Statistics and Electricity: Bridging Data and Power
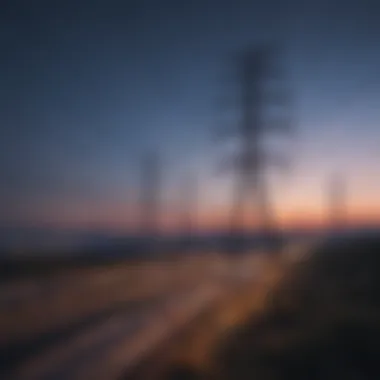
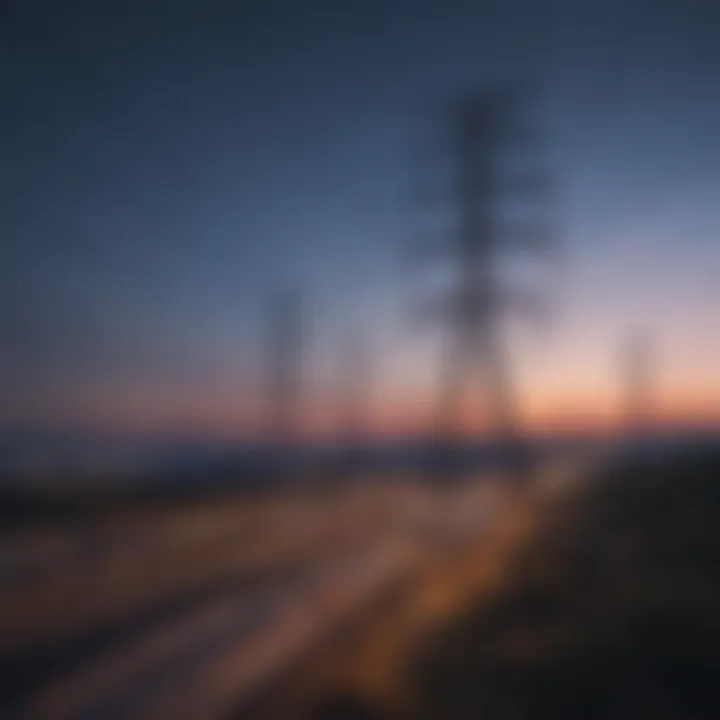
Intro
Electricity runs the world. From the light bulbs flickering in our homes to the massive power grids that fuel cities, electrons dance around us, quietly weaving through the fabric of modern life. But how do we understand this invisible force? Enter statistics—a tool that unveils trends and patterns, shedding light on the often complex behavior of electrical systems.
This exploration aims to bridge two seemingly disparate worlds: statistics and electricity. By equipping ourselves with statistical knowledge, we can not only measure demand and consumption but also optimize the way we generate and use energy. In a landscape that constantly shifts toward sustainable solutions, understanding the statistical principles behind electricity becomes paramount.
In this piece, we will delve into key concepts that underpin this integrative approach, examine current trends in research, and discuss their significance in an era where energy efficiency is king. As we navigate these interconnected disciplines, it becomes clear that deciphering electricity through a statistical lens is no longer just an academic endeavor; it's a necessity for a sustainable future.
Foreword to Electricity and Statistics
Electricity is a fundamental component of modern society. Its influence stretches from the flick of a switch in our homes to powering complex industries. Understanding how electricity operates is crucial for a multitude of stakeholders: policymakers, educators, and everyday consumers. Statistics emerge as a core tool in this exploration, allowing us to methodically analyze patterns, make predictions, and enhance efficiency. This intersection—the blend of electricity and statistical analysis—forms the backbone of effective research and practical applications in the energy sector.
In discussing the importance of this relationship, we unveil several key elements:
- Quantitative Analysis: Statistics offers methodologies to quantify data related to electricity, including consumption rates, peak usage times, and system reliability.
- Optimization: By utilizing statistical techniques, energy management can identify areas for improvement, minimizing waste and improving sustainability.
- Predictive Insights: Electricity usage is not static; it evolves. Statistical tools help forecast demand, aligning production with consumption and ensuring consistent supply.
This section will delve deeper into what electricity is, laying a solid foundation before we explore the statistical methods that support our understanding of its usage. By comprehending these principles, readers will appreciate how informed decisions are made in energy management and policy formulation.
