The Role of Data in Modern Agricultural Practices
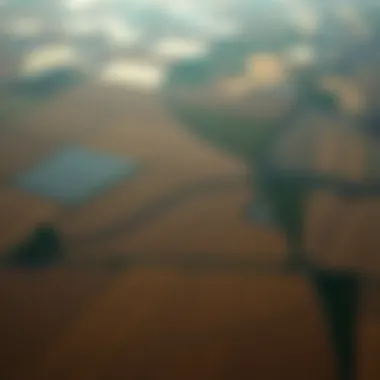
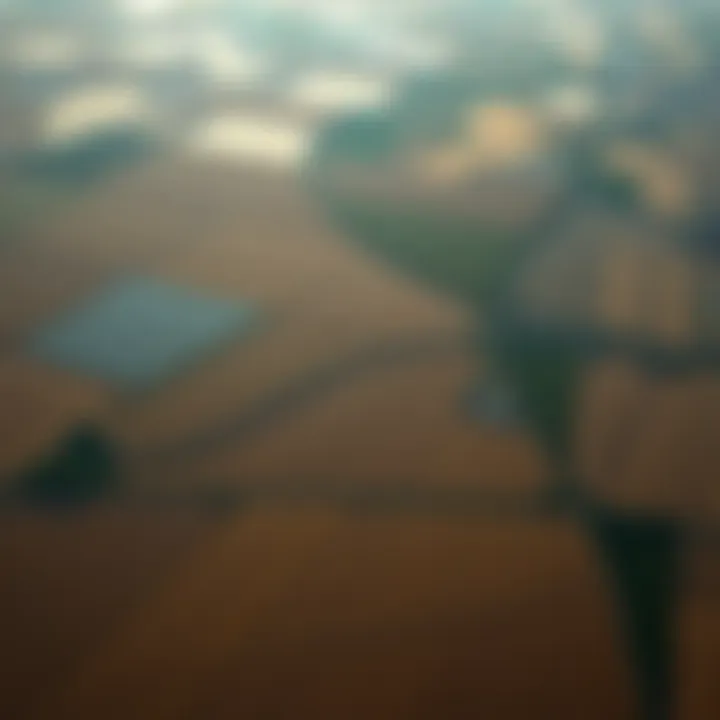
Intro
In an age where data reigns supreme, agriculture is no exception. As farmers navigate a changing landscape, the integration of data into farming practices has become essential. The notion of agriculture data encompasses a vast array of information sources, such as satellite imagery, soil moisture levels, and climatic patterns. These elements serve as the bedrock for informed decision-making, aiming to optimize productivity while fostering sustainability.
The interplay between technology and agriculture is transforming traditional methods into precision farming. This means that farmers harness specific datasets to tailor their approaches, addressing the unique needs of their crops and fields. You don’t have to be a tech whiz to understand the basics; however, recognizing the potential of agriculture data can greatly influence both yield and resource management.
As we delve deeper into this exploration, we will highlight key concepts, current research trends, and the ethical considerations that accompany the rise of big data in farming practices. Our goal is to unravel how data can be leveraged to enhance efficiency and sustainability in the agricultural sector. Let's embark on this journey into the agriculture data landscape, shedding light on its significance and implications for the future of farming.
Key Concepts
Definition of the Main Idea
At its core, agriculture data refers to the quantitative and qualitative information collected from various sources to inform agricultural practices. This can include:
- Satellite imagery: Provides an overhead view of land use and crop health.
- Soil sensors: Measure pH, moisture, and nutrients levels to inform fertilization and irrigation strategies.
- Weather patterns: Impacts decisions on when to plant, irrigate, and harvest crops.
The integration of this data allows for a holistic approach to farming, helping to maximize both yield and resource conservation.
Overview of Scientific Principles
Understanding the practical applications of agricultural data requires familiarity with several scientific principles that underpin its utility:
- Remote Sensing: Utilizes satellite and drone technology to assess land and crop conditions.
- Data Analytics: Transforms raw data into actionable insights through statistical and computational techniques.
- Precision Agriculture: Combines technology with traditional farming methods to optimize inputs and reduce waste.
By leveraging these scientific principles, farmers can make informed choices that align with both immediate production goals and long-term sustainability.
Implementing data-driven strategies in agriculture doesn’t just enhance productivity; it also plays a critical role in combatting climate change and ensuring food security for future generations.
