Exploring Clinical Data Review Tools: A Comprehensive Overview

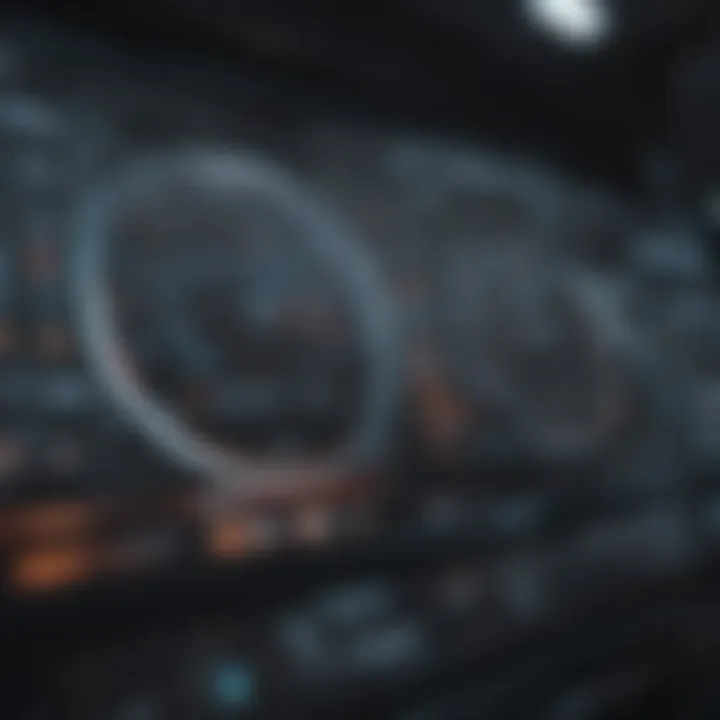
Intro
In the evolving field of clinical research, the analysis and scrutiny of data is crucial. It can significantly influence clinical outcomes, ensure patient safety, and facilitate regulatory approvals. Clinical data review tools serve as essential instruments in this process. This article aims to provide insights into various types of these tools, their functionalities, and the technological advancements shaping the future of data review in healthcare and research environments.
Key Concepts
Definition of Clinical Data Review Tools
Clinical data review tools are software applications and platforms that assist researchers in evaluating the integrity, accuracy, and completeness of clinical data. These tools enable users, such as clinical researchers and healthcare professionals, to analyze large sets of data efficiently. They streamline processes by employing algorithms and analytics, allowing for better identification of trends and anomalies.
Overview of Scientific Principles
The principles behind these tools are rooted in statistical analysis, data mining, and regulatory compliance. They utilize methodologies such as:
- Data Validation: Ensuring accuracy and compliance of collected data.
- Statistical Analysis: Applying statistical methods to interpret complex data sets.
- Quality Control: Maintaining data integrity throughout the research process.
The intersection of technology with these principles has led to innovative solutions that enhance data usability and foster informed decision-making in clinical trials.
Current Research Trends
Recent Studies and Findings
The field of clinical data review is constantly evolving. Recent studies indicate that tools that utilize artificial intelligence can greatly improve data monitoring and review efficiency. These modern approaches not only reduce human errors but also speed up the time required for data evaluation.
Significant Breakthroughs in the Field
Several breakthroughs have been noted, including:
- Enhanced data visualization techniques that simplify interpretation.
- Integration of machine learning algorithms for predictive analysis.
- Improved user interfaces that increase accessibility.
As these tools continue to advance, they address the challenges posed by increasing data volumes and complexity, ultimately supporting better decision-making in clinical research.
Preface to Clinical Data Review Tools
Clinical data review tools play a vital role in the landscape of clinical research. Their importance cannot be overstated, as they provide structured methodologies for analyzing vast amounts of health data. These tools enhance accuracy, ensure compliance, and facilitate efficient data management. The rise of advanced clinical data review instruments signifies a shift towards more data-driven decision-making processes in medicine. Clinical trials and research studies produce extensive datasets, requiring effective tools to review and utilize this information efficiently.
Defining Clinical Data Review Tools
Clinical data review tools refer to software and platforms that assist researchers in analyzing, managing, and validating clinical data. These tools can encompass various functionalities, from basic data handling to complex statistical analysis. They often integrate features that allow for data cleaning, real-time monitoring, and actionable reporting. In essence, these tools serve as fundamental assets in modern clinical research by transforming raw data into informed insights.