Defining Electricity
Electricity, in simplest terms, is the flow of electric charge. It can manifest as static electricity, which is the accumulation of electric charge on surfaces, or as current electricity, which is the flow of electrons through a conductor. This latter form is what powers our devices and fuels our economies. Understanding this phenomenon involves grasping several key concepts:
- Voltage: The force that pushes electric charges through a circuit. In analogy, think of voltage akin to water pressure in a hose—higher pressure pushes more water.
- Current: The rate at which electric charges flow. This is similar to how much water flows through the hose at any given time.
- Resistance: It opposes the flow of current, much like a narrowing in a hose that restricts water flow.
Grasping these concepts allows one to not only comprehend phenomena such as power outages but also the design of efficient electricity systems.
The Role of Statistics in Scientific Research
The realm of electricity isn’t just about what happens; it’s about understanding why it happens. Enter statistics, the filtering lens through which data becomes knowledge. In scientific research, statistics play various crucial roles:
- Data Representation: Statistics helps in visualizing complex data sets. Charts and graphs can make it easier to identify trends in electricity consumption over time.
- Hypothesis Testing: Researchers formulate hypotheses about electricity use patterns and test them using statistical methods to determine whether findings are significant.
- Error Measurement: In any study, there’s always room for error. Statistical techniques provide methods to measure uncertainty, allowing researchers to understand the reliability of their conclusions.
- Correlation and Causation: Statistics aids researchers in exploring the relationship between different variables, helping to distinguish between mere correlation and true causation.
Statistics is the language that breathes meaning into electricity data. Without it, our understanding would be akin to wandering in the dark—illuminated only by disconnected numbers. By embracing statistical analysis, we pave the way for informed decision-making, better management practices, and innovative energy solutions that can adapt to the changing demands of society.
"Without data, you're just another person with an opinion." - W. Edwards Deming
"Without data, you're just another person with an opinion." - W. Edwards Deming
Together, let’s embark on this journey to demystify the relationship between electricity and statistics, fostering a clearer perspective for everyone involved in the energy discourse.
Statistical Principles Relevant to Electricity
Understanding statistical principles is a crucial endeavor when grappling with the complexities of electricity. These principles not only underlie the metrics that govern electrical systems but also shape the approaches taken for analysis and decision-making. The field of electricity, with its vast datasets and dynamic nature, benefits significantly from robust statistical methodologies. Here, we will explore the core statistical principles relevant to this domain and why they are essential for both practitioners and researchers alike.
Descriptive Statistics
Descriptive statistics serve as the first line of attack when analyzing electricity data. This branch of statistics is all about summarizing and organizing information in a way that makes it easily digestible. Think of it as a way to get the general gist of what's going on before diving into the nitty-gritty details.
Key elements of descriptive statistics include:
- Measures of central tendency: Mean, median, and mode help pinpoint the average electricity consumption or the most prevalent voltage level.
- Measures of dispersion: Standard deviation and range provide insights into variability in electricity usage. Are people in a neighborhood using far more energy than others? Descriptive stats shine a light on those disparities.
Through visual representations such as histograms and box plots, descriptive statistics allow us to spot patterns and outliers in energy consumption. For instance, if a particular region shows an unusual spike in energy usage, descriptive stats can help flag that for further investigation.
Inferential Statistics
While descriptive statistics lay the groundwork, inferential statistics take it a step further by allowing us to make predictions or generalizations about electricity consumption based on sample data. This is particularly useful when dealing with large populations, like an entire city or a national grid, where collecting data from every source isn’t feasible.
Important concepts in inferential statistics include:
- Hypothesis testing: Helps determine if observed phenomena, such as an increase in energy demand during summer months, are statistically significant.
- Confidence intervals: Provide estimates around the average energy use that give us a range of values, ensuring we understand the uncertainty involved in our estimates.
By leveraging these methods, policymakers can forecast energy needs and plan accordingly. If a utility company suspects that demand will rise in the coming month, they can utilize inferential statistics to back those claims with data.
"Statistical principles enable utility companies to anticipate demand trends, ultimately leading to better resource management."
"Statistical principles enable utility companies to anticipate demand trends, ultimately leading to better resource management."