Implementing data-driven strategies in agriculture doesn’t just enhance productivity; it also plays a critical role in combatting climate change and ensuring food security for future generations.
Current Research Trends
Recent Studies and Findings
In recent years, an array of studies has revealed the profound impact that agriculture data can have on farm management. Notable findings include:
- Farmers using satellite imagery improved crop yield predictions by over 20%.
- Analysis of soil sensor data led to a 30% reduction in water usage without compromising yield.
These insights underscore the importance of incorporating data into farming practices as a means to boost productivity and conserve resources.
Significant Breakthroughs in the Field
The landscape of agriculture data is also witnessing significant breakthroughs, such as:
- Artificial Intelligence (AI): AI algorithms allow for more accurate forecasting and anomaly detection in crop health.
- Blockchain Technology: Ensures transparency and traceability in food supply chains.
These advancements not only promise to revolutionize farming practices but also raise new questions about data ownership and ethical use.
As we explore this realm further, it is vital to recognize that alongside the benefits, there are challenges to address. Issues surrounding data privacy and ethical implications of data use remain at the forefront of ongoing discussions in the agricultural sector.
To understand these complexities and their implications for future agricultural practices, we will continue to unravel the intertwined themes of data utility and ethical considerations in subsequent sections.
Understanding Agriculture Data
In the modern landscape of farming, agriculture data has emerged as a critical element influencing various aspects of production. Understanding how this data works is not merely academic; it's foundational for anyone involved in the agricultural sector, from farmers to policymakers. At its core, agriculture data encompasses a range of information that can enhance decision-making, optimize resources, and ultimately increase yield.
When diving into agriculture data, one must consider its vast applications, which can bolster productivity and efficiency. Think of this data as the backbone of precision farming, where each piece of data collected helps in fine-tuning techniques for crop management. Armed with the right data, farmers can make informed choices, such as when to plant, irrigate, or harvest. This ability to analyze and act upon accurate data translates directly into improved outcomes, not just for individual farms but for the entire food supply chain.
Another layer of importance in understanding agriculture data is its role in sustainability. Increased scrutiny on agricultural practices has led to an demand for transparency regarding resource usage. By utilizing data, stakeholders can track water consumption, soil health, and carbon emissions. This shift toward data-driven methods supports sustainable initiatives while addressing ethical concerns regarding land and resource management.
In summary, understanding agriculture data opens up new avenues for enhancing productivity and sustainability. It encourages the adoption of technology in farming practices, ensuring that stakeholders are equipped to meet present and future challenges. Without a doubt, the relevance and benefits of agriculture data are pivotal in fostering a resilient agricultural ecosystem.
Defining Agriculture Data
Agriculture data can be broadly defined as the information pertaining to various farming activities, environmental factors, and market dynamics. Understanding what encompasses this term is essential for harnessing its potential. Essentially, it includes data about crop yields, soil conditions, weather patterns, market prices, and even data harvested from satellite imagery and sensors. This comprehensive view allows for complex analyses and more informed decision-making.
Types of Agriculture Data
Agriculture data can be categorized based on several criteria, which help clarify its various applications. Here, we break it down into several critical types.
