Comprehensive Overview of Online RNA Sequencing
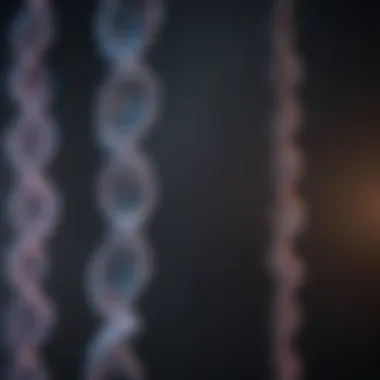
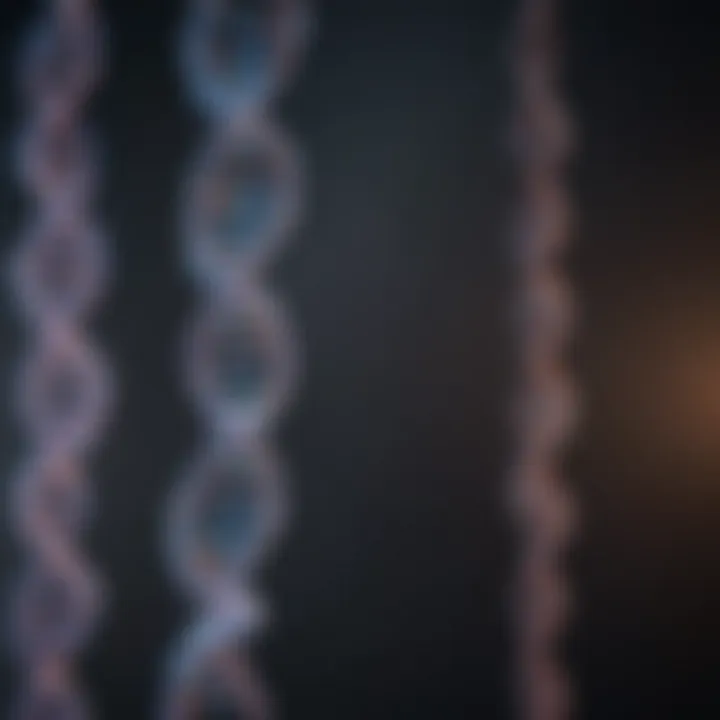
Intro
RNA sequencing analysis has gained prominence as a vital tool in molecular biology. It enables scientists to explore gene expression with remarkable precision. Understanding how RNA sequencing works is crucial for both beginners and seasoned researchers. This section delves into the foundational aspects of RNA sequencing, shedding light on its significance and applications in research.
Key Concepts
Definition of the Main Idea
RNA sequencing, often abbreviated as RNA-seq, is a technique that allows researchers to examine the transcriptome of a cellβbasically capturing all the RNA produced. This method provides insights into gene activity and can reveal how genes are regulated. By converting RNA into complementary DNA (cDNA) and sequencing it, scientists can quantify gene expression levels and identify new transcripts.
Overview of Scientific Principles
The basic principle behind RNA sequencing is that RNA molecules are transcribed from DNA and serve as templates for protein synthesis. During RNA-seq, RNA is extracted from the sample of interest, then subjected to reverse transcription to generate cDNA. This cDNA is subsequently sequenced through different platforms like Illumina or Oxford Nanopore Technologies. The resulting sequence data is analyzed to generate expression profiles, helping to elucidate the biological roles of genes.
Current Research Trends
Recent Studies and Findings
Recent advancements in RNA sequencing technology have led to more sensitive and accurate measurements of gene expression. For instance, studies have focused on single-cell RNA sequencing. This method allows for the analysis of individual cells, revealing heterogeneity in gene expression that bulk RNA sequencing might miss. New algorithms are continually being developed to enhance data analysis, improving the ability to interpret complex datasets.
Significant Breakthroughs in the Field
Significant breakthroughs include the adoption of long-read sequencing technologies, which provide greater context to RNA sequences by capturing longer contiguous sequences. This advancement has been crucial in identifying splice variants and understanding gene regulation more comprehensively. Furthermore, the integration of RNA-seq with other omics approaches has opened up avenues for a more holistic understanding of cellular functions.