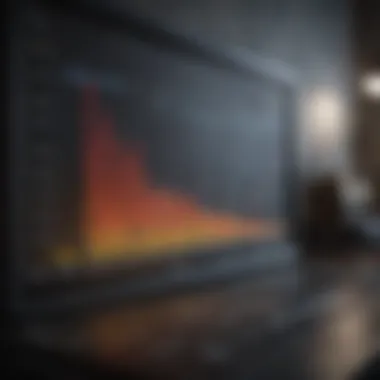
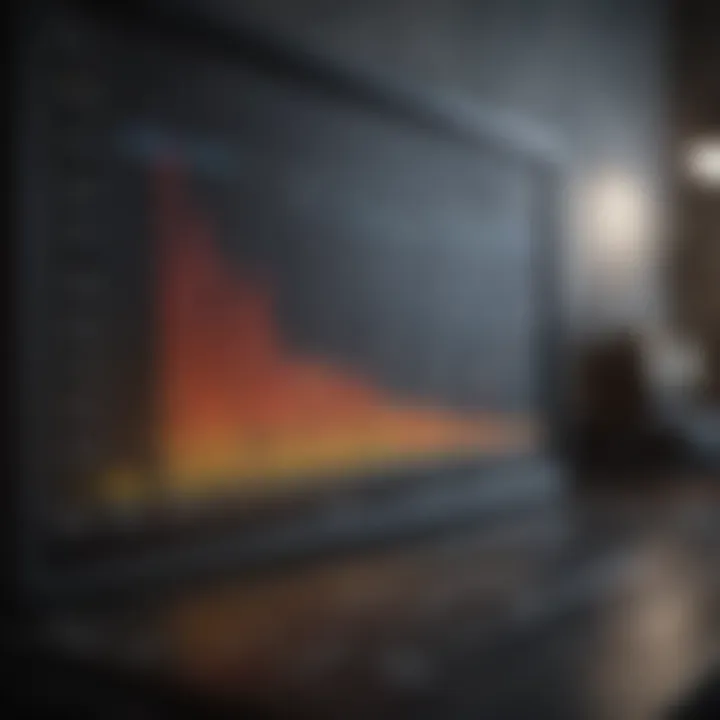
Regression Analysis
Finally, we get to regression analysis, a powerful statistical tool used to explore relationships between variables. In the context of electricity, regression can help uncover connections between various factors influencing energy consumption.
Key applications of regression analysis include:
- Predicting future demand: By analyzing past consumption patterns against variables such as temperature, time-of-year, and even local events, we can better forecast future electricity needs.
- Identifying influential factors: This makes it easier to pinpoint what drives energy use—whether it's the prevalence of electric vehicles or the adoption of energy-efficient appliances.
Using linear regression models, for example, researchers can analyze how a variable like the average daily temperature correlates with energy consumption across seasons. The outcomes inform strategies for energy conservation and management.
Data Collection Methods in the Electricity Sector
The collection of data within the electricity sector is essential. Without accurate and detailed information, it is challenging to make informed decisions. In today's age, understanding the nuances of electricity consumption, generation, and distribution can significantly impact policies and practices. The role of data collection goes beyond mere number crunching; it lays the groundwork for shaping energy-saving initiatives, optimizing grid performance, and planning for future energy needs.
Surveys and Public Records
Surveys can be a robust method for gathering information about electricity usage patterns. They can be designed to explore various aspects, from household consumption habits to industrial usage trends. By conducting surveys, utility companies can directly engage with customers and obtain firsthand data that might not be recorded elsewhere. This data can be used to assess customer satisfaction, understand service needs, and identify areas for improvement.
Public records, such as usage reports and outage logs, augment this qualitative information. These records allow researchers and policymakers to examine historical patterns of electricity supply and demand. Combining these two sources often yields a more comprehensive understanding of the energy landscape.
- Pros of Surveys and Public Records:
- Direct feedback from consumers
- Access to extensive historical data
- Ability to gauge public sentiment on energy issues
However, it's important to consider the limitations as well. Surveys often rely on self-reported data, which can lead to inaccuracies. Public records might not always be up to date or comprehensive. Hence, a balanced approach is necessary to triangulate findings from various sources. Each method carries its own weight, and combining insights provides a fuller picture of electricity consumption.
Sensor Data and IoT Devices
The proliferation of technology has transformed data collection in the electricity sector through the use of sensors and Internet of Things (IoT) devices. These tools provide real-time data on various electrical parameters, enabling faster responses to fluctuations in demand or supply. For instance, smart meters can record energy consumption at a granular level, offering insights into peak usage times.
IoT devices can also monitor the health of electrical infrastructure. Sensors placed on transmission lines can detect faults before they escalate into major issues, helping to enhance grid reliability. Analyzing this data can lead to predictive maintenance strategies, ultimately saving time and resources.
