturdy building; if the base is weak, everything else may crumble."
"Choosing the right normalization technique is akin to laying the groundwork for a sturdy building; if the base is weak, everything else may crumble."
Strengths and Weaknesses
Analysis of various normalization approaches reveals a spectrum of strengths and weaknesses.
- Quantile Normalization:
- TMM (Trimmed Mean of M-values):
- RPKM and TPM Normalization:
- Size Factor Normalization:
- Strengths: It standardizes distributions across samples, making data comparison across conditions much easier. By ensuring that each sample follows the same statistical distribution, researchers can make more reliable inferences.
- Weaknesses: However, it assumes that the overall distribution should be uniform, which might not always hold true in biological systems. This oversimplification can mask biological variations.
- Strengths: Highly effective in dealing with RNA-seq data with varying library sizes. It adjusts for compositional differences, which often skew results.
- Weaknesses: The method can be computationally intensive and may not be suited for small sample sizes where variance estimation becomes tricky.
- Strengths: They take into account both gene length and sequencing depth, making them useful for comparing expression levels of genes within the same sample. This adaptability is vital in large-scale studies.
- Weaknesses: RPKM, for instance, does not handle multi-mapping reads well, which can lead to misrepresentations in quantification.
- Strengths: This method excels in addressing library size differences across samples – thus ensuring comparability in expression data.
- Weaknesses: It assumes that all genes are equally expressed, which can skew results for low-variance genes.
Real-World Applications and Results
Normalization techniques have countless applications, impacting research outcomes significantly. Examples that bring these methods to life include:
- Cancer Genomics: Studies involving large patient cohorts have effectively utilized TMM to balance compositional biases, leading to more accurate differential expression analysis in identifying tumor markers.
- Single-cell RNA-seq: In this highly nuanced area, quantile normalization has been pivotal. By ensuring single-cell samples from heterogeneous populations are comparable, researchers can uncover unique cellular functions and interactions.
- Environmental Studies: RPKM and TPM approaches have been applied to evaluate how different environmental stressors—like pollution—affect gene expression in various aquatic species, yielding insights into ecological health.
- Neurobiology Research: Here, size factor normalization is often used in studies of brain tissues for understanding genetic changes associated with neurological disorders. Researchers manage to draw meaningful conclusions from seemingly chaotic data.
The ability to draw meaningful comparisons across various experimental settings has immense implications for advancing science and refining knowledge. By weighing the pros and cons of each method and examining their applied outcomes, researchers can better tailor their approaches, ultimately enhancing the precision and reliability of RNA-seq studies.
Impact of Normalization on Downstream Analyses
Normalization in RNA sequencing is not just a checkbox to tick; it’s a fundamental step that lays the groundwork for reliable interpretations of biological data. Imagine trying to compare apples to oranges—without effective normalization methods, comparing gene expressions across different conditions or samples might lead to skewed or entirely misleading conclusions. It’s like reading a book with some pages missing; you won't get the complete story.
Differential Expression Analysis
Differential expression analysis serves as a cornerstone in RNA-seq research, where the objective is often to identify genes whose expression levels vary significantly between different conditions. Here, normalization plays a pivotal role. For example, if one sample has an exceptionally high number of reads due to technical reasons—like varying sequencing depths—this could create a false indication of differential expression.
When proper normalization techniques are employed, such as TMM or RPKM, the results of differential expression analysis become far more trustworthy. A corrected dataset allows researchers to detect biological insights rather than artefactual discrepancies.
