NDVI Crop Monitoring: Techniques and Insights
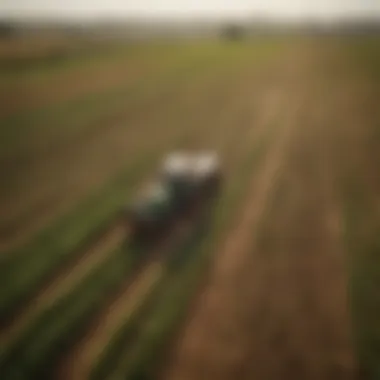
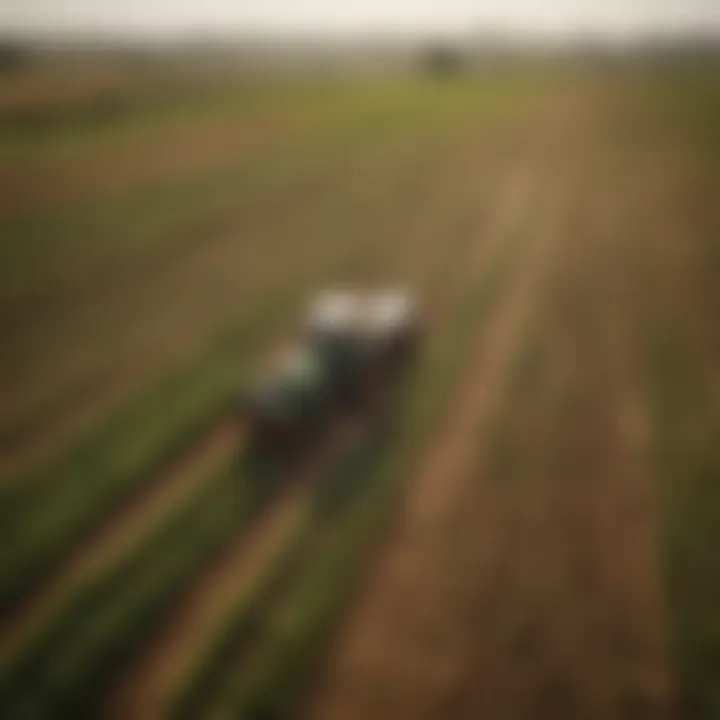
Intro
The Normalized Difference Vegetation Index (NDVI) has emerged as a pivotal tool in precision agriculture. By enabling farmers and researchers to monitor crop health from a distance, NDVI facilitates informed decision-making in agronomy. This article provides a technical overview of NDVI, exploring its principles, methodologies, tools, and case studies while emphasizing its significance in enhancing agricultural sustainability.
Key Concepts
Definition of the Main Idea
NDVI is an index derived from remote sensing data, specifically using bands of near-infrared and red light to assess vegetation health. By calculating the ratio of reflected light in these bands, NDVI allows for the identification of vegetation density and vigor. Its values range from -1 to +1, where higher values indicate healthier vegetation.
Overview of Scientific Principles
NDVI is grounded in the principles of reflectance. Healthy crops absorb most of the visible light for photosynthesis while reflecting a significant portion of near-infrared light. The NDVI formula is as follows:
In this equation, NIR refers to near-infrared reflectance, and Red represents red reflectance. The application of this index enables the quantification of various parameters such as chlorophyll content, plant stress, and overall health.
Current Research Trends
Recent Studies and Findings
Recent studies have focused on enhancing the accuracy of NDVI readings through various technological advances. For example, the integration of drones equipped with multispectral cameras allows for more detailed and localized monitoring of crop conditions. Research indicates that this approach is particularly effective in identifying areas of crop stress before visible signs appear.
Significant Breakthroughs in the Field
Innovations in machine learning and artificial intelligence have also played a crucial role in optimizing NDVI data analysis. These technologies can analyze large datasets quickly, identifying patterns and anomalies that human operators might miss. As a result, farmers can respond more rapidly to emerging agricultural challenges, reducing loss and increasing efficiency.
"The use of NDVI in conjunction with advanced analytics can potentially revolutionize farming practices, enabling a proactive approach to crop management."
"The use of NDVI in conjunction with advanced analytics can potentially revolutionize farming practices, enabling a proactive approach to crop management."