- Benefits of Sensor Data and IoT Devices:
- Real-time monitoring for immediate decisions
- Enhanced reliability of electrical systems
- Efficient resource management through predictive insights
Nonetheless, the implementation of these devices comes with considerations. Data privacy, security, and the cost of deployment are valid concerns. Moreover, integrating new technologies into existing systems requires careful planning and execution.
Thus, data collection methods in the electricity sector—whether through traditional surveys or cutting-edge IoT devices—play a pivotal role in shaping the future of energy management. Ultimately, they give stakeholders the tools and information necessary to navigate a complex and evolving landscape.
Electricity Consumption Patterns
Electricity consumption patterns are a cornerstone of understanding how power is utilized across various sectors. Recognizing these patterns helps in the efficient planning and management of electrical systems. Various variables come into play in assessing how electricity is consumed, providing valuable insights for utilities and consumers alike. Analyzing these patterns is particularly important for predicting demand, optimizing grid performance, and facilitating the integration of renewable energy sources.
When we talk about electricity consumption, it’s essential to note the diverse factors that influence usage. These can include economic conditions, technological advancements, and even cultural behaviors. By delving into these elements, we can uncover trends that guide energy policy and improvements in infrastructure. A well-analyzed consumption pattern leads to better demand forecasting which is crucial for sustaining the balance of supply and demand.
"Understanding consumption patterns opens the door to smarter energy management."
"Understanding consumption patterns opens the door to smarter energy management."
Understanding Usage Trends
Identifying usage trends enables us to draw parallels between different consumer groups. For instance, residential, commercial, and industrial sectors each have unique consumption behaviors. Going deeper into the data can reveal surprising insights. For example, a spike in electricity use on hot days often corresponds with higher demands for air conditioning. Conversely, evenings tend to see a rush in consumption as people return home and start their daily routines, illuminating how lifestyle choices drive demand.
The analysis can become more nuanced when one considers external factors like holiday seasons or special events, which could cause temporary surges in demand. Utilities can employ this trend analysis not only to manage the current supply but also to strategize on how best to allocate resources for future expansions.
Seasonal Variations in Electricity Demand
When it comes to seasonal variations, these trends are often predictable yet significant. During warmer months, the demand for electricity commonly increases due to air conditioning and cooling needs. In contrast, colder months may see a spike during periods that necessitate heating, potentially pushing demand peaks to hazardous levels if not managed properly.
Utilities can benefit from observing these seasonal trends, as they assist in scheduling maintenance for generating facilities during off-peak times. They allow for accurate modeling of energy needs, ensuring that providers can meet customer requirements without overextending resources. Adjusting generation strategies in tandem with seasonal forecasts leads to remarkable efficiencies in both cost-saving and service delivery.
In sum, grasping the nuances of electricity consumption patterns not only informs immediate operational decisions but also shapes long-term strategies for a sustainable energy future. Whether it’s planning a new power plant or integrating renewable energy sources, these insights form the bedrock of informed energy management.
Statistical Modeling to Predict Electricity Demand
Statistical modeling plays a pivotal role in predicting electricity demand, serving as a bridge between raw data and actionable insights. With the ever-increasing complexity of energy systems and consumption behaviors, having reliable models becomes essential. Through effective statistical models, utilities and policymakers can forecast demand peaks, allocate resources more accurately, and enhance grid stability. Moreover, informed predictions can lead to both economic savings and improved environmental outcomes by optimizing energy consumption.