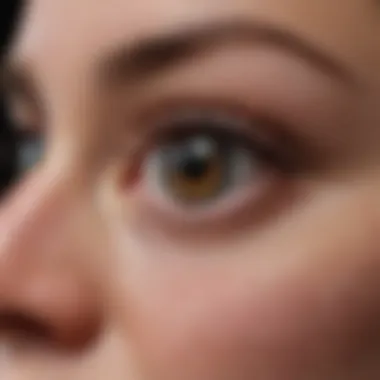
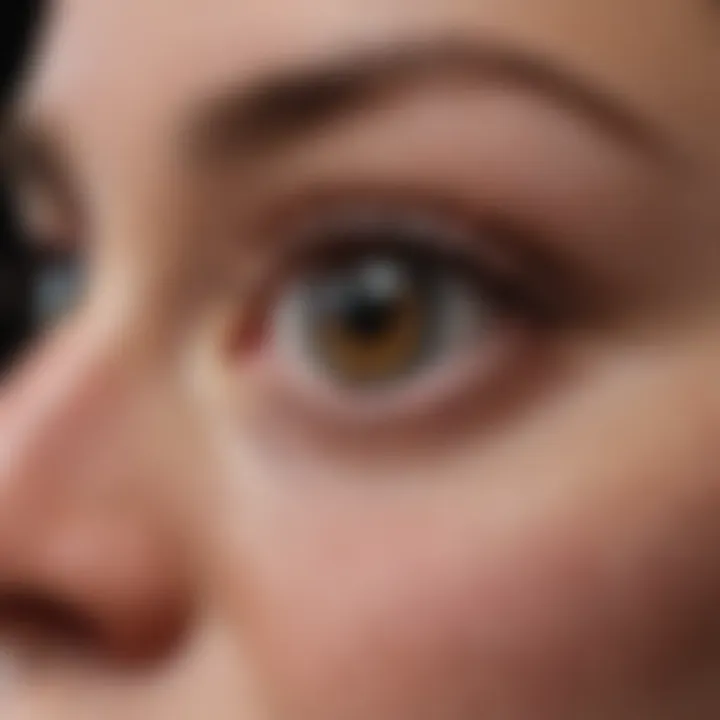
To quantify how normalization affects differential expression results, several studies have shown that non-normalized data can produce up to 50% false positives. This not only misrepresents the biological reality but also wastes valuable resources on follow-up experiments aimed at incorrect findings.
Clustering and Visualization Techniques
In addition to differential expression, normalization is crucial for clustering and visualization of RNA-seq data. When clustering methods, like hierarchical clustering or k-means, are applied without normalization, they can group samples based on underlying biases rather than genuine biological relationships. Poorly normalized data may lead to misleading clusters, suggesting similarities that don't exist.
A properly normalized dataset enhances the clarity of visualizations such as heatmaps or PCA (Principal Component Analysis) plots. These visual tools can reveal patterns and relationships among samples, provided that the underlying data is accurately represented. Without normalization, one might find themselves interpreting random noise as significant trends, akin to trying to make sense of a scrambled puzzle.
Interestingly, using appropriately normalized datasets in visualization not only makes patterns clearer; it also aids in better communication of results to a broader audience, enhancing reproducibility and transparency in research findings.
"Effective normalization is the backbone of reliable RNA-seq downstream analyses, directly influencing the robustness of biological conclusions drawn from the data."
"Effective normalization is the backbone of reliable RNA-seq downstream analyses, directly influencing the robustness of biological conclusions drawn from the data."
In summary, the impact of normalization on downstream analyses cannot be overstated. It enhances the reliability of differential expression analysis and improves the effectiveness of clustering and visualization techniques—critical tools for elucidating the biological phenomena underlying gene expression. Researchers must pay close attention to normalization methods if they hope to avoid pitfalls in interpretation and draw meaningful conclusions from their RNA sequencing data.
Advancements in RNA-seq Normalization Techniques
As we delve into the realm of RNA sequencing, it becomes apparent that methodologies are evolving at a rapid pace. This section sheds light on crucial advancements in RNA-seq normalization techniques, underscoring their significance for ensuring the accuracy and reliability of gene expression analyses. With the constant barrage of data pouring in from various experimental setups, the need for effective normalization methods has never been as pressing.