Through its multidimensional applications, NDVI is reinforcing the framework of precision agriculture. As the technology develops, its inclusion in agricultural practices is likely to become more prevalent, driving efforts towards sustainable farming.
Finale
Preamble to NDVI
The Normalized Difference Vegetation Index (NDVI) is a critical tool in modern agriculture, particularly in the domains of crop monitoring and precision farming. Its significance lies in its ability to provide insights into vegetation health, growth potential, and overall agricultural productivity. Understanding NDVI involves grasping its definition, historical context, and applications. This knowledge is essential for those who seek to optimize farming practices for increased efficiency and sustainability.
Definition of NDVI
NDVI is a remote sensing index that measures the difference between near-infrared (NIR) and red light reflected by vegetation. The index is calculated using the following formula:
A higher NDVI value, ranging from -1 to +1, indicates healthier and more vigorous vegetation. Values close to 0 suggest sparse vegetation, while negative values often correspond to non-vegetated surfaces such as water or bare soil.
Historical Context
The application of NDVI began in the late 1970s, coinciding with advances in satellite technology. The use of remote sensing for agricultural monitoring was a novel idea at that time. Researchers such as Rouse et al. were instrumental in developing NDVI, allowing it to become widely utilized in agronomy and environmental studies. Over the years, NDVI has evolved, integrating more complex algorithms and satellite imagery for enhanced accuracy. Its historical development illustrates the shift towards data-driven agriculture, opening avenues for more informed decision-making in farming practices.
The use of NDVI has become standard practice for monitoring crop health and predicting yields, marking a significant advancement in precision agriculture.
The use of NDVI has become standard practice for monitoring crop health and predicting yields, marking a significant advancement in precision agriculture.
As we delve deeper into the subsequent sections of this article, we will explore the technical aspects of NDVI, the scientific principles behind it, and its various applications in agriculture.
Understanding Vegetation Indices
Understanding vegetation indices is crucial for effectively monitoring crop health and optimizing agricultural practices. These indices serve as important tools that help farmers, researchers, and agronomists assess plant conditions over large areas. They rely on remote sensing technologies to gather data that is often inaccessible through traditional methods. This section delves into the meaning of vegetation indices and discusses prominent types used in the field.
What are Vegetation Indices?
Vegetation indices are mathematical combinations of spectral reflectance values, primarily from the red and near-infrared portions of the electromagnetic spectrum. These indices help quantify plant health and vigor by leveraging the differences in how healthy vegetation and stressed plants reflect light. The most notable characteristic of healthy vegetation is the ability to absorb visible light for photosynthesis while reflecting a significant amount of near-infrared light.
The most famous vegetation index is the Normalized Difference Vegetation Index (NDVI). It highlights the ratio of the difference between near-infrared and red reflectance to their sum. The formula looks like this:
[ NDVI = \fracNIR - RedNIR + Red ]
Where:
- NIR represents near-infrared reflectance
- Red represents red reflectance
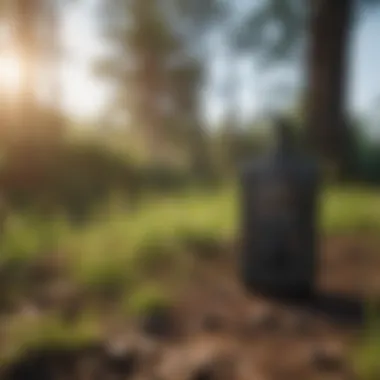
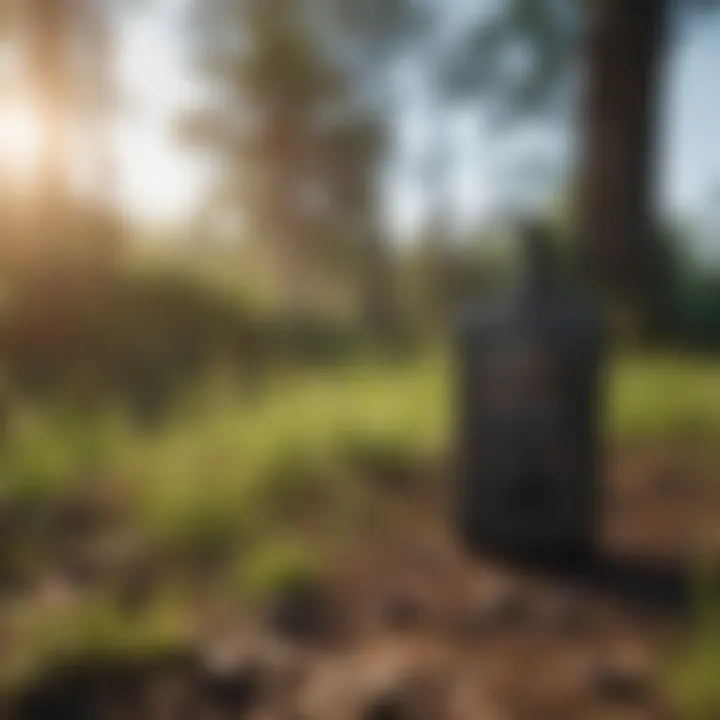
By using NDVI, practitioners can evaluate areas of crop stress, identify potential diseases, and manage irrigation more effectively. Other indices provide distinct insights based on the specific spectral characteristics they assess.