Time Series Analysis
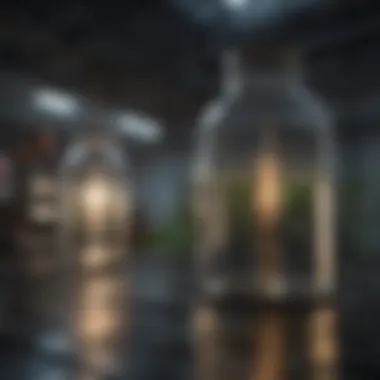
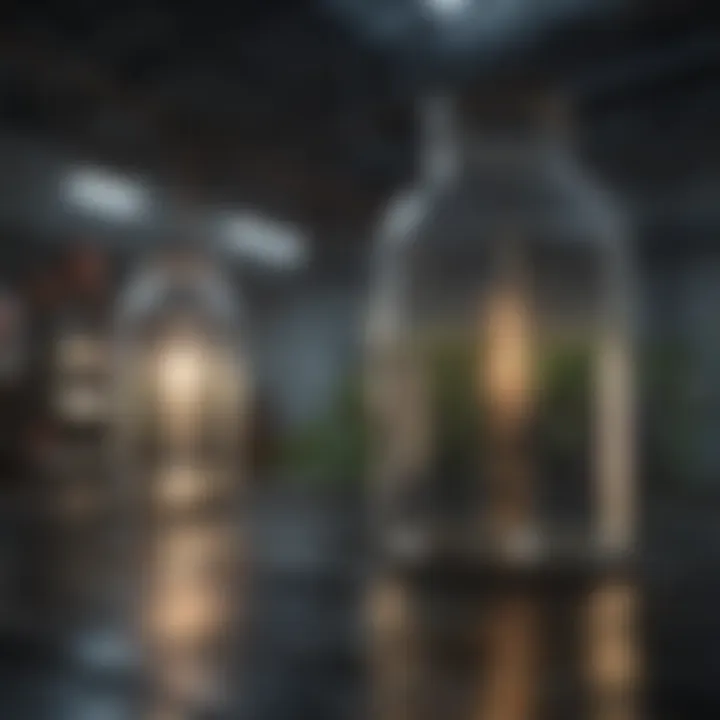
Time series analysis is a cornerstone technique in forecasting electricity demand. By examining historical data collected over a defined time period, it allows researchers and stakeholders to spot trends, seasonal patterns, and fluctuations. For instance, if one were to analyze the electricity consumption data from a metropolitan area over several years, you might find distinct spikes during summer months—which often correlate with increased air conditioning use.
To illustrate, consider the example where a utility company wants to predict demand for the upcoming summer. By employing time series analysis, they can leverage past consumption data, calculate the average temperature correlated to usage spikes, and identify any cyclical changes that happen yearly. This approach not only refines forecasting accuracy but also helps inform investment and infrastructure decisions. Here’s a simple model snippet for visual representation:
Notably, time series analysis also offers the ability to implement seasonal decomposition, wherein variations can be broken down into trend, seasonal, and residual components. This dissection can be incredibly valuable for long-term planning and operational strategies.
Machine Learning Algorithms in Demand Forecasting
As the data landscape evolves, the adoption of machine learning in demand forecasting adds another layer of sophistication. These algorithms can sift through vast amounts of data—more than conventional statistical methods can handle—allowing for more nuanced predictions.
A practical example is using regression models enriched with multiple input variables. By considering factors such as time of day, weather conditions, and historical trends, machine learning algorithms can generate forecasts that reflect the multifaceted nature of electricity consumption. For instance, a random forest algorithm might analyze hundreds of features to determine how demand changes with temperature, day of the week, and even special events like a local festival.
As an advantage, machine learning models can continuously improve over time by learning from new data, decreasing the likelihood of overfitting while enhancing prediction precision. In the long run, using these algorithms can significantly improve decision-making processes within utilities, ultimately benefiting consumers and the broader energy network.
Statistical modeling and forecasting are not just about predicting numbers; they empower a more sustainable and efficient energy system that adapts to evolving demand patterns.
Statistical modeling and forecasting are not just about predicting numbers; they empower a more sustainable and efficient energy system that adapts to evolving demand patterns.
In summary, the integration of statistical modeling, specifically through time series analysis and machine learning, reinforces the foundation for anticipating electricity demand. This framework not only drives efficiency in operations but also underpins innovations toward smarter energy systems.
Applications of Statistical Analysis in Grid Management
Understanding how statistical analysis plays a role in managing electrical grids is crucial in today’s energy landscape. As energy demand fluctuates and the integration of renewable sources increases, efficient grid management becomes a non-negotiable task for energy providers. By harnessing statistical methods, managers can improve decision-making, enhance system reliability, and optimize operations in ways that would’ve seemed impossible only a few decades ago.
Statistical analysis not only assists in real-time monitoring of the electric grid but also supports long-term strategic planning. Through detailed numerical insights, stakeholders can identify patterns in electricity consumption, predict future demand, and take preventive measures against potential disruptions. This intersection of data and electricity management highlights a pathway toward more resilient and adaptable energy systems.
Load Balancing and Optimization
One of the primary applications of statistical analysis within grid management is load balancing and optimization. During peak times, electricity demand can surge dramatically. If the grid isn't managed properly, it can lead to brownouts or even widespread outages. Load balancing ensures that electricity supply meets demand effectively, distributing power across various points in the grid based on statistical evaluations of usage patterns.