One of the standout features of modern RNA-seq normalization is its adaptability to different types of data and experimental conditions. Instead of a one-size-fits-all approach, advancements now allow for tailored normalization strategies that take into account sample-specific characteristics. This is vital, as conventional methods can sometimes strip away biologically relevant variances, resulting in a distorted view of gene expression profiles.
Emerging Technologies and Their Role
Emerging technologies play a pivotal role in shaping the landscape of RNA-seq normalization. One notable advancement is the advent of single-cell RNA sequencing, which has opened new avenues for understanding cell-to-cell variability. In this context, normalization techniques such as scran and SCnorm have emerged to handle the intricacies of single-cell data—where traditional bulk normalization methods fall short.
Moreover, new sequencing platforms are continually being developed, each generating unique types of data. These innovations demand specialized normalization approaches that can adequately account for differences in sequencing depth and the complexity of transcripts.
Here are a few technologies making waves in RNA-seq normalization:
- Single-cell RNA-seq platforms: Yield insights into cellular heterogeneity.
- Long-read sequencing technologies: Improve the detection of transcript variants.
- Massively parallel RNA sequencing: Boost throughput, highlighting the need for sophisticated normalization.
As these technologies proliferate, researchers find themselves at a crossroads, needing to pick the right normalization strategy for their specific context to ensure robust conclusions.
Integration of Machine Learning and AI
The past few years have witnessed an emerging synergy between RNA-seq normalization and machine learning. Researchers are increasingly leveraging AI algorithms to enhance normalization processes, which can lead to improved data quality and more reliable analyses. This integration involves using machine learning models to predict optimal normalization parameters that account for various biases inherent in gene expression data.
A few key points on how AI and machine learning are transforming RNA-seq normalization include:
- Adaptive normalization: Models that adjust normalization processes in real-time as new data is accrued.
- Bias detection: Algorithms can identify and correct for biases that might go unnoticed in traditional methods.
- Predictive modeling: Utilizing training datasets to predict optimal thresholds for reads, allowing for more nuanced analysis.
"The future of RNA-seq normalization hinges on not just understanding the science, but also exploiting the potential of technological advances."
"The future of RNA-seq normalization hinges on not just understanding the science, but also exploiting the potential of technological advances."
Practical Considerations for Researchers
Understanding the landscape of RNA sequencing normalization is pivotal for ensuring high-quality outcomes in gene expression studies. As researchers delve into this complex field, there are several practical considerations that can significantly shape their results. Normalization is not merely a procedural step; it plays a crucial role in the integrity of the data generated from RNA-seq experiments.
First and foremost, awareness of the different normalization methodologies is essential. Each technique comes with its specific strengths and weaknesses, which can influence the interpretation of the data. For instance, methods that work well in one type of dataset may not be as effective in another. Recognizing these nuances allows researchers to tailor their approaches, creating a more robust framework for analysis.
Moreover, practical concerns about sample types and their respective quality shouldn't be overlooked. The biological variability present in RNA samples can introduce additional layers of complexity. For example, factors like tissue heterogeneity or variations in RNA extraction methods can skew results. Thus, choosing a normalization strategy that accounts for these variables is fundamental.
In addition to methodological and biological considerations, it’s also important to think about computational resources. Normalization methods can demand varied computational power and time. Researchers must assess their available tools and potentially invest in robust software capable of handling their specific datasets. This helps to streamline the process while reducing the likelihood of errors or discrepancies in data analysis.
Lastly, collaboration is a cornerstone of good research practices. Engaging with bioinformaticians or statisticians can provide invaluable insights into choosing the best normalization methods suited for particular research questions. Through sharing expertise, researchers can elevate the standard of their findings, leading to more impactful contributions to the field.
"The right normalization strategies can turn a mountain of raw RNA-seq data into a summit of insights and discoveries."
"The right normalization strategies can turn a mountain of raw RNA-seq data into a summit of insights and discoveries."
By being aware of these practical considerations, researchers can make informed decisions that not only enhance their analyses but also contribute to the broader scientific community.