RNA sequencing not only allows the assessment of gene expression but also helps in discovering novel RNAs, crucial for understanding gene regulation.
RNA sequencing not only allows the assessment of gene expression but also helps in discovering novel RNAs, crucial for understanding gene regulation.
Prelude to RNA Sequencing
The exploration of RNA sequencing has grown exponentially in significance within molecular biology. This introductory section serves as a foundational layer for understanding RNA sequencing and its essential role in contemporary genomic research. RNA sequencing, often abbreviated as RNA-seq, has transformed how researchers examine gene expression and comprehend the intricate biological networks within cells.
As an evolving technology, RNA-seq provides a detailed view of the transcriptome, allowing scientists to observe which genes are active at any given time. This understanding is paramount in various research domains, including developmental biology, cancer research, and neurobiology. During this section, we will cover key concepts that form the backbone of RNA sequencing, its historical development, and its current relevance in genomic studies.
Definition of RNA Sequencing
RNA sequencing is a cutting-edge technique used to analyze the quantity of RNA in a sample. Essentially, it captures the complete set of transcripts expressed by the genome at a specific time under defined conditions. By sequencing RNA, researchers can obtain insights into gene expression levels, identify novel transcripts, and determine alternative splicing events. This method provides a high-resolution view of the transcriptomic landscape, which is essential for understanding complex biological processes.
Historical Context and Development
The evolution of RNA sequencing has roots in earlier technological advancements in genomics. The initial techniques involved Sanger sequencing, which was primarily used for DNA analysis. However, with the advent of next-generation sequencing (NGS) technologies in the mid-2000s, RNA-seq emerged as a practical and powerful method. NGS allowed for massively parallel sequencing, enabling researchers to analyze millions of RNA fragments simultaneously. The simplicity and efficiency of RNA-seq have led to its rapid acceptance and widespread utilization across various fields of biology.
Significance in Genomic Research
Understanding the transcriptome is a vital aspect of genomic research. RNA sequencing has revolutionized the study of gene expression, providing significant advantages over traditional methods like microarrays. Here are some key benefits that RNA-seq brings to genomic research:
- Comprehensive Data: RNA-seq provides a more complete picture of gene expression, detecting low-abundance transcripts that microarrays might miss.
- Quantitative Outcomes: It offers quantitative analysis of gene expression levels, enabling detailed comparisons across different conditions or treatments.
- Novel Discoveries: RNA-seq facilitates the discovery of new transcripts and splicing variants, enhancing our understanding of gene regulation.
The importance of RNA sequencing cannot be overstated, as it has become indispensable for unraveling the complexities of gene function in health and disease.
The importance of RNA sequencing cannot be overstated, as it has become indispensable for unraveling the complexities of gene function in health and disease.
As a result of these advantages, RNA sequencing is regarded as a vital tool for both basic and applied research in molecular biology, accelerating progress in fields such as personalized medicine, developmental biology, and therapeutic interventions.
The Workflow of RNA Sequencing
The workflow of RNA sequencing is integral to obtaining accurate and meaningful results in genomics. This process includes multiple stages that ensure the integrity of the data from biological sample to final analysis. Understanding this workflow is essential for researchers and practitioners in the field, as each step influences the outcome and interpretation of the sequencing results. The primary considerations in RNA sequencing workflow include sample preparation, sequencing technologies, and data acquisition, all of which play crucial roles in the success of RNA-seq experiments.
Sample Preparation
Sample preparation is a foundational step in the RNA sequencing workflow, and it dictates the quality of the data produced. This phase involves several critical processes that ensure the RNA extracted is suitable for downstream applications.