Other Common Vegetation Indices
Besides NDVI, several other vegetation indices are utilized for diverse purposes. Here is a brief overview of some significant ones:
- EVI (Enhanced Vegetation Index): This index enhances sensitivity in high biomass regions and corrects for atmospheric effects. It incorporates blue reflectance to provide improved estimates of vegetation health.
- GNDVI (Green Normalized Difference Vegetation Index): GNDVI uses green reflectance rather than red. It is particularly helpful in assessing chlorophyll content and overall plant vitality.
- SAVI (Soil-Adjusted Vegetation Index): This index accounts for soil brightness and is effective in areas with sparse vegetation. It helps to reduce soil background noise in NDVI assessments.
- MSAVI (Modified Soil-Adjusted Vegetation Index): This is a further refinement of SAVI. It reduces the effect of soil brightness and provides a clearer signal of vegetation health in arid conditions.
These indices complement NDVI by offering distinct perspectives on vegetation characteristics, thus broadening the tools available for precision agriculture practices. Understanding these indices allows for more tailored interventions based on the unique needs of crops in different environmental conditions.
"The ability to accurately assess and manage crop health through various vegetation indices can lead to significant improvements in yield, sustainability, and resource management."
"The ability to accurately assess and manage crop health through various vegetation indices can lead to significant improvements in yield, sustainability, and resource management."
Through the combination of these methods, researchers and agronomists continue to enhance agricultural practices, taking strides towards sustainable farming solutions.
The Science Behind NDVI
The science of NDVI, or Normalized Difference Vegetation Index, is crucial to understanding how it benefits crop monitoring. NDVI provides insight into plant health and vigor. This quantitative method relies on the analysis of spectral reflectance, allowing agricultural professionals and researchers to assess the condition of vegetation effectively. By analyzing the unique spectral signatures of plants, one can identify a variety of factors, such as growth stages and stress levels, quickly and efficiently.
This methodology allows for timely interventions in agricultural practices, ultimately supporting sustainable farming. Understanding the scientific principles behind NDVI can lead to better decision-making in crop management and resource allocation.
Spectral Reflectance
Spectral reflectance directly relates to the different wavelengths of light that foliage reflects. Healthy vegetation tends to reflect more infrared light and absorb more visible light. Hence, by capturing and analyzing these reflectance patterns, researchers can gauge the overall health of crops.
The spectral reflectance curve often exhibits peaks and troughs that correspond to the levels of chlorophyll in plants. Chlorophyll absorbs light in the red part of the spectrum for photosynthesis, whereas healthy leaves reflect infrared light. Therefore, the differences in these reflectance values form the backbone of NDVI calculations.
Calculation of NDVI
Calculating NDVI is a straightforward process based on the principles of spectral reflectance. The formula fundamentally compares the amount of light reflected in the red and near-infrared spectrum. This comparison enables users to derive a numerical value that indicates vegetation health.
Formula for NDVI
The formula for NDVI is:
[ NDVI = \fracNIR - RNIR + R ]
Where:
- NIR = Near-Infrared Reflectance
- R = Red Reflectance
This formula is preferred for several reasons. Its simplicity allows for easy computation across various platforms, from handheld devices to satellites.