Best Practices in Normalization
Implementing best practices in RNA sequencing normalization is crucial for producing accurate and reliable gene expression profiles. Here are several key principles to keep in mind:
- Plan Early: Integrate normalization into the design phase of your experiment. This foresight can guide your sample collection approaches and how you handle data.
- Understand Your Data: Familiarize yourself with the types of data you are working with. Are your samples pooled or individual? Do they come from controlled experiments or heterogeneous backgrounds? Understanding these factors can greatly influence the choice of normalization method.
- Multiple Methods: Don't hesitate to apply different normalization techniques to your data set. This can help elucidate inconsistencies or reveal hidden patterns that might otherwise go unnoticed.
- Document Everything: Keep a thorough record of the methods used, values obtained, and decisions made throughout the process. Clear documentation is vital for reproducibility and validation by others in the field.
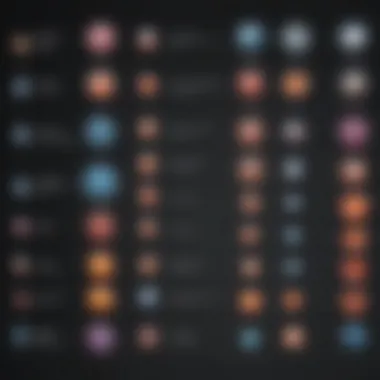
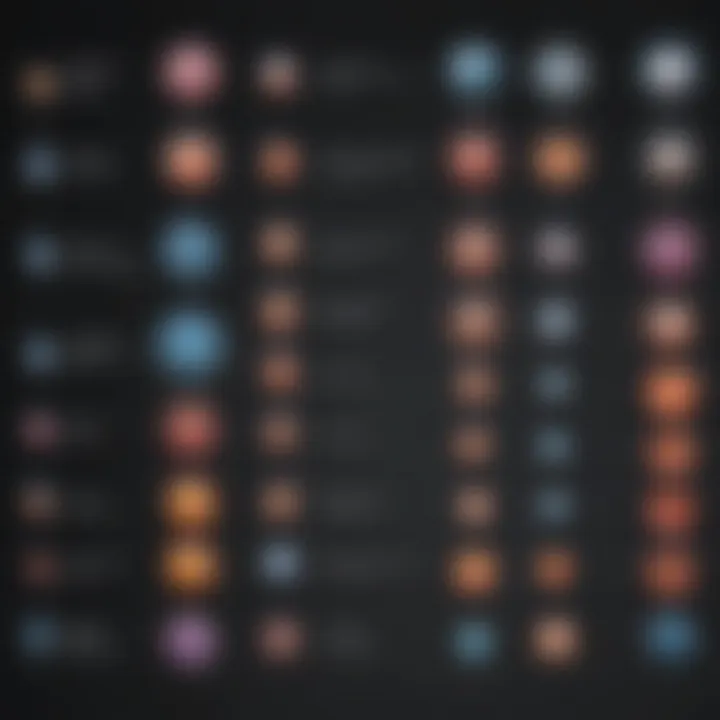
Following these guidelines not only solidifies the foundations of your analysis but also enhances the reliability of your conclusions.
Choosing the Right Normalization Method
Selecting the appropriate normalization method for RNA sequencing can often be a daunting challenge due to the variety of techniques available. Here are some factors to consider when making your choice:
- Nature of the Data: Take into account whether your data is count-based or log-transformed. For instance, techniques like TMM are well-suited for count data while methods like RPKM could be more appropriate when dealing with log-transformed datasets.
- Sample Size: The scale of your experiment can determine the normalization method's efficiency. Larger samples might allow for methods that require more complex calculations, while smaller datasets may benefit from simpler approaches.
- Variability Sources: Identify the sources of variability affecting your results. If biological variability is significant, methods that correct for this may be necessary. Conversely, technical variance could require different strategies altogether.