RNA Extraction
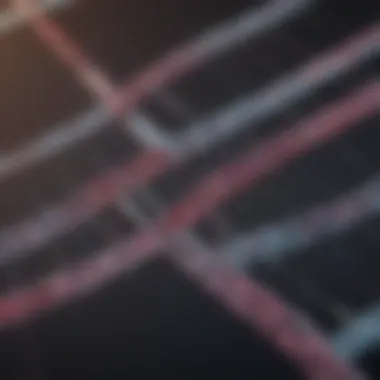
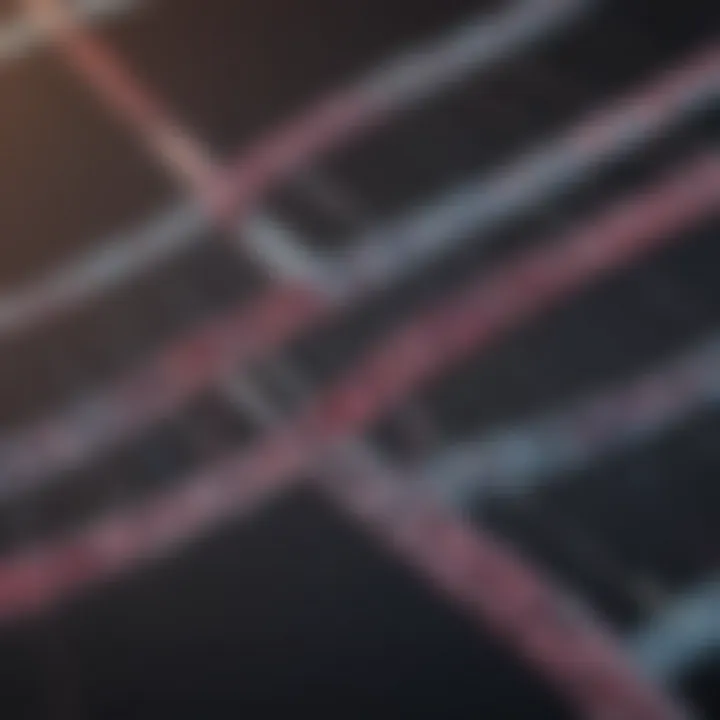
RNA extraction is the process of isolating RNA from biological samples. This step is vital as high-quality RNA is needed for effective sequencing. Methods for RNA extraction include organic solvent extraction and silica-based methods. Silica-based extraction has gained prevalence because it yields cleaner samples and minimizes contamination. Its affinity for RNA is a key characteristic that makes it a popular choice for laboratories. The unique feature of silica-based extraction is its ability to selectively bind RNA, thus allowing for the effective removal of proteins and other contaminants. However, it may require careful handling to prevent RNA degradation.
Quality Control Measures
Quality control measures are essential in the RNA sequencing workflow to evaluate the integrity and concentration of RNA samples. These measures often involve electrophoresis and spectrophotometric analysis to assess the RNA's purity. The key characteristic of quality control is its role in guaranteeing that only suitable RNA samples proceed to sequencing. This focus on quality helps mitigate risks associated with low-quality data. The unique aspect of these measures is that they provide immediate feedback on sample quality, which can save time and resources in the long run. Nevertheless, they may add additional steps to the workflow, which could delay processing times.
Library Preparation
Library preparation is the next vital step after quality control. This process involves converting RNA into a format that can be sequenced. It requires the generation of cDNA from RNA, followed by the fragmentation and addition of sequencing adapters. A significant aspect of library preparation is that it determines how effectively the RNA will be sequenced. This stepβs characteristic is its complexity, which often requires specific reagents and protocols tailored to the samples being used. The unique feature of library preparation is its precision in managing the size and diversity of the cDNA fragments, which ultimately influences the depth of coverage in sequencing. However, meticulous attention is needed during this process, as poor library preparation can lead to biased data.
Sequencing Technologies
Sequencing technologies represent the means through which RNA libraries are transformed into usable sequence data. Each technology has its strengths and weaknesses, affecting both cost and data accuracy.
Sanger Sequencing
Sanger sequencing is a method known for its high accuracy in sequencing small segments of DNA or RNA. It is particularly effective when precise sequences are essential, often used in validating results obtained from next-generation sequencing. The key characteristic of Sanger sequencing is its ability to produce highly reliable data with relatively low error rates. This makes it a trusted choice in the verification stage of RNA sequencing. The unique feature is its capacity for reading longer DNA fragments compared to newer technologies. However, its throughput is lower and more expensive relative to next-generation sequencing.