Quantitative vs Qualitative Data
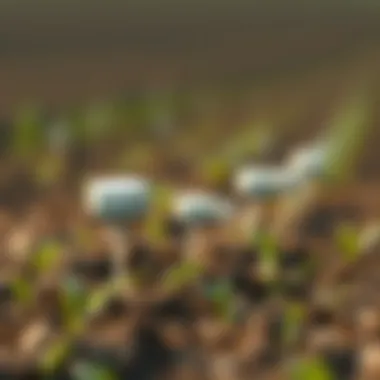
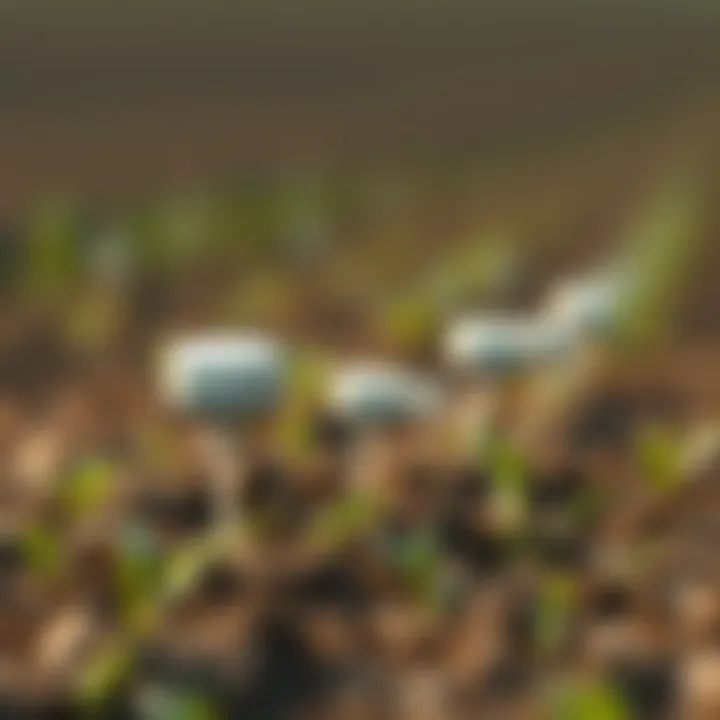
When we talk about quantitative data, we're diving into the realm of numbers and statistics. This type includes measurable factors like crop yield per hectare, rainfall amounts, or market prices. It's very beneficial for providing clear and objective analyses, essential for performance evaluations.
On the flip side, qualitative data brings in the anecdotal evidence or subjective insights from farmers, like crop health observations or the effectiveness of certain farming practices. This data type is particularly useful in understanding trends that numbers alone might miss. For example, a farmer’s experience regarding climate change may not be easily quantifiable but offers deep insights into resilience strategies.
Each type has its strong suits; quantitative data usually allows for broad comparisons and trends, while qualitative data adds richness and context, nurturing an environment where comprehensive strategies can be derived.
Primary vs Secondary Data
Primary data comes from firsthand sources, often collected through surveys, observations, or experiments. This type is particularly powerful because it offers fresh and relevant insights directly tied to current conditions on the ground. For instance, field trial results or soil tests would be categorized as primary.
Conversely, secondary data includes information that has been collected and analyzed by others, such as academic research, government reports, or industry publications. While secondary data can be valuable for trend analysis or historical context, it may lack the specificity needed for localized decisions. Using both types optimally can bring together the best of both worlds, bridging gaps and enhancing understanding.
Real-time vs Historical Data
Real-time data is the information that is collected and processed instantly, allowing for immediate decision-making. Weather updates for crop irrigation or current market prices are prime examples. This immediacy can be a game changer, enabling farmers to react to conditions as they unfold.
Historical data, however, provides valuable context over longer periods. It looks at trends and patterns from past seasons, helping farmers anticipate what may happen based on previous knowledge. For example, analyzing last year’s crop yield in relation to rainfall can be crucial for planning this year’s growth strategies.
To sum up, both types of data serve their purpose well. Real-time input fosters quick responses to changing conditions, while historical data informs long-term strategies and planning. These insights empower stakeholders, enhancing their ability to navigate an increasingly complex agricultural environment.
Sources of Agriculture Data
Understanding the sources of agriculture data is vital as it lays the foundation for informed decision-making in farming practices. Different data types come from diverse origins, each offering unique insights that feed into agricultural analysis and planning. These sources not only help farmers monitor crop performance but also provide critical information for resource management, yield prediction, and market dynamics.
Each source brings its own set of advantages and potential limitations. For instance, data derived from satellite imagery can provide a broad view across vast landscapes, while soil sensors provide granular detail about specific fields. This mosaic of data types offers farmers a comprehensive toolkit to optimize their operations.
Satellite Imagery
Remote Sensing Techniques
Remote sensing techniques utilize satellite imagery to gather data about the Earth's surface without needing direct contact. This technology's key characteristic is its ability to capture detailed images over wide areas, showcasing highly relevant agricultural conditions from above. Its usefulness lies in providing a comprehensive picture of crop health and land use.
One unique feature is the resolution of the images captured, which can vary significantly depending on the satellite. Higher resolution images allow for precise assessments of crop conditions but require more substantial investment in data processing. The benefit of remote sensing techniques is clear, as these capabilities enable farmers to make data-driven decisions quickly; however, a significant drawback could arise from the need for technical expertise to interpret the data effectively.