Importance in Clinical Research
The significance of clinical data review tools in clinical research is profound. These tools contribute to the reliability of clinical trials by ensuring that the data collected is accurate and compliant with regulatory standards. They bolster the integrity of findings, which can influence treatment protocols and patient care.
Moreover, efficient data review processes streamline research workflows. This efficiency translates to shorter timelines for study completion, which is critical in fast-paced medical environments. In the context of rising health challenges, timely insights from clinical research can lead to quicker therapeutic advancements.
"Implementing robust clinical data review tools is not just a recommendation; it is an essential strategy for achieving data integrity and compliance in research."
"Implementing robust clinical data review tools is not just a recommendation; it is an essential strategy for achieving data integrity and compliance in research."
In summary, with the increasing complexity of clinical trials and regulatory requirements, the integration of clinical data review tools becomes not only beneficial but necessary for maintaining the quality and reliability of research outcomes.
Types of Clinical Data Review Tools
The classification of clinical data review tools offers insights into their specific roles and functionalities in clinical research. Understanding the various types enhances comprehension of how they can elevate data quality and streamline the research process. Each category brings unique elements that cater to distinct needs within the clinical data review landscape. This section will cover the key types of tools, providing clarity on their individual significance.
Statistical Analysis Software
Statistical Analysis Software, such as SAS or R, is essential for processing and analyzing clinical data. These tools provide a wide array of statistical tests and models, facilitating quantitative analysis. They help researchers understand trends, correlations, and significant outcomes from collected data. The capabilities of these software programs include:
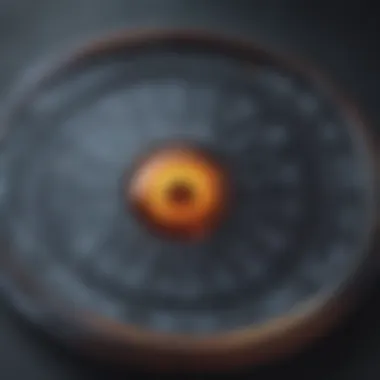
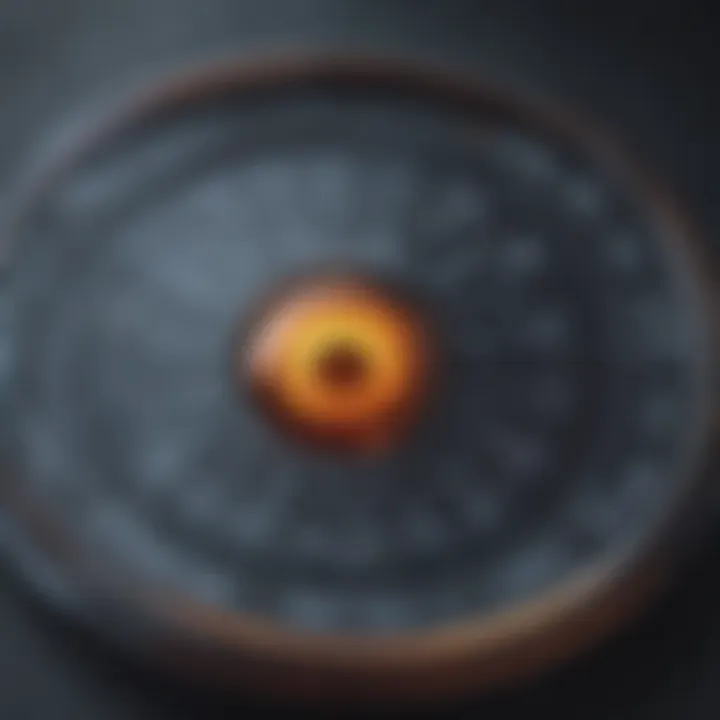
- Data summarization: Ability to compute means, medians, variances, and standard deviations.
- Hypothesis testing: Supports conducting t-tests, ANOVAs, and regression analyses.
- Predictive modeling: Enabling risk assessment and patient outcome prediction through logistic regression or survival analysis.
Utilizing statistical analysis software not only enhances the credibility of findings but also assists in regulatory submissions by providing rigorous documentation.