Next-Generation Sequencing
Next-generation sequencing (NGS) has revolutionized how sequencing is conducted, allowing for massively parallel processing of RNA samples. NGS can sequence millions of fragments simultaneously which lowers the cost per base of sequencing. The major characteristic of NGS is its scale, leading to high-throughput capabilities. This technology is a beneficial of choice for large-scale studies due to its efficiency and cost-effectiveness. The unique feature of NGS is the depth of data it can generate quickly. However, challenges remain, especially regarding the complexity of data analysis and the potential for sequencing biases.
Third-Generation Sequencing
Third-generation sequencing refers to newer technologies that directly sequence RNA molecules in real-time, offering the ability to work with longer reads. This aspect allows for a more comprehensive insight into RNA structure and variants, providing a fuller picture of the transcriptome. The key characteristic of third-generation sequencing is its capability to generate long reads that span entire transcripts. This makes it a highly beneficial option for applications involving isoform detection. The unique feature of this technology is its real-time data generation, which can significantly shorten turnaround time for results. However, it can be more expensive and may still be in developmental stages for broader applications.
Data Acquisition
Data acquisition represents the final stage in the workflow of RNA sequencing, where the sequenced data is compiled for further analysis. During this phase, proper methodologies for data capture ensure that the information is organized effectively for downstream analysis.
Efficient data acquisition is vital in maintaining the integrity of large datasets. The increasing amount of data generated continuously necessitates robust systems for storage and management. This step prepares the groundwork for subsequent data analysis and interpretation.
Data Analysis Techniques in RNA-Sequencing
Data analysis is a crucial phase in RNA sequencing. It transforms raw sequencing data into meaningful biological insights. This segment will emphasize various methods employed to analyze RNA-seq data, focusing on their significance, functionality, and relevance in understanding gene expression.
Processing Raw Sequence Data
Processing raw sequence data involves several steps to clean and prepare the data for further analyses. These steps enhance the reliability and accuracy of the results obtained from RNA sequencing.
Trimming and Filtering
Trimming and filtering are two primary tasks in processing raw RNA-seq data. This process involves removing low-quality sequences and artifacts that might compromise outcomes. The key characteristic of trimming is its ability to manage sequencing errors, which is a common issue in high-throughput sequencing. This technique ensures that only high-quality sequences are analyzed.
Trimming and filtering are popular choices because they directly improve data quality. A unique feature of these processes is the ability to apply specific quality control metrics to evaluate sequence integrity. Advantages include reducing noise and enhancing the signal from genuine biological variants. However, a disadvantage may arise if overly aggressive trimming leads to the loss of potentially valuable data.
Alignment to Reference Genomes
Alignment to reference genomes refers to the procedure where processed sequences are matched against a known genome. This method is essential for identifying the origins and functions of gene transcripts. Its key characteristic is that it allows for the identification of gene expression patterns, linking back to particular genes in the reference genome. This is a beneficial choice for researchers aiming to interpret complex genomic information accurately.
A unique feature of alignment procedures is their capacity to handle multi-mapping reads, which occur when a sequence can align to multiple positions. This can be advantageous in studies of gene regulation but may complicate interpretations. One must also be cautious of alignment bias. If the reference genome is not closely matched to the sample organism, the results may misrepresent the actual expression levels.
Quantification of Gene Expression
Quantification of gene expression is a fundamental part of RNA sequencing analysis, providing insights into the variability and dynamics of gene activity under different conditions or treatments. This section will cover counting reads and normalization methods, both critical for deriving meaningful conclusions from sequencing data.
Counting Reads
Counting reads involves determining how many times a particular transcript is detected across the sequences. This analysis is critical because it translates sequencing depth into quantifiable gene expression levels. The primary characteristic of counting reads is its straightforward approach to measure expression levels directly from the raw data.
This method is a beneficial choice because it provides a clear representation of gene activity. A unique feature is its ability to facilitate comparisons across multiple conditions or time points, making it valuable for time-series experiments. Nonetheless, a potential drawback might be the assumptions made about read distribution, leading to potential biases in expression estimates.