Uses in Crop Monitoring
The use of satellite imagery in crop monitoring is critical. It helps farmers identify issues such as pest infestations, nutrient deficiencies, and water stress early on. This proactive approach to monitoring enables timely interventions that can greatly enhance yield.
The ability to visualize crop progress over large areas is a significant advantage, as farmers can efficiently allocate their resources where needed most. However, the reliance on satellite data might pose challenges in terms of accessibility and affordability for smaller-scale farmers. Despite these hurdles, the benefits of continuous monitoring often outweigh the disadvantages, leading many to adopt this method.
Soil Sensors
Types of Soil Sensors
Soil sensors are an integral source of agriculture data, measuring parameters like moisture levels, temperature, and nutrient concentration directly in the field. Their distinct advantage lies in the ability to provide real-time readings, which can help farmers make immediate adjustments to irrigation and fertilization practices.
Different types of soil sensors, such as capacitance sensors and TDR (Time Domain Reflectometry) sensors, cater to a variety of needs depending on the soil type and crop being cultivated. The unique capability of these sensors to transmit data wirelessly allows for easy integration into broader farm management systems. However, installation costs and maintenance can become a barrier for some farmers, particularly in regions with less regulatory support.
Data Collection Methods
Data collection methods for soil sensors vary, incorporating manual and automated approaches. Automated systems tend to be more efficient, as they continually gather information without requiring farmer intervention. This is particularly valuable in environments where weather conditions can change rapidly.
A key characteristic of these methods is intensity; a greater coverage area can significantly enhance data usability. Nevertheless, the complexity of setting up these systems can be daunting for those unfamiliar with technology. Despite the initial learning curve, farmers equipped with soil sensor data can fine-tune their farming practices for better crop yields.
Weather Data
Impact on Crop Yields
Weather data significantly affects agricultural productivity. It includes temperature, rainfall, humidity, and wind conditions, all of which play a role in crop development. Understanding how weather impacts crop yields is essential for farmers aiming to maximize their harvest.
For instance, prolonged drought conditions or unexpected frosts can devastate yields, driving home the importance of weather forecasting. By incorporating accurate weather data, farmers can mitigate risks associated with climate fluctuations. However, reliance on weather forecasts raises concerns, as models may not always be precise, leading to potential losses if decisions are based on inaccurate predictions.
Weather Forecasting Models
Weather forecasting models leverage historical data and advanced algorithms to predict future weather conditions. By analyzing patterns, these models help farmers prepare for upcoming challenges. One benefit of using sophisticated forecasting models is their capability to offer localized predictions, which is crucial for farm-level decision-making.
Nevertheless, the technology involved can often be complicated to understand and implement, posing a barrier to some. The volatility of weather conditions also means forecasts can sometimes err, which can influence the trust farmers place in these tools. Despite challenges, reliable forecasting models can enable farmers to be proactive in their planning and minimize unforeseen impacts.
Market and Economic Data
Pricing Trends
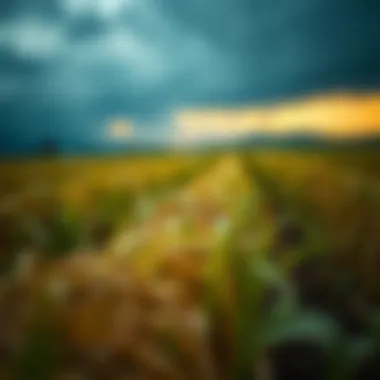
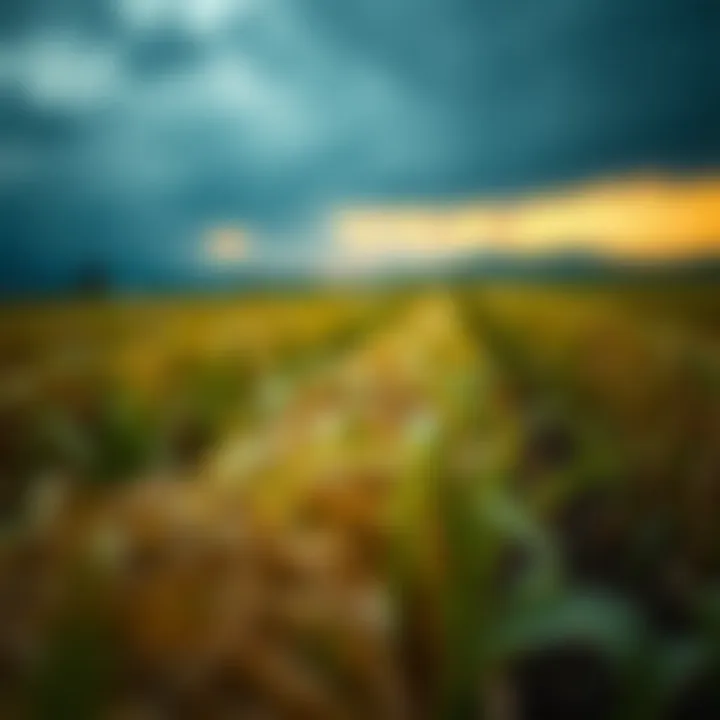
Understanding pricing trends is fundamental for farmers as it allows them to strategize their production and sales efforts. By keeping tabs on commodity prices, farmers can time their harvests and sales to maximize profit potential. This economic data is crucial; fluctuations in market values can have a profound impact on the farming business.