Data Visualization Tools
Data Visualization Tools are vital for effectively communicating clinical data insights. Tools like Tableau or Microsoft Power BI transform complex datasets into intuitive visual formats. This adaptation is crucial for stakeholders and researchers who need to comprehend data quickly. The benefits of data visualization tools include:
- Simplified understanding: Graphs, charts, and dashboards make intricate data accessible.
- Enhanced collaboration: Visual representations foster conversations among team members.
- Real-time insights: Many tools provide updates as new data is added, allowing for immediate interpretation.
In this age of information, visual clarity is paramount for facilitating informed decision-making in clinical scenarios.
Electronic Data Capture Systems
Electronic Data Capture (EDC) Systems, such as Medidata or Oracle's InForm, are revolutionizing how clinical data is collected, managed, and stored. These systems streamline data entry and minimize errors linked to manual data handling. Key features include:
- Centralized data collection: All data is consolidated in one platform, improving accessibility.
- Audit trails: EDC systems maintain detailed logs of data changes, supporting transparency.
- User-friendly interfaces: Intuitive designs reduce training time and enhance user engagement.
Efficient data collection is crucial to successful clinical trials, making EDC systems indispensable.
Clinical Trial Management Systems
Clinical Trial Management Systems (CTMS) play a pivotal role in organizing and overseeing various aspects of clinical trials. Platforms like Veeva Vault or Medidata's Clinical Cloud provide solutions for study planning, monitoring, and reporting. The advantages of utilizing a CTMS include:
- Workflow management: Streamlines interactions among researchers, sites, and sponsors.
- Resource tracking: Helps manage trial budgets, timelines, and personnel assignments.
- Regulatory compliance: Facilitates adherence to guidelines by managing necessary documentation efficiently.
These systems are integral to synchronizing the complexities involved in clinical trials, ensuring that every phase is executed with precision and oversight.
Functionalities of Clinical Data Review Tools
The functionalities of clinical data review tools are vital for enhancing the efficiency and effectiveness of data analysis in clinical research. These tools help ensure that the processes of data cleaning, integration, monitoring, and reporting are executed smoothly. A well-functioning data review tool maximizes accuracy and reduces errors, leading to reliable results for studies. Here, we will explore four key functions: data cleaning and validation, integration with existing systems, real-time data monitoring, and reporting and insights generation.
Data Cleaning and Validation
Data cleaning and validation are foundational aspects of clinical data review tools. Accurate data collection is paramount; however, inconsistencies and errors can occur during data entry. Tools focus on identifying discrepancies in datasets, such as missing values or incorrect formatting. By employing algorithms for validation, they ensure that the data adheres to predefined standards before it is analyzed. This step is essential as it directly influences the quality and reliability of the subsequent analysis. Regular cleaning helps maintain the integrity of data over the research lifecycle.
Integration with Existing Systems
Integration with existing systems reflects the interoperability of clinical data review tools with various platforms utilized in clinical research. Many organizations employ diverse software for data collection and management. Thus, it's important that review tools effectively communicate with these systems, like Electronic Data Capture (EDC) systems or Clinical Trial Management Systems (CTMS). Integration minimizes redundancy and fosters a seamless workflow. It ensures that data management processes are efficient and centralized, thus saving time and resources.
Real-time Data Monitoring
Real-time data monitoring is a critical function of clinical data review tools. With the increasing volume of data being generated, the need for timely insights becomes more apparent. These tools provide researchers with the capability to observe data flow continuously, allowing for immediate detection of anomalies or trends as they occur. This proactive approach aids in informed decision-making, improving overall data management and patient safety.
Reporting and Insights Generation
Reporting and insights generation are the culmination of effective data management processes. Clinical data review tools offer sophisticated reporting functionality, transforming raw data into insightful summaries. These summaries play a pivotal role in presenting findings to stakeholders, facilitating regulatory submissions, or guiding strategic planning. Moreover, insightful graphics and visualizations can enhance comprehension and retention of complex data, making results accessible to a broader audience.
Effective functionalities in clinical data review tools provide a robust framework for ensuring comprehensive data integrity and fostering informed decision-making throughout the research process.
Effective functionalities in clinical data review tools provide a robust framework for ensuring comprehensive data integrity and fostering informed decision-making throughout the research process.