- Software Compatibility: Ensure that the normalization method you choose is compatible with the tools and software packages you are using. This can facilitate smoother data processing and analysis.
Case Studies of RNA-seq Normalization
Understanding RNA sequencing normalization becomes clearer when discussing specific case studies that demonstrate its application in real-world scenarios. Case studies are not just useful anecdotes; they provide concrete evidence and insight into the effectiveness or pitfalls of different normalization methods. As researchers dive into their data, these examples can offer practical guidance while also highlighting the challenges faced in various contexts. Moreover, case studies showcase the evolution of methodologies and present potential strategies for future research.
Notable Research Examples
Several research initiatives have utilized RNA sequencing normalization to uncover significant biological insights. Here are a few noteworthy examples that solidify the importance of careful normalization:
- Cancer Genomics Studies: A research team focusing on cancer genomics applied TMM normalization in their analyses to identify differentially expressed genes across various cancer types. This approach helped clarify the unique gene expression signatures associated with each type, facilitating more tailored therapeutic strategies.
- Developmental Biology: In a study assessing gene expression during embryonic development, researchers used quantile normalization to handle technical variations arising from sample collection. This precision allowed them to identify crucial gene regulatory networks involved in early developmental processes, indicating how normalization can aid in understanding complex biological systems.
- Metagenomics: A research project centered on metagenomic analysis employed size factor normalization to account for sequencing depth variations across microbial communities. This normalization method revealed vital ecological interactions that would have remained obscured without appropriate normalization, illustrating the relevance of methodologies across different fields.
These examples emphasize the diverse applications of RNA-seq normalization and how the choice of technique can significantly influence research outcomes.
Lessons Learned from Case Studies
The insights gained from these case studies reveal several key lessons for researchers:
- Normalization Matters: One significant takeaway from all these examples is that neglecting normalization can lead to misleading results. Accurate normalization methods are crucial for reliable data interpretation, underscoring the necessity of incorporating these techniques from the start.
- Tailored Approaches: No one-size-fits-all solution exists when it comes to RNA-seq normalization methods. The studies indicate that researchers need to tailor their normalization strategies to the specific nuances of their data and research goals. For instance, what works in a metagenomic study may not be suitable for cancer research.
- Incremental Improvements: Each case illustrates that continual refinement of normalization methods can lead to improved accuracy and reproducibility in results. Researchers need to stay abreast of advancements and consider how emerging techniques may enhance their work.
- Collaborative Learning: Engaging with the broader research community through case studies fosters an environment of knowledge sharing. Learning from others' experiences helps avoid common pitfalls and can inspire innovative approaches to RNA-seq normalization.
By examining case studies, researchers not only gain practical knowledge but can also foresee potential challenges and strategize their research accordingly.
By examining case studies, researchers not only gain practical knowledge but can also foresee potential challenges and strategize their research accordingly.
In sum, implementing lessons drawn from well-documented examples provides an invaluable resource for new and experienced researchers alike. Each study showcases the substantial role that appropriate normalization plays in ensuring the integrity and applicability of RNA sequencing data in addressing fundamental biological questions.
Future Directions in RNA Sequencing Normalization
As RNA sequencing technology continues to evolve, so too do the methodologies surrounding normalization. This section examines what lies ahead in RNA sequencing normalization, focusing on emerging innovations, cross-disciplinary collaboration, and how they shape the future landscape of gene expression analysis.
Innovations on the Horizon
The field of RNA sequencing normalization is experiencing rapid advancements that promise to enhance data reliability and interpretability. As researchers grapple with the complexities of biological systems, the importance of precision cannot be overstated. One core innovation is the development of dynamic normalization methods that adapt based on the characteristics of specific datasets. These techniques, unlike static models, can adjust in real-time to variations in data distribution and sample attributes.
Additionally, there's a growing trend towards utilizing advanced computational algorithms to manage and analyze normalization techniques. Machine learning, for instance, is becoming increasingly integrated into normalization workflows, helping to identify hidden patterns and biases in large genetic datasets. Leveraging techniques like these leads to a more refined understanding of biological processes, paving the way for more nuanced interpretations of gene expression.