Regular analysis of consumption data helps in:
- Predicting Peak Times: By examining historical usage data, managers can foresee when demand is likely to spike, allowing them to prep the grid accordingly.
- Resource Allocation: Knowing usage trends enables utilities to allocate resources such as power generation from different plants, be it coal, natural gas, or renewable energy sources.
- Energy Efficiency: Statistical models can identify areas where energy is wasted, paving the way to increase efficiency and reduce costs for both providers and consumers.
Utilizing statistical algorithms can also aid in balancing intermittent energy sources like wind and solar, which may produce varying amounts of energy based on environmental conditions. This is where smart grids come into play, as they utilize real-time data analytics for continuous monitoring and adjustments.
Reliability Assessments
In the context of grid management, reliability assessments serve as a bedrock for ensuring that energy systems operate smoothly. Reliability refers to the probability that the electric supply will meet the anticipated demand under various conditions.
Statistical analysis cultivates reliability assessments by:
- Calculating Failure Rates: By tracking historical data on equipment malfunctions and outages, managers can determine the reliability of various components within the grid.
- Modeling Outages: Statistical techniques can simulate potential outage scenarios based on existing data, helping utilities understand the likely impacts of failures on the grid.
- Optimizing Maintenance Schedules: With the insights obtained through statistical evaluations, maintenance can be conducted in a proactive manner, rather than a reactive one. Every piece of equipment operates differently, and understanding these nuances through data can minimize the risk of unexpected failures.
"Statistical analysis transforms raw data into actionable insights, enabling utilities to enhance grid reliability and operational efficiency."
"Statistical analysis transforms raw data into actionable insights, enabling utilities to enhance grid reliability and operational efficiency."
Statistical Insights into Renewable Energy Sources
The integration of renewable energy sources like solar and wind is crucial both for sustainability and for the stability of our electric systems. As the world moves to reduce its carbon footprint, the importance of statistical insights can’t be overstated. By understanding the data behind these renewable sources, we can make informed decisions about energy production, consumption, and optimization.
Analyzing Solar and Wind Energy Outputs
When evaluating solar and wind energy, statistical analysis offers a clear view of performance trends and outputs. These insights reflect various factors - such as weather conditions, geographic considerations, and time of day - that significantly impact energy generation. Here's how we can break down the analysis:
- Data Collection: Harnessing data from solar panels and wind turbines is step one. This includes real-time measurements of energy output, operational efficiency, and environmental factors.
- Descriptive Statistics: By using measures like mean output, standard deviation, and variance, we can summarize how each system performs over time. For instance, a solar panel in Arizona might generate significantly more energy in summer than a wind turbine in a coastal area during winter.
- Visual Representations: Charts and graphs can provide visual imprints of these patterns, making it easy to compare the efficacy of different energy sources over set periods. The clearer these representations, the better stakeholders can understand the data.
"Statistical insights allow us to dissect energy data, illustrating not only where we are, but also where we can go."
"Statistical insights allow us to dissect energy data, illustrating not only where we are, but also where we can go."
Predictive Analytics for Renewable Integration
Looking ahead, predictive analytics serves as a powerful tool for anticipating energy outputs and optimizing resource allocation. It enables stakeholders to foresee how factors will influence energy generation in the future. Key components include:
- Time Series Analysis: Analyzing historical data to identify trends over time. This technique is pivotal in forecasting future energy outputs based on past performance.
- Machine Learning Models: By employing algorithms that can learn and adapt, energy providers can improve their predictions. For instance, by training a model with coastal wind patterns, the predictive accuracy may enhance, allowing for better planning.
- Scenario Analysis: This involves creating simulations based on varying conditions, such as changes in environmental policy or technological advancements. Stakeholders can evaluate potential impacts on energy output, which is essential for strategic decision-making.