A distinct benefit of monitoring pricing trends is that it equips farmers with the knowledge needed to negotiate better terms with suppliers and buyers. However, price volatility can sometimes lead to unpredictability, making it essential for farmers to stay informed about market dynamics regularly.
Supply Chain Information
Supply chain information provides insights into how agricultural products move from producers to consumers. An understanding of this interconnected system is vital for optimizing processes and ensuring timely deliveries. The key characteristic of using supply chain data hinges on its ability to identify bottlenecks or inefficiencies, which can lead to waste reduction.
Data Analysis in Agriculture
Data analysis plays a crucial role in optimizing agricultural practices. It provides insight into various aspects of farming, helping stakeholders make informed decisions. By examining patterns and trends within agricultural data, farmers can effectively manage resources, predict outcomes, and increase productivity. The implications of this analysis are vast, ranging from improved yield forecasts to better resource allocation, ultimately contributing to sustainability and profitability.
Data Processing Techniques
Data Cleaning
Data cleaning is essential in ensuring the accuracy of agricultural datasets. It involves identifying and correcting errors or inconsistencies within the data. This step is significant because even a small flaw can lead to misguided conclusions, impacting decision-making processes. A key characteristic of data cleaning is its ability to enhance data quality by eliminating noise and irrelevant information, making it a popular choice among data analysts in agriculture. The unique aspect of data cleaning lies in its systematic approach to resolving discrepancies, which can save time and resources in the long run, though it may be somewhat labor-intensive.
Data Integration
Data integration is the process of combining data from various sources to create a cohesive dataset. In agriculture, this can include integrating information from soil sensors, weather stations, and satellite imagery. The main benefit of data integration is that it provides a holistic view of agricultural conditions, which can improve real-time decision-making. The characteristic of data integration that stands out is its ability to merge diverse data types into a unified format, allowing for more comprehensive analyses. However, challenges can arise when trying to synchronize data formats, which can lead to compatibility issues if not handled properly.
Statistical Analysis
Descriptive Statistics
Descriptive statistics encapsulate the basic features of the data being analyzed. This includes measures such as mean, median, and mode, which help summarize key aspects of agricultural trends. The benefit of descriptive statistics is its straightforward nature—it directly conveys information about the dataset. A unique feature of descriptive statistics is its role in providing a clear snapshot, which can help stakeholders quickly grasp essential trends without getting bogged down in complex analysis. However, it does have limitations as it does not account for underlying patterns that might need further investigation.
Inferential Statistics
Inferential statistics allows for conclusions and predictions based on a sample of agricultural data. Its significance lies in its ability to extend findings from a small group to a larger population. One of the key characteristics of inferential statistics is hypothesis testing, which can guide farmers in making decisions based on sample outcomes. This is especially beneficial for making predictions about crop yields or pest infestations. A unique feature of inferential statistics is in its capacity to provide insights that can forecast trends, though it may introduce biases if the sample is not representative, leading to incorrect conclusions.