Beyond computation, the push towards open-source software and collaborative platforms is gaining traction. Researchers can share their tools and methodologies, fostering a more diverse approach to normalization strategies. This culture of innovation not only enriches the field but also facilitates a sense of community among scientists.
"Innovation in RNA sequencing normalization is not just about better numbers; it's about better science."
"Innovation in RNA sequencing normalization is not just about better numbers; it's about better science."
Collaboration Across Disciplines
To fully harness the potential of RNA sequencing normalization, collaboration across various disciplines is crucial. Biologists, bioinformaticians, statisticians, and even those from engineering backgrounds must come together to bridge the gap between experimental design and data analysis. Each discipline brings unique strengths that can enhance the quality of normalization techniques.
For instance, biologists can provide insights into the biological relevance of different normalization strategies, while bioinformaticians can offer expertise in developing algorithms that can address specific challenges. This sort of interdisciplinary cooperation not only leads to the refinement of existing methods but also facilitates the exploration of novel strategies that can be tailored to specific research questions.
At the same time, cross-disciplinary training programs play a vital role in preparing a new generation of researchers adept at navigating the complexities of RNA sequencing normalization. Such initiatives can foster a robust understanding of both the biological fundamentals and the statistical principles that underpin RNA-seq analysis.
In summary, the future directions in RNA sequencing normalization are marked by innovations that extend beyond traditional statistical methods, paving the way for richer biological insights. By embracing interdisciplinary collaboration, the field stands poised to tackle emerging challenges in RNA sequencing analysis, ultimately benefiting the broader scientific community.
Culmination
The conclusion serves as a critical capstone for the entire discourse on RNA sequencing normalization. It distills the essence of the article and brings into focus the various points discussed earlier. The goal is not just to recap but to provide a sense of closure that aligns readers with the implications of what they've learned.
Summarizing Key Findings
In essence, RNA sequencing normalization is a multifaceted aspect of data analysis that cannot be taken lightly. Some key findings warrant reiteration:
- Normalization Techniques: Diverse methods such as Quantile Normalization, TMM, RPKM, and even size factor normalization each have unique strengths and weaknesses. Choosing the right one arises not only from understanding how they function but also from considering the specific context in which they are applied.
- Impact on Data Integrity: The nitty-gritty details of normalization can drastically alter the outcomes of differential expression analyses and downstream applications. It's crucial to prioritize robust methodologies that lessen bias.
- Real-World Applications: Case studies highlighted the practical implications of normalization in various biological contexts, signifying that what works in theory could shift dramatically based on experimental conditions.
This synthesis informs researchers about the indispensable role of normalization in RNA-seq studies, affecting their trajectory of future work in profound ways.
Significance for Future Research
As research endeavors evolve, so does the methodology surrounding RNA sequencing normalization. Future work is likely to benefit from emerging technologies and interdisciplinary approaches. Here are some considerations for further exploration:
- Refinement of Techniques: Ongoing refinement in normalization methodologies will likely focus on enhancing accuracy while reducing variability in diverse data sets. Researchers should keep an eye on newer algorithms that may address current limitations.
- Integration with Machine Learning: The growing intersection of machine learning and RNA-seq normalization introduces new dimensions for analysis. As computational techniques continue to mature, the potential for better predictive modeling and data interpretation expands significantly.
- Broader Collaborations: Collaboration across disciplines will enrich the understanding of normalization’s implications. Biostatisticians, computational biologists, and experimentalists must engage in dialogue to push the boundaries of existing knowledge.
In summation, while there’s much to digest from the nuances of normalization, the benefits of harnessing its full potential are monumental. By focusing on these elements, future research can not only enhance the accuracy of gene expression studies but also foster a deeper understanding of the complex biological landscapes they aim to navigate.