Primary characteristic: The ratio nature of the NDVI formula allows for values that range between -1 and 1. It effectively normalizes the input data, making comparisons between different time periods and locations valid.
Unique features: While NDVI is effective, it does have limitations. For instance, values close to zero can indicate sparse vegetation or soil background, complicating assessments. Despite these disadvantages, NDVI remains a beneficial tool for precision agriculture.
Data Sources for NDVI Calculation
The data sources for NDVI calculation play a significant role in its utility. Various platforms, including satellite imagery and drone technology, are commonly used. These data sources are critical because they offer comprehensive coverage and sizable datasets to analyze.
Key characteristic: High-resolution satellite imagery, such as that from Landsat or Sentinel-2, provides the necessary data for accurate NDVI calculations. These sources capture data across specific spectral wavelengths, essential for NDVI accuracy.
Unique features: Each data source has its pros and cons. For example, while satellites provide extensive coverage, they might lack the fine-detail observations that drones can deliver.
The accessibility of different data sources allows for a flexible approach to NDVI monitoring. Utilizing multiple sources can enhance the credibility of analyses, offering a more thorough understanding of crop health.
"NDVI is an instrumental technology that becomes more impactful through the integration of diverse data sources."
"NDVI is an instrumental technology that becomes more impactful through the integration of diverse data sources."
A broad perspective on data and its application results in improved agricultural practices, reflecting NDVI's importance in modern agriculture.
Applications of NDVI in Agriculture
The applications of the Normalized Difference Vegetation Index (NDVI) in agriculture are vast and varied, providing essential insights into crop management. Monitoring crop health, predicting yields, and managing irrigation are three core areas where NDVI plays a critical role. Understanding how NDVI applies in these contexts can lead to more informed decisions and improved agricultural outcomes.
Crop Health Assessment
Crop health assessment using NDVI is foundational in precision agriculture. NDVI provides insights into the vegetation's physiological status by measuring its greenness. High NDVI values typically indicate healthy plants with adequate chlorophyll, while low values may signal stress or disease. Therefore, farmers can early detect problems by regularly monitoring NDVI values.
For effective crop management, NDVI data can be visualized in colored maps. These maps help farmers identify areas needing attention. A well-timed aerial analysis delivers current data. Moreover, NDVI contributes to understanding how crops respond to nutrients and water intake. This insight aids in optimizing fertilizer application and preventing wastage, ultimately impacting overall crop yield.
Yield Prediction
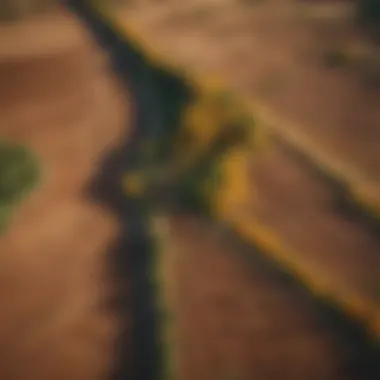
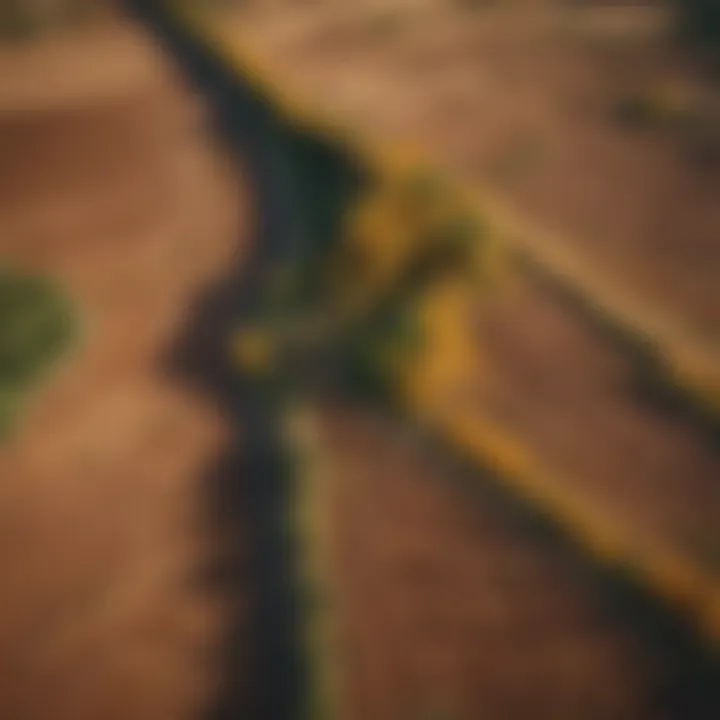
Yield prediction is another significant application of NDVI in agriculture. By analyzing NDVI data throughout the growing season, farmers can establish relationships between NDVI measures and final yields. Higher NDVI readings correlate with better yields, serving as an indicator for potential harvest amounts.