Regulatory Considerations
Regulatory considerations play a critical role in the effective utilization of clinical data review tools. These considerations are essential for ensuring the integrity, security, and compliance of data used in clinical research. As the landscape of healthcare and clinical research evolves, adhering to regulations is not only a legal obligation but also a fundamental aspect that enhances the quality and reliability of clinical outcomes.
Understanding and implementing regulatory frameworks helps institutions safeguard patient data, foster trust, and promote transparency in research processes. Non-compliance can lead to severe consequences, including financial penalties and reputational damage. Therefore, a detailed grasp of relevant regulatory guidelines is necessary for researchers and organizations involved in clinical trials.
Compliance with GCP Guidelines
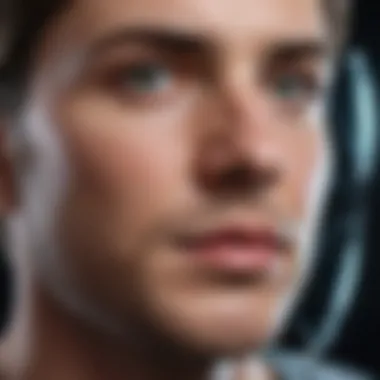
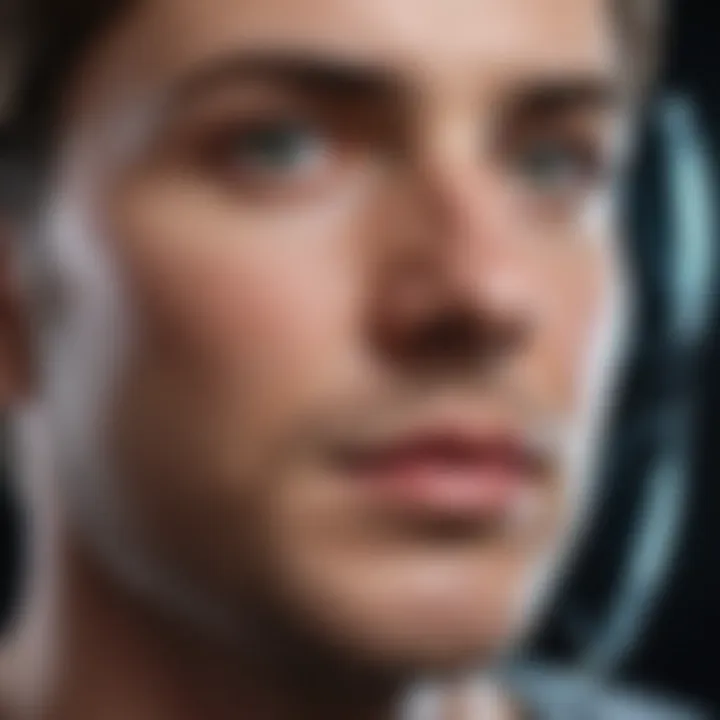
Good Clinical Practice (GCP) guidelines are a cornerstone of regulatory compliance in clinical research. These guidelines provide a framework to ensure that clinical trials are designed, conducted, and reported in a manner that protects participants' rights and leads to credible data. GCP emphasizes the necessity of following ethical standards, which are paramount when dealing with human subjects. Organizations must implement measures that ensure compliance, including thorough monitoring and documentation of trial procedures.
Ensuring compliance with GCP can significantly improve the validity of trial results. It facilitates better data handling, allows for easier identification of data discrepancies, and reinforces data integrity. Institutions that adhere to GCP guidelines also enhance their reputation within the research community. For researchers, having a strong GCP compliance framework translates into reliable and reproducible findings, which are crucial for advancing medical knowledge.
Data Privacy Regulations
Data privacy regulations are vital for protecting sensitive patient information in clinical research. These regulations provide the necessary guidelines to safeguard personal data against misuse. They are designed to enhance individuals' autonomy and control over their information, ensuring that it is only used in ways they consent to.
GDPR Compliance
The General Data Protection Regulation (GDPR) is one significant legal framework in this sphere. Its main contribution is to establish stringent standards for data protection and privacy in the European Union. A key characteristic of GDPR is its emphasis on consent; researchers must obtain explicit agreement from participants before using their data. This makes GDPR a popular choice among institutions aiming to enhance their data handling practices.
A unique feature of GDPR is the right to erasure, also known as the right to be forgotten, which allows individuals to demand the deletion of their data in certain circumstances. This empowers patients, enhancing trust in clinical research. However, complying with GDPR can be resource-intensive, requiring organizations to implement robust data management practices and processes.