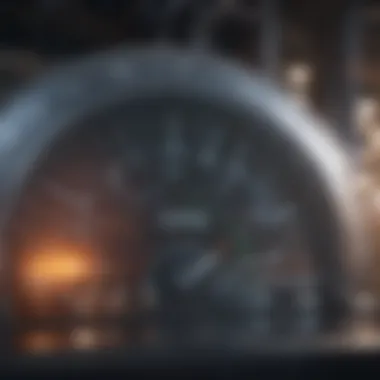
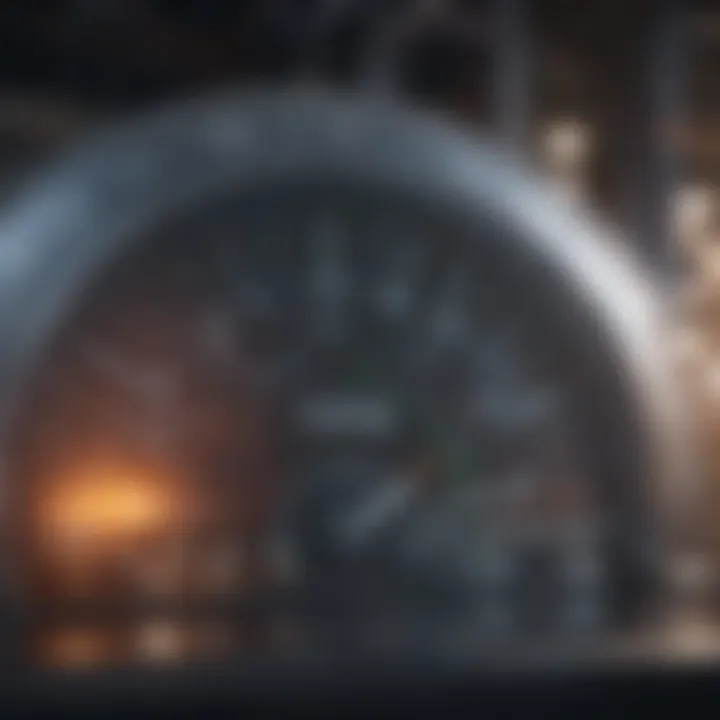
The interplay of these statistical methods reveals a clearer picture of how renewable energy can be effectively integrated into electric systems. By leveraging these tools, society can better prepare for a future that embraces sustainable practices while ensuring reliability in energy supply.
Challenges and Limitations of Statistical Approaches
Statistical methods are invaluable tools in the field of electricity management. However, like any other analytical approach, they come with their quirks and hurdles. Understanding these challenges is key to harnessing statistics effectively. Delving into data quality issues and the risks of overfitting provides a clearer picture of the landscape in which these analyses operate. This knowledge equips researchers and professionals with the foresight needed to navigate the complexities of electricity statistics accurately.
Data Quality and Accuracy Issues
When it comes to data collection in the electricity sector, the old adage "garbage in, garbage out" rings particularly true. The reliability of statistical outcomes hinges significantly on how well the data has been gathered and curated. Poor data quality can stem from several sources, such as:
- Inconsistent Data Entry: Data might be recorded differently across systems, creating an inconsistent dataset.
- Missing Values: Incomplete information can lead to misinterpretations while being analyzed.
- Faulty Sensors: If sensors in smart grids or IoT systems malfunction, the data they provide could be wildly inaccurate.
The implications of these quality issues are far-reaching. If a model is constructed from flawed data, the predictions generated could lead stakeholders astray, impacting decision-making processes and strategies. For instance, a forecast that inaccurately shows a spike in consumption could prompt unnecessary grid enhancements, wasting resources. Therefore, three critical practices are vital in addressing data quality issues:
- Regular Audits: Consistent reviews of data collection processes are essential to maintain accuracy.
- Validation Checks: Implementing validation rules can catch errors before they skew results.
- Training Personnel: Those involved in data entry should be well-trained to minimize mistakes.
Overfitting in Predictive Models
As data analytics becomes increasingly prominent, the tendency to overfit predictive models poses a significant challenge. Overfitting occurs when a model learns not only the underlying patterns but also the noise found in the data. This situation often arises when:
- Models are too complex for the amount of data available.
- Too many parameters are included in the modeling process.
- The training data is not representative of the broader trends.
The ramifications of overfitting can be dire—it can result in models that perform brilliantly on historical data but falter when applied to new or unseen situations. Imagine a company investing heavily in predictive maintenance for electrical equipment but basing their strategy on an overfit model. The result could lead to inefficient maintenance schedules, missed opportunities for energy savings, and ultimately, higher operational costs.
To combat overfitting, several strategies can be employed:
- Simplifying Models: Keeping the model complexity in check can yield more robust predictions.
- Cross-Validation: Dividing the dataset into training and validation sets ensures the model generalizes well.
- Regularization Techniques: These help maintain a balance between fitting the training data well while not adapting too closely to it.