Farmers can employ NDVI values to adjust their expectations based on real-time conditions. For instance, if NDVI starts to decline mid-season, it can indicate impending challenges that may reduce yields. This early warning allows for timely interventions, such as additional fertilization or pest control. Through yield prediction, agricultural planning can be more strategic, reducing risks associated with crop failure.
Irrigation Management
NDVI also enhances irrigation management. Water stress drastically affects plant health. By analyzing NDVI patterns, farmers can assess water needs more accurately. NDVI provides insights into whether plants are receiving sufficient water or if additional irrigation is necessary. This precise understanding facilitates optimal water usage, transforming irrigation practices.
In regions where water supply is limited, NDVI allows for better allocation of resources. By targeting areas demonstrating low NDVI values for irrigation, farmers can prioritize efforts where they are most needed. This method not only preserves water but also supports the sustainability of farming practices.
"The integration of NDVI data into agricultural practices provides significant advantages in monitoring, prediction, and resource management."
"The integration of NDVI data into agricultural practices provides significant advantages in monitoring, prediction, and resource management."
In summary, the applications of NDVI in agriculture are multifaceted. They enable precise health assessments, yield predictions, and efficient irrigation strategies. By leveraging NDVI, farmers can maximize productivity while promoting sustainability in their practices.
Technologies and Tools for NDVI Monitoring
Technologies and tools for NDVI monitoring are central to the effectiveness of this remote sensing technique in agriculture. The evolution of these tools has facilitated the collection of large-scale, precise data which informs decision-making processes. By employing advanced technologies, farmers and researchers can obtain timely insights into crop health, optimizing resource allocation and enhancing yield potential. This section discusses the primary technologies involved in NDVI monitoring, particularly focusing on satellite imagery and drones.
Satellite Imagery
Satellite imagery plays a significant role in NDVI monitoring, providing broad coverage and repetitive data collection capabilities. Satellites equipped with specialized sensors capture images that measure the reflectance of different wavelengths of light. This data is then processed to generate NDVI maps, which display variations in vegetation health across large agricultural areas.
One of the key advantages of satellite imagery is its ability to cover extensive regions within a short timeframe. For instance, satellites like Landsat and Sentinel-2 offer high-resolution images and revisits every few days. This allows for frequent monitoring, which is crucial for understanding crop health changes over time.
Moreover, the availability of freely accessible satellite data significantly lowers the barriers to entry for farmers and researchers. Platforms such as Google Earth Engine facilitate easy access to vast datasets, enabling users to conduct analyses without requiring substantial financial resources. However, it is essential to consider some limitations. Cloud cover can obscure imagery, particularly in tropical regions, reducing the effectiveness of data collection during certain seasons.
In summary, satellite imagery serves as a vital tool for NDVI monitoring, offering broad spatial coverage and cost-effective access to data. Continuous advancements in satellite technology promise further improvements in data quality and availability.
In summary, satellite imagery serves as a vital tool for NDVI monitoring, offering broad spatial coverage and cost-effective access to data. Continuous advancements in satellite technology promise further improvements in data quality and availability.
Drones and UAVs
Drones or Unmanned Aerial Vehicles (UAVs) have gained popularity in recent years for NDVI monitoring. They provide a complementary approach to traditional satellite imaging by offering higher resolution images at a lower altitude. While satellite data can capture large areas, drones excel in providing detailed information about specific fields or crops, allowing for more localized analysis.