HIPAA Regulations
The Health Insurance Portability and Accountability Act (HIPAA) is another critical regulation governing data privacy in health-related research in the United States. Its main focus is on protecting health information from unauthorized access. A defining characteristic of HIPAA is its requirement for covered entities to ensure the confidentiality, integrity, and availability of protected health information (PHI). Its provisions are beneficial for organizations as they foster accountability and help in building public confidence.
A unique aspect of HIPAA is establishing a minimum necessary standard, which limits the use or disclosure of PHI to only what is required for a specific purpose. This helps in minimizing the risk of data breaches. Nonetheless, implementing HIPAA's compliance requirements can pose challenges, often necessitating significant investments in training and technology to safeguard information effectively.
Understanding these regulatory considerations equips professionals with the knowledge to navigate the complexities of clinical data review effectively, ensuring both compliance and ethical integrity in research practices.
Challenges in Utilizing Clinical Data Review Tools
The application of clinical data review tools is not without its difficulties. Understanding these challenges is crucial for enhancing the effectiveness of these instruments in clinical research and healthcare. The barriers can range from issues in data standardization to complexities involved in system integration. Addressing these challenges ensures that the full potential of clinical data review tools is realized in improving research integrity.
Data Standardization Issues
Data standardization is the process by which disparate data sources are harmonized to ensure consistency across datasets. This is critical in clinical research where data typically originates from numerous clinical sites and systems. Without standardization, analyzing data becomes problematic, leading to errors and misinterpretations.
- Variability in Data Formats: Clinical data is often collected in various formats depending on the sources. This variability complicates data aggregation and can lead to data loss or inaccuracies.
- Lack of Common Terminology: Different institutions may use disparate terminologies for similar clinical concepts. This inconsistency can result in difficulties during the data analysis phase as algorithms misinterpret data.
- Challenge in Compliance: Regulatory agencies expect data to be standardized to meet necessary compliance requirements. Inconsistencies can lead to failures in audits or regulatory assessments.
User Training and Adoption Barriers
The effectiveness of clinical data review tools heavily relies on the users' ability to utilize them properly. Therefore, user training and the overall adoption of these tools present significant challenges that need to be addressed.
- Technical Proficiency Requirement: A high level of technical know-how is often necessary for users to navigate complex software. This can deter less experienced personnel from adopting the tools.
- Training Programs: The absence of effective training programs can contribute to a reluctance to fully engage with these tools. Regular workshops and hands-on training sessions can mitigate this issue.
- Cultural Resistance: Existing workflows may also influence the adoption of new tools. If personnel are accustomed to established processes, introducing new tools can meet with resistance, decreasing overall productivity.
Integration Complexity
Integration complexity arises when clinical data review tools must work together with existing systems. This challenge may lead to inefficiencies and hinder the full utilization of these tools.
- Diverse Systems: Organizations may employ various systems for data collection, analysis, and reporting. Ensuring that clinical data review tools can seamlessly integrate with these different systems can be a daunting task.
- Resource Investment: Significant resources may be needed to reconfigure existing infrastructures to accommodate new tools. This includes not only financial investment but also time and personnel training.
- Data Security Risks: Integration can expose organizations to potential data security risks if not carefully managed. Ensuring data protection protocols are in place during integration is essential to safeguard sensitive information.
Addressing these challenges is critical to fostering an environment where clinical data review tools can thrive, ultimately enhancing the quality of clinical research.
Addressing these challenges is critical to fostering an environment where clinical data review tools can thrive, ultimately enhancing the quality of clinical research.
Best Practices for Effective Clinical Data Review
Effective clinical data review is fundamental for ensuring the integrity and reliability of research outcomes. Practicing robust methods allows teams to maximize the potential of their data review tools. Therefore, adherence to proven best practices is crucial for achieving accurate and actionable insights.
Establishing a Standard Operating Procedure
Creating a Standard Operating Procedure (SOP) is essential for any clinical data review process. An SOP provides a clear framework for data handling, analysis, and reporting. It ensures consistency across teams, reducing the risk of errors and improving efficiency.
Key benefits of establishing an SOP include:
- Clarity: An SOP eliminates ambiguity in processes, making it easier for team members to understand their roles and responsibilities.