The Future of Statistical Applications in Electricity
The rapid evolution of technology has transformed many sectors and electricity is no exception. As we look forward, the integration of statistics into the electricity domain holds tremendous potential. This section explores why the future of statistical applications in electricity is critical. The focus is on three aspects: innovations in big data analytics, the emergence of smart grids, and their consequent impact on energy management and sustainability.
Innovations in Big Data Analytics
Big data is revolutionizing how we approach electricity management. With the advent of various data collection methods, such as smart meters and IoT devices, the ability to gather vast amounts of information has grown exponentially. This data explosion enables utilities and researchers to analyze patterns and anomalies like never before.
For instance, consider a city experiencing fluctuating electricity consumption. By employing big data analytics, energy companies can identify specific factors contributing to spikes in usage—perhaps local events or weather changes. This identification allows for better forecasting and more responsive management of resources. The predictive capabilities of big data not only improve operational efficiency but also enhance customer satisfaction by minimizing outages and optimizing service responses.
The integration of methodologies such as machine learning with traditional statistical techniques further enhances the analytical power at our disposal. By continuously learning from new data sets, models become more precise, leading to actionable insights that drive energy innovations.
Emerging Trends in Smart Grids
Smart grids represent a massive leap forward when it comes to electricity distribution and consumption. The traditional electricity grid is often likened to an ancient one-size-fits-all system. In contrast, smart grids use advanced communications technology to create a more dynamic, efficient structure. Today, integrating statistical insight into their operation is not just an innovation but a necessity.
Through real-time data analysis, smart grids enable better resource allocation. For example:
- Demand Response Programs: These allow consumers to adjust their usage based on grid conditions, often incentivized by lower rates during off-peak hours.
- Distributed Energy Resources (DER): The ability to manage decentralized energy sources, such as solar panels or battery storage, requires an adaptive statistical framework for stability and reliability.
Furthermore, as we adopt electric vehicles on a larger scale, their charging patterns can heavily influence grid performance. Statistical systems must monitor these changes closely and adapt accordingly. All in all, the future landscape is geared towards successful integration via smart grids.
"The increasing reliance on data-driven solutions for electricity management reflects a growing recognition of the critical role of statistics in enabling a sustainable energy future."
"The increasing reliance on data-driven solutions for electricity management reflects a growing recognition of the critical role of statistics in enabling a sustainable energy future."
The intersection of big data and smart grids is paving the way for significant advancements, not only in energy efficiency and operational effectiveness but also in enhancing the overall sustainability of the energy ecosystem. By embracing these innovative statistical applications, we stand not just to improve current practices but also to set the stage for future energy developments—developments that are efficient, reliable, and ecologically sound.
Ending
In this article, the exploration of statistics in the realm of electricity brings to light its indispensable role in shaping future energy policies and grid management. Understanding the quantitative aspects of electricity not only enhances our ability to analyze current consumption trends but also assists in forecasting future demands. This prediction can lead to optimized operations and a reduction in energy waste, which is crucial as people increasingly seek sustainable solutions.
The key takeaways from the various discussions are significant. Firstly, the integration of statistical modeling into electricity demand forecasting holds the potential to fine-tune energy distribution, particularly during peak usage times. Secondly, it enables a more nuanced understanding of consumer behaviors and their impact on the overall energy economy.
"Statistics is like the sun; it shines on everything, revealing the patterns every single time."
"Statistics is like the sun; it shines on everything, revealing the patterns every single time."
Key Takeaways:
- Statistical methods provide insights into consumption patterns, helping in load forecasting and mitigating overload risks.
- Advanced analytics, including machine learning techniques, are increasingly pivotal in demand forecasting.
- Recognition of data quality and relevance is essential in ensuring that statistical analyses yield meaningful insights.
Implications for Policy and Practice:
- Policymakers can leverage statistical insights to formulate evidence-based regulations aimed at enhancing the efficiency of the electric grid.
- There is a pressing need for investment in data collection technologies to streamline the acquisition of accurate information, enabling more reliable analyses.
- Moreover, tapping into community-level data can help tailor local energy strategies that address specific challenges and opportunities in diverse regions.
As we look to the future, it becomes critical to continuously refine our statistical approaches and adapt our policies based on emerging trends in energy consumption and production. This dynamism will not only support a transition to renewable energy sources but also ensure the resilience of our electrical systems in the face of evolving technological and environmental challenges.