Normalization Methods
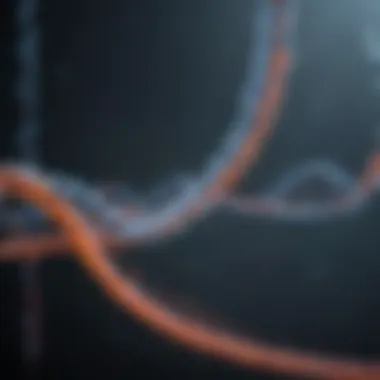
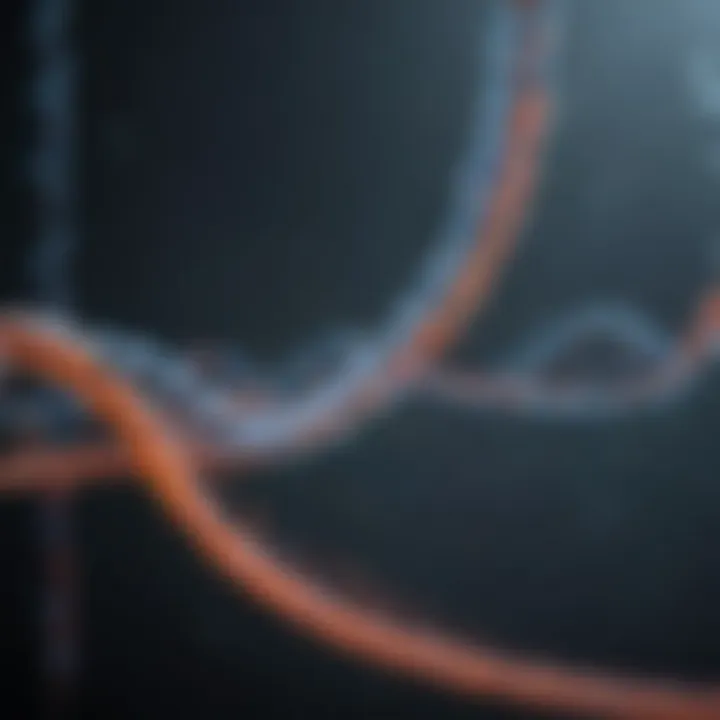
Normalization methods are techniques used to adjust the gene expression data from various samples. This process addresses biases related to sequencing depth and other variables. The key characteristic of normalization is its capability to make data from different conditions comparable, enabling fair analysis.
Normalization methods are essential for achieving accurate results, especially in experiments with multiple samples. A unique feature of these methods is their various approaches, such as TPM (transcripts per million) and RPKM (reads per kilobase of transcript per million mapped reads), each offering specific advantages. However, they can also introduce their own complexities, as incorrect normalization can lead to misleading interpretations of expression levels.
Statistical Analysis Methods
Statistical analysis methods play an essential role in interpreting RNA sequencing data. Through rigorous analysis, researchers can derive insights into the significance of gene expression changes between different conditions or phenotypes.
Differential Expression Analysis
Differential expression analysis identifies genes that exhibit significant changes in expression levels between two or more conditions. This process is critical for understanding the biological implications of an experimental treatment or condition. Its key characteristic lies in its ability to generate lists of differentially expressed genes that can be further analyzed for biological relevance.
This method is a popular choice due to its straightforward approach and the strong foundation of statistical models used, such as the negative binomial distribution. A unique feature is its emphasis on false discovery rate correction to minimize false positives. However, the complexity arising from low-count genes can pose a challenge, as they might lead to unreliable results due to random variation.
Machine Learning Approaches
Machine learning approaches are increasingly applied to analyze RNA-seq data. They leverage algorithms to recognize patterns and predict outcomes from complex datasets. The key characteristic of these approaches is their ability to process large volumes of data, providing robust analysis without assuming specific distribution patterns.
Machine learning methods have become a beneficial choice in discovering hidden relationships in gene expression data. A unique feature is their adaptability, allowing for iterative refinement of models as new data becomes available. Despite their advantages, reliance on machine learning requires meticulous validation to avoid overfitting and ensure that findings are biologically meaningful.
Data analysis techniques in RNA-sequencing are essential not only for obtaining insights into gene expression but also for paving the way for future discoveries in molecular biology, influencing fields such as genomics, biomedicine, and therapeutic development.
Data analysis techniques in RNA-sequencing are essential not only for obtaining insights into gene expression but also for paving the way for future discoveries in molecular biology, influencing fields such as genomics, biomedicine, and therapeutic development.