Machine Learning Applications
Predictive Modelling
Predictive modelling utilizes data mining, statistics, and machine learning to analyze patterns and predict future outcomes in agriculture. Its contribution is significant, enabling predictions of market trends or crop performance based on historical data. The standout characteristic of predictive modelling is its iterative learning process, continuously improving its accuracy over time. This is certainly advantageous for agricultural stakeholders, as it allows for proactive rather than reactive strategies. However, reliance on complex algorithms can make it challenging for some farmers to fully understand or trust the insights provided.
Crop Yield Forecasting
Crop yield forecasting leverages various data inputs to estimate future crop outputs. Its importance is clear, as accurate forecasts allow farmers to plan for harvest seasons and manage resources effectively. The unique aspect of crop yield forecasting lies in its combination of biological, environmental, and economic data, creating a multifaceted approach to yield estimation. This technique is largely beneficial in supporting food security initiatives, yet it carries the risk of inaccuracies due to unpredictable environmental factors, which can influence crop performance beyond the modeled parameters.
In agriculture, knowledge derived from data analysis not only drives efficiency but also supports sustainable practices. By adjusting production based on data-driven insights, farmers can conserve resources and minimize environmental impacts.
In agriculture, knowledge derived from data analysis not only drives efficiency but also supports sustainable practices. By adjusting production based on data-driven insights, farmers can conserve resources and minimize environmental impacts.
Understanding and applying data analysis is vital for modern agriculture, offering a path toward increased productivity and sustainability.
Big Data in Agriculture
The proliferation of big data is transforming the landscape of agriculture. With vast quantities of information available, farmers and agricultural businesses can harness this data to boost efficiency and productivity. Farmers today are not just laborers tending to crops; they are also data-savvy individuals who utilize information to make well-informed decisions. The sheer volume of data at their disposal—from market trends to environmental conditions—allows for a more nuanced approach to farming that is increasingly data-driven.
Impact on Farming Efficiency
Precision Agriculture
Precision agriculture represents a significant leap forward in farming techniques. This method utilizes detailed data, collected from various sources like soil sensors and satellite imagery, to make precise farming decisions. A key characteristic of precision agriculture is its ability to tailor farming practices to specific field conditions. By understanding the variability of soil health or moisture content, farmers can optimize water usage, fertilizers, and other inputs.
- Unique Feature: By integrating GPS technology and remote sensing, farmers can create detailed maps of their fields, allowing for targeted interventions in crop management.
- Advantages: This fine-tuning can result in substantial cost savings and yield improvements, making it a popular choice among modern farmers. However, there are also challenges. The initial investment in technology can be substantial, which might be a barrier for smaller operations.
Resource Management
Resource management in agriculture has grown increasingly sophisticated due to the insights provided by big data. Effective resource management enables farmers to use resources—whether water, nutrients, or equipment—more effectively.
- Key Characteristic: It involves analyzing data to determine the best times to plant, irrigate, or harvest based on specific conditions.
- Unique Feature: The ability to analyze historical weather patterns alongside real-time data can optimize resource allocation, preventing waste.
- Advantages: By harnessing data for decisions, farmers can significantly enhance their sustainability practices. Still, excessive dependence on data without proper interpretation can lead to misguided decisions. Finding the right balance is critical.
Challenges of Big Data
As beneficial as big data may be, it is not without its pitfalls.
Data Overload
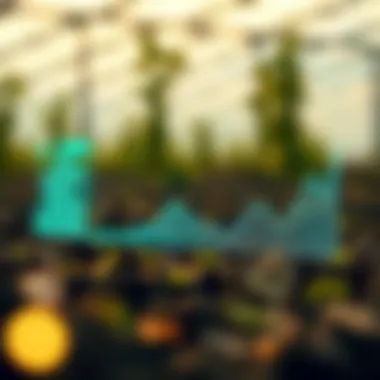
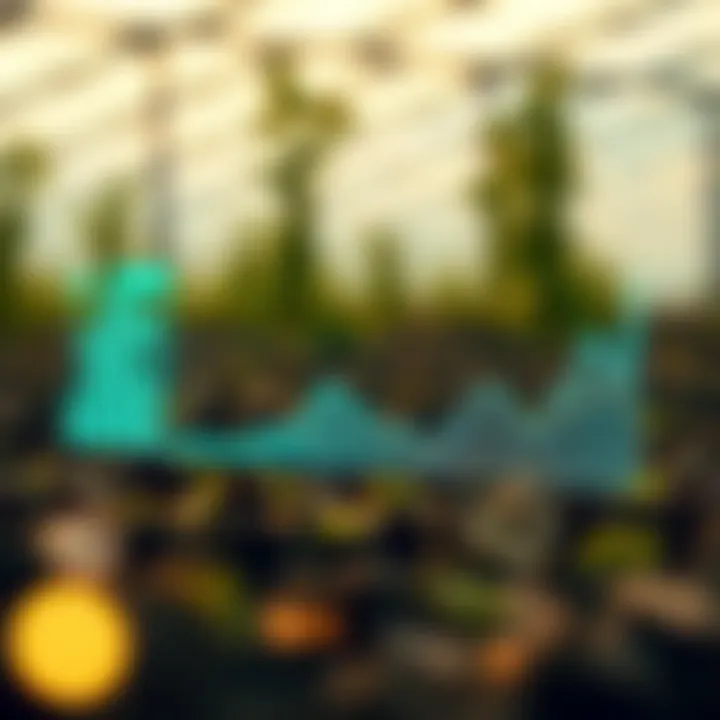
Data overload is a common concern among farmers today. With the abundance of information available, sifting through it all to find actionable insights can be daunting.