The flexibility of drones enables users to select optimal flight paths and altitudes for data collection. This means farmers can monitor specific crops when needed and focus on areas that may show signs of stress or disease. Several drones are equipped with multispectral cameras, which capture the necessary spectral bands to calculate NDVI accurately.
The advantages of using drones include reduced data acquisition costs and increased temporal resolution. Drones can quickly collect data several times throughout the growth cycle, allowing for timely interventions.
Nonetheless, drone usage also requires considerable expertise in operating and data processing, which may present a challenge for some users. Regulatory considerations regarding airspace and usage can also complicate drone deployment.
The integration of drone and satellite technologies presents a comprehensive framework for effective NDVI monitoring, leading to better crop management and increased sustainability.
Case Studies of NDVI in Action
In this section, we will explore case studies showcasing the effectiveness of NDVI in various agricultural scenarios. The insights gathered from these studies are critical in understanding the practical applications and potential challenges associated with NDVI crop monitoring. By observing real-world implementations, both benefits and limitations of NDVI can be appreciated, enhancing its relevance in the field of precision agriculture.
Field Studies
Field studies provide empirical evidence on the utility of NDVI in evaluating crop health and performance. They often entail monitoring specific plots over a growing season, capturing NDVI values at intervals to track how vegetation responds to environmental changes or management practices. For instance, researchers at the University of Nebraska conducted a study on corn and soybean fields using NDVI measurements to assess how irrigation timing affected crop vigor.
The findings from this research indicated a strong correlation between NDVI values and crop yield. Higher NDVI readings were associated with optimal irrigation schedules, demonstrating that NDVI can effectively identify areas requiring immediate attention. This illustrates how NDVI supports decision-making in water management strategies.
Key Considerations in Field Studies:
- Data Collection Frequency: Frequent observations provide a better understanding of crop development.
- Local Conditions: Soil type and weather patterns affect NDVI readings; thus, localized interpretations are necessary.
- Management Practice Impacts: Understanding how specific practices influence NDVI can guide future agricultural strategies.
Comparative Analyses
Comparative analyses utilize NDVI alongside other assessment methods to validate findings. For example, a comparative study may involve using NDVI together with leaf area index (LAI) measurements in vineyards to evaluate grapevine health. By contrasting results from different indicators, researchers can draw more accurate conclusions about crop conditions.
An example is a research project conducted in Chile's wine-producing regions, where NDVI was compared with traditional ground surveys. Results indicated that NDVI not only provided quicker assessments across a larger area but also correlated well with yield estimates, outperforming some conventional methods.
This type of analysis underscores how NDVI complements existing methodologies while enhancing overall efficiency in crop monitoring.
Benefits of Comparative Analyses:
- Enhanced Accuracy: Combining NDVI with other methods reduces error margins.
- Broader Insights: Multiple data sources can reveal complex relationships in crop dynamics.
- Technology Integration: These studies often incorporate remote sensing technology, leading to innovative monitoring solutions.
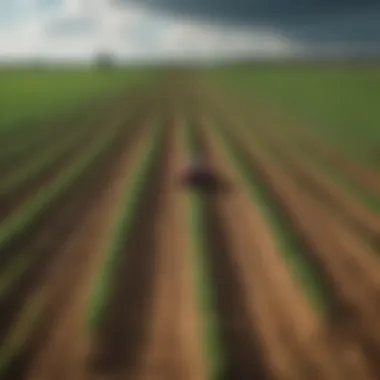
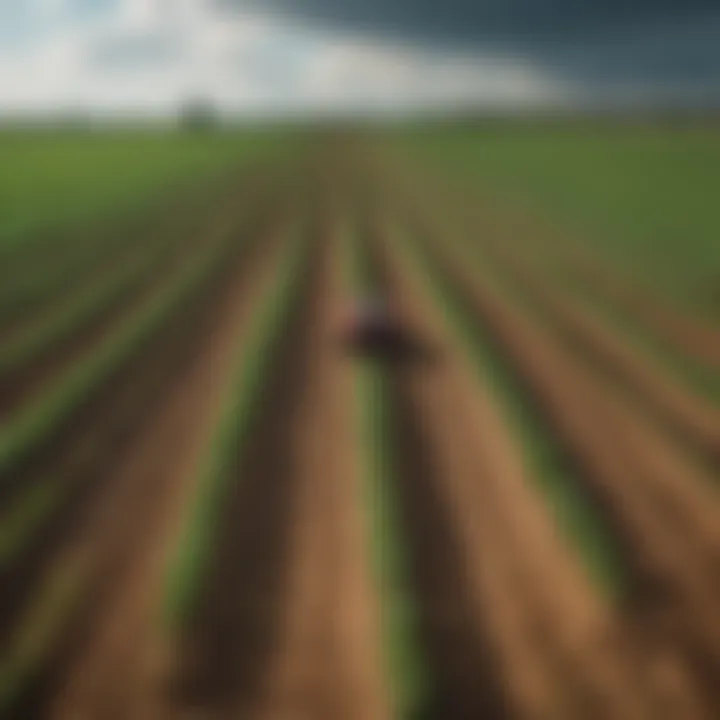
The integration of NDVI with other assessment methods leads to a more comprehensive understanding of agricultural health. This synergy can drive advancements in precision farming and contribute to sustainable practices.