Common Software and Tools for RNA-seq Analysis
The field of RNA sequencing analysis is heavily reliant on software and tools that facilitate data interpretation and result generation. The use of the right tools can significantly affect the outcome of a study, impacting accuracy and efficiency. As researchers delve into RNA-seq data, understanding these software solutions is crucial to their success. They not only help in processing vast amounts of data but also enable insightful analysis of gene expression levels. Thus, familiarity with these tools is beneficial for anyone engaged in molecular biology.
Overview of Popular Tools
STAR
STAR stands out as a highly efficient aligner specifically designed for RNA sequencing data. Its ability to handle large datasets is essential for modern research, making it very popular in the community. The key characteristic of STAR is its speed, which enables alignment of reads to a reference genome quickly. This efficiency is especially crucial when dealing with extensive datasets, where the time for processing can be a bottleneck.
One unique feature of STAR is its capability to align reads across splice junctions, which is vital for accurate RNA-seq analysis, as many genes are transcribed in ways that involve splicing. However, STAR does require substantial computational resources, which might be a limitation for smaller labs without access to high-performance computing.
DESeq2
DESeq2 is another critical tool that focuses on differential gene expression analysis. Its primary feature lies in statistical algorithms that normalize gene counts, allowing accurate comparison across conditions or groups. This software is considered a standard in RNA-seq analysis due to its robustness in assessing significance of detected changes in gene expression.
A unique aspect of DESeq2 is its use of the negative binomial distribution to model count data, which makes it suitable for datasets with overdispersion. This capability helps in managing variability in biological replicates. Despite its strengths, DESeq2 can be complex for beginners, requiring some knowledge of R and statistical principles for effective application.
EdgeR
EdgeR focuses on the analysis of digital gene expression data and is similar to DESeq2 in its statistical approach. It is designed to detect differentially expressed genes from RNA-seq data, using methods that account for biological variability. A key feature of EdgeR is its ability to work with complex experimental designs, including those with multiple conditions.
What sets EdgeR apart is its capability to perform multiple testing corrections, which enhances the reliability of results. However, like DESeq2, familiarity with R programming is necessary to fully utilize EdgeR's potential, which can present a learning curve.
Web-Based Analysis Platforms
Galaxy
Galaxy provides a user-friendly web-based platform for RNA-seq analysis, which appeals to users who prefer graphical interfaces over coding. Its significance lies in making complex bioinformatics analyses accessible to a broader audience, including those without programming skills. Users can create workflows visually, making it easier to replicate and share analyses.
One unique feature of Galaxy is its comprehensive collection of tools, which allows users to perform various analyses ranging from data management to visualization without needing extensive technical knowledge. Nevertheless, reliance on internet connectivity and server speed can hinder performance during intense computational tasks.
Cufflinks
Cufflinks is notable for its ability to assemble transcripts and estimate their abundances from RNA-seq data. Its main contribution is in transcriptome reconstruction, especially in scenarios with novel transcript discovery. Cufflinks offers significant benefits, allowing identification of alternative splicing events and isoform expression.
A unique feature of Cufflinks is its package, which allows for visual inspection of transcript expression. However, it usually requires a prior alignment step, which can complicate the process for some users.