- Key Characteristic: The challenge here is not just the sheer volume of data, but also determining which pieces are relevant and useful.
- Unique Feature: Many farmers find themselves bombarded with notifications and updates from various data sources, which can lead to confusion rather than clarity.
- Advantages: There are tools designed to filter this information, yet it can require additional time and expertise to set up effectively.
Integration Issues
Integration issues arise when trying to combine data from various sources into a cohesive framework. Different systems and technologies may not be designed to work together smoothly, leading to gaps in information.
- Key Characteristic: The inability to seamlessly integrate data can result in missed opportunities for effective decision-making.
- Unique Feature: Organizations often rely on multiple platforms for specialized data, which can complicate analysis.
- Advantages: While a comprehensive understanding of data is crucial, the complexity of integration makes it harder for farmers to get a complete view of their operations. Simplifying these processes can open doors to more innovative solutions in farming.
"While big data holds the promise of revolutionizing agriculture, it must be approached with a balanced mindset to realize its full potential."
"While big data holds the promise of revolutionizing agriculture, it must be approached with a balanced mindset to realize its full potential."
Integrating big data into agriculture continues to be a journey filled with rewards and challenges. Consideration of both its opportunities and hurdles is necessary to navigate this ever-evolving domain effectively.
Ethics of Agriculture Data Use
In the age where data reigns supreme, understanding the ethics of agriculture data use is crucial. This section dives into the moral landscape that surrounds the collection, processing, and application of agricultural data. With an increasing reliance on technology, it's imperative that we examine how data practices impact not only the efficiency of farming but also the integrity of the agriculture community and its stakeholders.
Privacy Concerns
One of the pressing issues associated with agricultural data is privacy. Farmers are often hesitant to share their data due to fears that it might be misused or exploited. For example, if data collected from soil sensors or crop yields are directly shared with third-party companies, farmers may worry about the commercial exploitation of their practices. This concern is amplified by the increasing frequency of data breaches in various sectors. Farmers must feel assured that their personal and operational data are safeguarded against unauthorized access or potential misuse.
Here are a few examples of privacy concerns farmers might face:
- Loss of Control: How data is used or sold can lead to loss of agency over their own farming practices.
- Targeted Marketing: Companies might use personal data for aggressive marketing tactics, leading farmers to mistrust these entities.
- Regulatory Compliance: The need for adherence to data protection regulations can complicate how farmers collect and store agricultural data.
Data Ownership
Data ownership remains a contentious subject in agriculture. Who truly owns the data generated from farming practices? This complex question can influence how farmers approach data sharing. For instance, if a farmer opts to use a precision agriculture platform such as FieldView or Climate FieldView, is the data they generate solely theirs, or does the platform have claim to it?
There's a growing need for clearer guidelines around ownership. Without such regulations, farmers may find themselves in precarious situations where their data is treated as the property of a tech company rather than themselves.
Factors influencing data ownership include:
- Contracts with Technology Providers: Terms of service agreements can dictate data ownership rights unfairly.
- Interoperability Standards: Compatibility issues between platforms can further cloud ownership questions.
- Intellectual Property: As data becomes more integral to innovative farming techniques, understanding intellectual property rights is vital.