- Compliance: Following a documented procedure helps in adhering to industry regulations and guidelines.
- Training Efficiency: New team members can be onboarded more quickly when clear guidelines are in place.
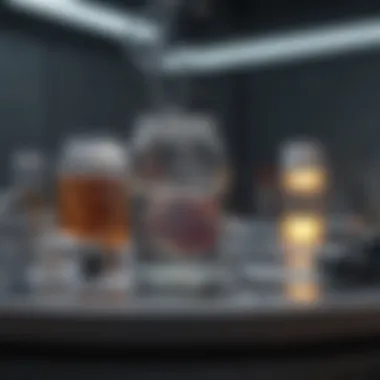
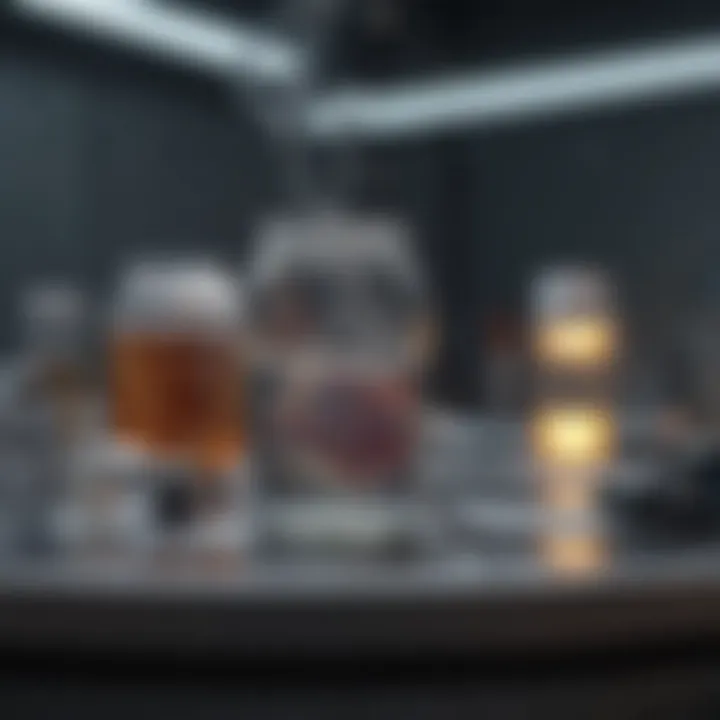
SOPs should be regularly reviewed and updated to reflect changes in technology, regulations, or internal processes. The creation of a living document helps organizations stay adaptable and aligned with best practices.
Regular Audits and Quality Checks
Regular audits and quality checks are vital components in maintaining the integrity of clinical data. These practices help ensure that data is accurate, complete, and reliable over time.
Audits can identify deviations from the established SOP and help in recognizing potential issues early. Key elements include:
- Frequency: Audits should be scheduled regularly to capture any developing trends or anomalies.
- Documentation: Maintaining comprehensive records of audit findings is critical for accountability and continuous improvement.
- Action Plans: Following an audit, it is important to develop a detailed action plan. This plan should address any identified issues and outline steps for remediation.
Quality checks, such as data validation and verification processes, are also crucial. These checks can catch errors before they can propagate, safeguarding the overall quality of the data.
Investing in User Training and Support
User training is often overlooked but is necessary for the successful implementation of clinical data review tools. Well-trained personnel will understand not only how to use tools effectively but also how to interpret the data they generate.
Considerations for an effective training program include:
- Tailored Content: Training should be tailored to the needs of different user groups, from data entry staff to managers.
- Interactive Learning: Workshops or hands-on sessions can enhance learning by allowing users to become familiar with the tools in a practical setting.
- Ongoing Support: Providing continuous support and refresher courses can help maintain high levels of competence amongst team members.
By investing in user training and support, organizations can ensure that their clinical data review tools are utilized to their full potential, ultimately leading to better research outcomes.
Regular audits and proper training significantly enhance the accuracy and reliability of clinical data review.
Regular audits and proper training significantly enhance the accuracy and reliability of clinical data review.
Future Trends in Clinical Data Review Tools
The landscape of clinical data review tools is evolving rapidly due to technological advancements and the growing emphasis on data-driven decision-making. Understanding these future trends is crucial for professionals in the field, as they promise to enhance accuracy, efficiency, and overall research outcomes. The integration of cutting-edge technologies will reshape how clinical data is collected, analyzed, and reported. Those involved in clinical research must stay informed of these trends to leverage new opportunities and navigate challenges effectively.