RNA-Sequencing Analysis Contraptions
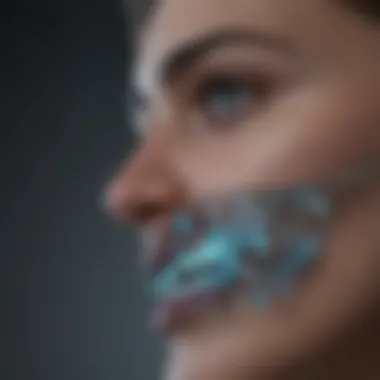
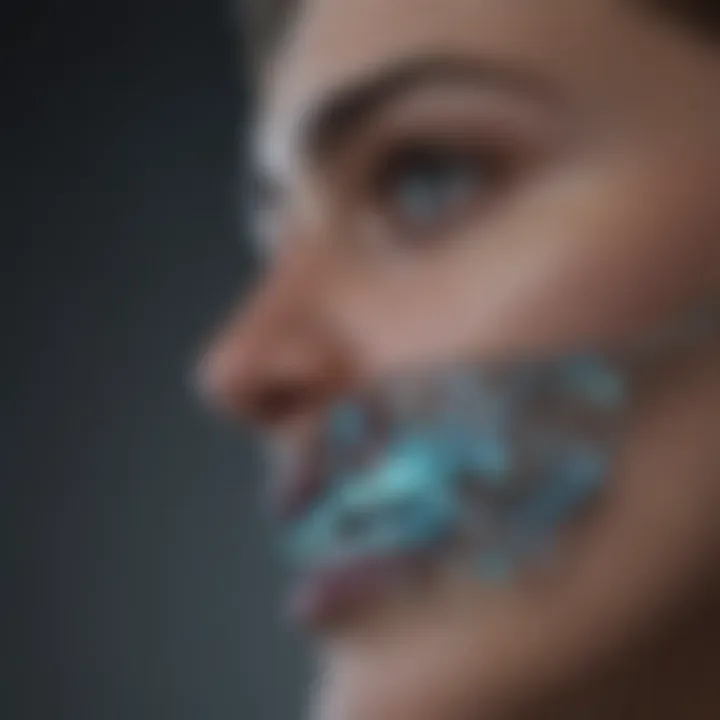
RNA-sequencing analysis contraptions encompass various integrated tools that streamline workflows for RNA-seq data analysis. These tools are designed to simplify the analysis pipeline, handling steps from quality control to differential expression analyses seamlessly. The versatility and modeling capabilities of these contraptions make them valuable in research settings.
One of their key benefits is providing a comprehensive environment for users to manage their analysis tasks without switching between different software. However, the complexity of some contraptions may overwhelm novice users.
Comparison of Tool Usability
When comparing the usability of RNA-seq tools, several factors come into play: user interface design, accessibility, required knowledge base, and the extent of built-in help features. While tools like Galaxy are highly user-friendly, others like DESeq2 and EdgeR require more expertise. Choosing the right tool depends on the userβs specific needs and their readiness to learn. Each software solution has its unique strengths and weaknesses, making it crucial to evaluate them based on project requirements and individual skill levels.
Challenges and Limitations in RNA Sequencing Analysis
Exploring the challenges and limitations in RNA sequencing analysis is crucial for researchers aiming to interpret their data accurately. A robust understanding of these obstacles not only improves the reliability of results but also enhances the overall validity of conclusions drawn from RNA-seq studies. Addressing these issues can lead to more effective experimental designs and better interpretation of biological phenomena.
Bias in RNA-seq Data
Bias in RNA-seq data can arise from various sources, including library preparation, sequencing technologies, and biological variability. One significant concern is the bias introduced during the process of RNA extraction and library preparation. Certain RNA species, particularly low-abundance transcripts, may be underrepresented in the final sequence data. This can skew the overall expression profiles, leading researchers to miss critical insights about gene regulation.
Moreover, the selection of sequencing methods can also impact data quality. Next-generation sequencing technologies like Illumina may produce quantity, while platforms like PacBio could offer more detailed sequence information but at a lower throughput. These trade-offs create an intrinsic bias that can complicate comparisons across studies and necessitates careful selection of methodologies suited for the research objectives.
Finally, biological variation inherent to the samples can further contribute to data bias. Individual differences in gene expression profiles, influenced by factors such as environment and development stage, can mask the true biological signals if not accounted for properly.
Interpreting Complex Biological Data
RNA sequencing generates massive amounts of data, making it challenging to correlate these results with biological functions. The complexity of this data can provoke difficulties in interpreting true biological relevance. Often, researchers must distinguish between noise and meaningful signals, which can be a daunting task.
Data interpretation often relies on computational algorithms and statistical models. However, the choice of tools and methods can lead to disparate outcomes. For instance, methods for quantifying gene expression can vary greatly, affecting downstream analyses like differential expression. Incorrect interpretation of data can result in misleading conclusions about gene functions or regulatory networks. Thus, a thorough understanding of the limitations of analytical methods is essential.
Furthermore, the biological context must be carefully considered. Not all differentially expressed genes have functional implications, making it crucial to integrate additional biological knowledge with sequencing data for viable interpretations.