The integration of NDVI with other assessment methods leads to a more comprehensive understanding of agricultural health. This synergy can drive advancements in precision farming and contribute to sustainable practices.
Limitations of NDVI
The Normalized Difference Vegetation Index (NDVI) is a powerful tool for assessing vegetative health and crop performance. However, it is not without its limitations. Understanding these limitations is crucial for scholars, farmers, and industry professionals who depend on this technology for precision agriculture. This section will examine specific environmental factors that can skew NDVI readings, as well as limitations tied to the sensors used in NDVI calculation.
Environmental Factors
NDVI calculations can be heavily influenced by various environmental conditions. One major factor is atmospheric interference, which can alter the reflectance signals received. For example, clouds, dust, and humidity can distort spectral data. These atmospheric conditions may lead to inaccurate readings, thereby hindering the reliability of NDVI assessments.
Additionally, soil background can affect NDVI values. When vegetation cover is low, soil type and color can become significant factors in reflectance. Different soil types can reflect light differently, producing misleading NDVI results. For example, if NDVI is used in a region with a high proportion of dark soil, it may underrepresent the health of the vegetation present.
Moreover, seasonal changes can also present challenges. As crops grow or enter different growth stages, NDVI values can fluctuate, sometimes leading to misinterpretation of crop health. For instance, a green crop may exhibit lower NDVI values if it undergoes a transition phase, resulting in misinformed decisions about irrigation or fertilization.
In summary, environmental factors such as atmospheric conditions, soil characteristics, and seasonal variations can obscure true vegetation health. Accurate interpretation of NDVI data requires awareness of these challenges.
Sensor Limitations
The effectiveness of NDVI is also contingent on the technology and sensors utilized to capture the data. Variability in sensor quality can lead to discrepancies in readings. Different drone or satellite sensors have distinct specifications, leading to variations in spectral resolution and accuracy. For example, some low-cost sensors may not capture the necessary wavelengths effectively, resulting in compromised NDVI calculations.
Another limitation stems from the spatial resolution of the sensors. High spatial resolution may capture minute variations in vegetation health, but it may not be feasible for large-scale agricultural assessments due to increased costs and data management challenges. Conversely, lower resolution sensors can miss critical details, resulting in an oversimplified view of the crop condition.
Furthermore, NDVI calculations depend on the calibration of sensors. If sensors are not properly calibrated, readings can be erroneous. Regular calibration is necessary to maintain accuracy, but it may not always be feasible in practical applications.
Proper sensor operation and calibration are essential to achieving reliable NDVI measurements. Without this, the validity of conclusions drawn from NDVI data diminishes significantly.
Proper sensor operation and calibration are essential to achieving reliable NDVI measurements. Without this, the validity of conclusions drawn from NDVI data diminishes significantly.
Future Directions in NDVI Research
The topic of Future Directions in NDVI Research is essential in understanding how to enhance crop monitoring and management methodologies. NDVI is already a powerful tool for agricultural practices. However, as technology evolves, its integration with other methods and systems becomes crucial for maximizing its potential. Here, we will explore two key aspects: the integration with other technologies and advancements in data analytics.