Artificial Intelligence and Machine Learning Integration
Artificial intelligence (AI) and machine learning (ML) are poised to revolutionize clinical data review tools. By applying algorithms to vast datasets, AI can identify patterns and insights that might be missed by traditional methods. This capability enhances predictive analytics, leading to more informed clinical decisions. For instance, AI can analyze patient data to predict outcomes, personalize treatment plans, and improve patient recruitment. However, this integration must be approached cautiously. The ethical implications of data usage and the need for transparency in AI decision-making processes cannot be overlooked. Maintaining compliance with regulations while harnessing these technologies is critical.
Increased Customization and User Experience
As end-user needs evolve, there is a growing demand for customization in clinical data review tools. Users want interfaces that cater to their specific requirements, whether they are data managers, researchers, or clinical trial coordinators. Customized dashboards and reporting features can streamline workflows and improve engagement with the data. Enhanced user experience can lead to better data interpretation and faster decision-making processes. Companies must focus on user-centered design principles to ensure their tools are intuitive and effective. In doing so, they can also reduce the barriers associated with user training and adoption.
Emerging Technologies in Data Analysis
Technological advancements in data analysis are reshaping clinical research protocols. The rise of cloud computing and big data analytics enables researchers to handle larger datasets with ease. Tools that support real-time data processing allow for quicker responses to changing circumstances during trials. This shift can lead to more dynamic research processes, where modifications can be made swiftly based on interim results. Furthermore, the incorporation of blockchain technology promises enhanced data security and integrity, addressing long-standing concerns in clinical research. These emerging technologies will not only improve current practices but will also pave the way for innovative methodologies in future clinical trials.
"The integration of AI, customization, and emerging technologies in clinical data review tools is an exciting frontier that holds the potential to redefine clinical research practices."
"The integration of AI, customization, and emerging technologies in clinical data review tools is an exciting frontier that holds the potential to redefine clinical research practices."
In summary, keeping abreast of future trends is vital for all stakeholders in clinical research. Understanding how to implement these advanced tools will lead to better processes, stronger compliance, and impressive results in clinical trials.
Epilogue
The conclusion of the article serves as a vital synthesis of the critical points discussed regarding clinical data review tools. Recognizing their significance is essential as they play a foundational role in the accuracy, integrity, and efficiency of clinical research processes. In a landscape increasingly governed by data, these tools empower researchers to enhance the quality of their analyses and ultimately improve patient outcomes.
Summarizing Key Insights
In summarizing the key insights from this exploration, it is clear that clinical data review tools vary extensively in their functionalities and applications. Tools such as statistical analysis software, data visualization platforms, and electronic data capture systems each contribute uniquely to the research process. The integration of these tools leads to effective data cleaning, validation, and seamless monitoring, which are critical to adhering to regulatory standards. Moreover, understanding the specific challenges, such as data standardization and user training, enhances user preparedness.
Moreover, the regulatory landscape governing clinical research cannot be overlooked. Compliance with good clinical practice (GCP) guidelines and data privacy regulations like GDPR and HIPAA remains paramount for any clinical research activity. Failure to comply can lead to severe consequences, making it even more important to implement best practices and keep abreast of future trends.
"The future of clinical data review tools will be shaped by advancements in artificial intelligence and machine learning, which are anticipated to provide deeper insights and greater customization in user experience."
"The future of clinical data review tools will be shaped by advancements in artificial intelligence and machine learning, which are anticipated to provide deeper insights and greater customization in user experience."
Looking Ahead at Clinical Data Review Tools
Looking forward, the evolution of clinical data review tools promises innovative advancements that are likely to change the research landscape. The integration of artificial intelligence and machine learning into these tools is one significant trend that stands to enhance data analysis, providing faster and more accurate results. Increased customization options will also enable tools to better fit the specific needs of users, improving overall effectiveness.
Furthermore, emerging technologies are expected to redefine data analysis methods. Cloud computing, for example, could facilitate real-time data sharing and enhance collaborative research efforts. As these trends unfold, staying informed will be crucial for professionals in the field. Continuous investment in user training and support will also be essential to leverage the full potential of these tools.