Issues with Reproducibility
Reproducibility in RNA sequencing studies is a widespread concern and represents a significant limitation. The variability stemming from different biopsies, batch effects, and even slight methodological differences can create inconsistencies in results. When attempts to replicate results yield different outcomes, it calls into question the reliability of the conclusions drawn from these studies.
To enhance reproducibility, standardization of protocols is vital. It is essential to minimize variables in sample handling and library preparation, as well as using consistent data analysis techniques. Additionally, sharing raw data and methodologies openly can improve transparency and facilitate collaboration, allowing researchers to verify findings.
In summary, recognizing and addressing the challenges and limitations in RNA sequencing analysis is essential for advancing scientific research. Continual improvements in methods and analytical techniques are needed to mitigate these issues and to foster a more reliable interpretation of gene expression data.
Future Directions in RNA Sequencing
With the rapid advancement of genomic technologies, the future of RNA sequencing holds significant promise. The exploration of RNA sequencing methodologies continues to evolve, which greatly influences the landscape of molecular biology. Understanding these future directions is essential for researchers, educators, and students who wish to stay informed about breakthroughs that could lead to novel insights into gene expression and biological processes.
Integration with Other Omics Technologies
The integration of RNA sequencing with other omics technologies is crucial. Combining RNA sequencing with proteomics and metabolomics allows a more holistic view of cellular functions. This multi-omics approach can enhance the understanding of various biological systems. By examining RNA, proteins, and metabolites simultaneously, researchers can identify correlations that are fundamental in understanding disease mechanisms.
Key benefits include:
- Comprehensive Data Analysis: This integration helps create a more complete picture of cellular activities, revealing underlying biochemical pathways.
- Enhanced Biomarker Discovery: The identification of novel biomarkers is more achievable when data across multiple layers of biological information is considered together.
- Improved Therapeutic Strategies: Insights from integrated data can lead to more effective treatments tailored to specific biological contexts.
RNA-sequencing in Clinical Applications
The application of RNA sequencing in clinical settings is growing steadily. As precision medicine becomes more prominent, RNA sequencing offers valuable insights into patient-specific gene expression profiles. It enables the stratification of patients based on their molecular signatures, which can inform targeted therapies and improve treatment outcomes.
Significant clinical applications include:
- Cancer Diagnostics: RNA sequencing assists in profiling tumors, revealing mutations and gene expression changes correlating with prognosis.
- Infectious Diseases: The technology aids in understanding host-pathogen interactions and provides insights necessary for designing effective vaccines and therapeutics.
- Rare Diseases: RNA sequencing can uncover novel mutations in patients with rare genetic disorders, ultimately guiding diagnosis and management.
Emerging Technologies in RNA Sequencing
Emerging technologies are set to further revolutionize RNA sequencing. Innovations continue to enhance speed, accuracy, and cost-effectiveness. Single-cell RNA sequencing is a significant advancement, allowing researchers to investigate gene expression at the individual cell level. This technology unveils the heterogeneity within populations of cells, revealing insights that bulk RNA-seq cannot provide.
Other promising advancements include:
- Long-read Sequencing: Technologies like Pacific Biosciences and Oxford Nanopore are making it possible to obtain longer sequences of RNA, which improve genome assembly and transcriptome analysis.
- Real-Time Sequencing: Real-time solutions offer dynamic insights into transcriptional changes as they occur, greatly benefiting time-sensitive studies.
- Portable Sequencing Devices: The advent of portable sequencers can facilitate rapid and cost-effective analysis in numerous settings, from clinics to remote research locations.
"The advancements in RNA sequencing technologies are reshaping our understanding of biological processes and have wide-ranging implications for health and disease."
"The advancements in RNA sequencing technologies are reshaping our understanding of biological processes and have wide-ranging implications for health and disease."
In summary, the future of RNA sequencing is bright and offers an array of possibilities for advancing scientific research and clinical applications. The integration of multi-omics approaches, clinical relevance, and emerging technologies will undoubtedly shape this field, enhancing our comprehension of complex biological phenomena.
For further reading on RNA sequencing and its implications, visit Wikipedia or Britannica.