Integration with Other Technologies
Integrating NDVI with other technologies can revolutionize how farmers monitor and manage their crops. One of the significant advancements is the combination of NDVI with machine learning algorithms. This relationship can enhance the accuracy of predictions regarding crop yield and health. Moreover, when coupled with geographic information systems (GIS), it allows for spatial analysis of crop conditions across different regions.
The importance of this integration extends to real-time data collection. For instance, when drones equipped with NDVI sensors work alongside IoT (Internet of Things) devices, they can provide immediate data about the field conditions. This allows farmers to react swiftly to any abnormalities. The benefits include:
- Improved accuracy in crop assessment
- Faster decision making based on real-time data
- More efficient resource management, leading to reduced waste
Thus, future research should focus on developing these integrations further. Collaborative systems employing NDVI data can significantly bolster sustainable agriculture practices.
Advancements in Data Analytics
The field of data analytics is progressing rapidly, and its impact on NDVI monitoring is noteworthy. The ability to analyze vast amounts of data from NDVI sensors can uncovers hidden patterns that can inform agricultural practices. Advanced analytics techniques, such as predictive modeling, can enhance our understanding of crop condition over time. As a result, farmers can implement interventions at optimal moments.
Additionally, employing artificial intelligence can streamline the data analysis process. Algorithms can sift through complex data sets faster than traditional methods, producing insights that can lead to actionable outcomes. Important considerations for future research in this area include:
- Development of user-friendly platforms for data interpretation
- Ensuring data security and privacy, especially when integrating multiple sources
- Training for farmers and agronomists on data analytics
"The potential of combining NDVI with advanced data analytics can redefine how we understand and manage agricultural practices."
"The potential of combining NDVI with advanced data analytics can redefine how we understand and manage agricultural practices."
Culmination
The conclusion of this article highlights the crucial significance of the Normalized Difference Vegetation Index (NDVI) in modern agriculture, specifically in crop monitoring. NDVI is not just an abstract concept; it directly impacts agricultural practices by providing actionable insights into crop health, soil conditions, and overall productivity. As we reviewed throughout this article, NDVI serves as a valuable tool that empowers farmers and agricultural professionals to make informed decisions.
Summary of Key Points
In summary, the following key points have been discussed:
- Definition and Historical Context of NDVI: Understanding what NDVI is and its development over time establishes a foundation for its application today.
- Understanding Vegetation Indices: NDVI's place within the broader category of vegetation indices emphasizes its relevance.
- Application in Agriculture: NDVI is essential for assessing crop health, predicting yields, and managing irrigation effectively.
- Technologies Utilized for Monitoring: Tools like satellite imagery and drones offer new possibilities and accuracy in data collection.
- Case Studies and Effectiveness: Real-world examples illustrate NDVI's successful implementation in diverse agricultural contexts.
- Limitations of NDVI: Being aware of environmental factors and sensor limitations fosters a realistic understanding of NDVI's capabilities.
- Future Directions: Exploring how NDVI can benefit from advancements in technology and data analytics points toward its evolving role in agriculture.
The Importance of NDVI in Sustainable Agriculture
NDVI is more than a measurement tool; it plays a pivotal role in promoting sustainable agricultural practices. By accurately monitoring crop health, NDVI aids farmers in optimizing inputs such as water, fertilizers, and pesticides. Effective resource management not only reduces costs for farmers but also minimizes the environmental impact associated with over-application of agrochemicals.
As agriculture faces growing challenges, including climate change and population growth, NDVI provides an essential framework for developing resilient farming systems. With its integration into precision agriculture, farmers can implement site-specific management strategies, reducing waste and improving productivity. This alignment with sustainability goals is vital as agriculture seeks to balance food production with ecological preservation.
"The integration of technologies like NDVI into agricultural practices represents a significant step forward in achieving sustainable food production." - Author Unknown
"The integration of technologies like NDVI into agricultural practices represents a significant step forward in achieving sustainable food production." - Author Unknown
By focusing on the data NDVI provides, stakeholders in agriculture can enhance their practices, contributing to a more sustainable future while ensuring that productivity meets the rising demand for food on a global scale.