Implications for Farmers
The ethical considerations surrounding agriculture data use have significant implications for farmers. How they navigate these concerns can make or break their relationship with technology providers and consumers alike. For instance, transparent data-sharing policies can foster trust between farmers and consumers, ultimately enhancing market perceptions.
However, failure to address these concerns can diminish the willingness of farmers to adopt innovative practices, which in turn could hinder advancements in agricultural efficiency and sustainability.
Here are a few implications that may arise from neglecting ethical data considerations:
- Loss of Trust: If farmers feel their data is mishandled, it can create a widespread distrust towards technology.
- Economic Disadvantages: Data that could have bolstered market competitiveness can instead become a liability for farmers who fear exploitation.
- Regulatory Backlash: Failing to address ethical concerns may lead to stricter regulations, which could stifle innovation in data-driven agriculture.
As data interweaves deeper into farming practices, the onus is on industry stakeholders to foster an atmosphere of integrity.
As data interweaves deeper into farming practices, the onus is on industry stakeholders to foster an atmosphere of integrity.
By ensuring ethical guidelines are respected, the agricultural community can move forward without compromising its foundational values.
Future Trends in Agriculture Data
As we look toward the future, agriculture is on the brink of a major evolution driven by data. The importance of understanding future trends in agriculture data can't be overstated, as they hold the potential to reshape practices, increase efficiency, and promote sustainability. Through this exploration, we will delve into technological advancements shaping agriculture and sustainability initiatives that are vital for food security and environmental stewardship.
Technological Advancements
Blockchain in Agriculture
Blockchain is becoming a household term in various industries, and agriculture is no exception. This technology provides a decentralized and secure way of recording transactions. A key characteristic of blockchain in agriculture is its ability to ensure traceability. With blockchain, every transaction related to a product can be recorded transparently, from the farm to the table.
One benefit of utilizing blockchain is accountability. When consumers can trace their food back to its source, it increases trust between farmers and the public. However, a unique feature of blockchain is its inability to alter past records, which could be both an advantage and a drawback. This ensures authenticity but may pose challenges when correcting mistakes. Overall, the integration of blockchain in agriculture represents a significant step toward enhanced transparency in food supply chains.
AI and Automation
Artificial Intelligence (AI) coupled with automation is revolutionizing farming practices. The specific aspect of AI's machine learning capabilities allows for predictive analytics and decision-making based on real-time data. A distinguishing feature of AI and automation is efficiency; they can analyze vast amounts of data much quicker than any human could. This capability makes them a popular choice as farmers seek to optimize crops and reduce waste.
Yet, while AI has many advantages, including reducing labor costs, it also presents challenges. There is a learning curve, and not every farmer has immediate access to this technology. Nevertheless, as technology becomes more affordable, expect to see greater adoption of AI within the agricultural sector.
Sustainability Initiatives
Data-Driven Sustainability Practices
Data-driven sustainability practices are essential as the world grapples with climate change and the need for sustainable food production. Utilizing data allows farmers to make informed decisions on resource allocation, pest control, and crop rotation. A core characteristic of these practices is efficiency; by leveraging detailed data analytics, farmers can minimize resource waste.
A unique feature of data-driven practices is their adaptability. Farmers can respond to changing weather patterns and pest pressures more effectively. However, while the integration of data-driven practices has substantial advantages, the challenge lies in data accessibility and interpretation. The goal, however, is clear: to use data to create farming methods that harmonize with our environment.
Global Food Security
Global food security is intertwined with the advancement of agriculture data. This refers to the idea that everyone should have access to sufficient and nutritious food. A defining characteristic of global food security is its interactive nature; it involves farmers, governments, and institutions working in tandem. The influence of agriculture data here is critical in identifying food shortages and allocating resources accordingly.
The unique feature of fostering global food security through data is predictive modeling. By analyzing trends and risks, stakeholders can better anticipate and address food insecurity. Though daunting challenges persist, such as geopolitical tensions and supply chain disruptions, leveraging agriculture data holds significant potential to improve worldwide food distribution.
In sum, understanding future trends in agricultural data is vital as it lays the groundwork for innovations that could transform food production, promote sustainable practices, and move towards achieving global food security.
In sum, understanding future trends in agricultural data is vital as it lays the groundwork for innovations that could transform food production, promote sustainable practices, and move towards achieving global food security.