In-Depth RNA-Seq Data Analysis with DAVID Tool
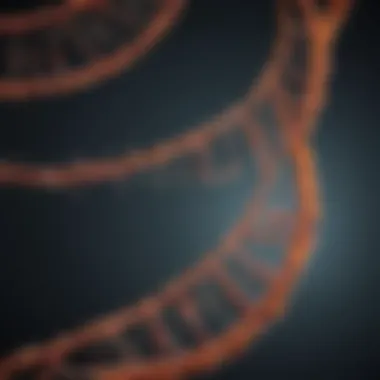
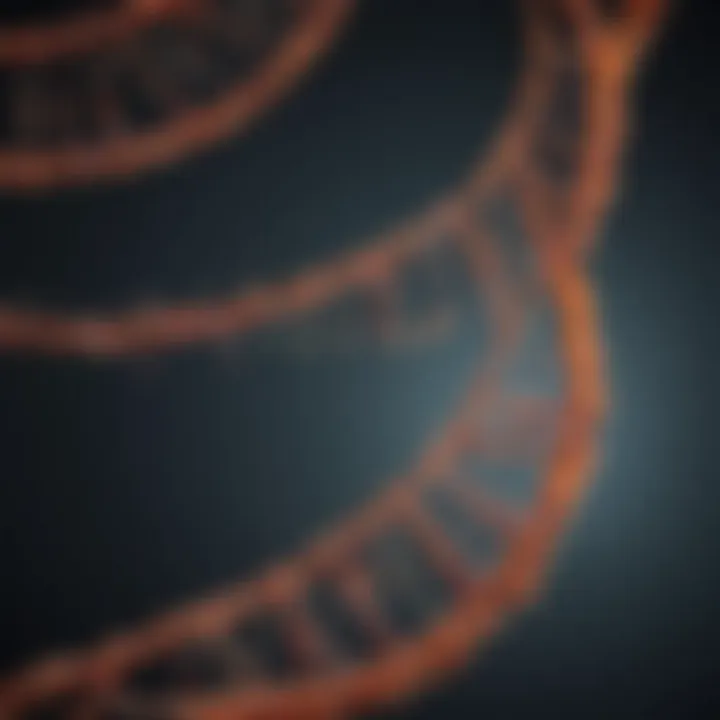
Intro
In recent years, RNA sequencing (RNA-Seq) has become a fundamental tool in genomics and transcriptomics. This technology allows researchers to analyze the transcriptome of organisms in unprecedented detail. One of the most valuable resources for interpreting RNA-Seq data is the Database for Annotation, Visualization, and Integrated Discovery (DAVID). This database offers tools for gene enrichment analysis, functional annotation, and biological interpretation. Understanding how to navigate and utilize DAVID is crucial for researchers and students in bioinformatics.
The goal of this article is to provide a comprehensive overview of the methodologies and techniques involved in analyzing RNA-Seq data with DAVID. Throughout this guide, we will explore the fundamental concepts, current trends in research, and practical applications of DAVID in biological studies. By focusing on essential aspects and case studies, we aim to empower readers with the skills to efficiently leverage DAVID for their research endeavors.
Key Concepts
Definition of the Main Idea
RNA sequencing is a method used to capture and quantify RNA in a sample. The resulting data can be analyzed to understand gene expression levels, alternative splicing, and other transcriptomic phenomena. DAVID assists in this process by providing a set of tools designed for functional annotation and enrichment analysis. These features enable users to connect genes of interest to biological functions and pathways.
Overview of Scientific Principles
At the core of gene enrichment analysis is the concept of identifying overrepresented gene categories. This may include biological process categories, cellular components, or molecular functions. DAVID uses statistical methods to determine if the observed counts of genes in specific categories differ significantly from what would be expected by chance.
Several key principles underpin RNA-Seq analysis with DAVID:
- Data Preprocessing: Raw RNA-Seq data must be processed to ensure accuracy. This includes quality control, alignment to a reference genome, and quantifying gene expression.
- Enrichment Analysis: DAVID offers tools to identify significant gene ontology terms or pathways that are enriched in a selected gene list.
- Integration with Other Databases: DAVID can integrate data from other databases, which enhances the contextual understanding of the analyzed genes.
"Understanding the principles of RNA-Seq analysis is essential for effectively utilizing DAVID's powerful features in research."
"Understanding the principles of RNA-Seq analysis is essential for effectively utilizing DAVID's powerful features in research."
Current Research Trends
Recent Studies and Findings
Recent advancements in RNA-Seq technology have broadened its applicability across various fields of research. Studies are increasingly utilizing DAVID to analyze complex datasets. The results have led to insights in oncology, microbiomics, and developmental biology. Notably, research has demonstrated how DAVID can elucidate cancer-associated pathways, providing a clearer understanding of tumor biology.
Significant Breakthroughs in the Field
One significant breakthrough is the integration of machine learning techniques with RNA-Seq analysis. This approach enhances the predictive power of gene expression data. Researchers are using DAVID to analyze predicted gene lists generated by machine learning models, which can reveal novel biological insights.
In summary, the application of DAVID in RNA-Seq analysis is not just limited to basic functions. Current research trends indicate a widening scope, where DAVID serves as a critical tool in modern genomic investigations.
Prelims to RNA Sequencing
RNA sequencing (RNA-Seq) has emerged as a powerful tool in genomics, enabling researchers to analyze the transcriptome of organisms at an unprecedented scale. This section provides a foundation for understanding how RNA-Seq operates and why it is an essential method in contemporary biological research. The significance of RNA-Seq goes beyond mere data generation; it offers insights into gene expression patterns, alternative splicing events, and potential regulatory mechanisms.
The implementation of RNA-Seq has democratized transcriptional analysis, making it more accessible and affordable for laboratories around the world. With its high throughput capabilities, RNA-Seq can simultaneously analyze thousands of genes in a single experiment. This capability makes it an indispensable method for studying cellular responses to various stimuli, developmental processes, and disease mechanisms.
Understanding RNA Sequencing Technology
RNA sequencing technology involves several steps, starting from the extraction of RNA from biological samples to the actual sequencing itself. During this process, RNA is typically converted into cDNA (complementary DNA) using reverse transcription. Followed by cDNA library preparation, sequencing techniques such as Illumina or PacBio are employed to generate sequencing reads.
- Sample Preparation: RNA extraction is critical. The quality of RNA is key to obtaining reliable results.
- cDNA Synthesis: Reverse transcription converts RNA to cDNA, essential for sequencing.
- Sequencing: Multiple platforms exist, each with its own advantages and weaknesses, influencing data quality and coverage.
The raw sequencing data obtained is processed through a series of bioinformatics steps including quality control, alignment, and quantification to generate meaningful insights.
Importance of RNA-Seq in Genomic Research
RNA-Seq plays a pivotal role in genomic research due to its high sensitivity and resolution. It allows for the comprehensive assessment of gene expression, uncovering insights that are often overlooked by traditional methods like microarrays.
- Comprehensive Data: Unlike microarrays, which typically require prior knowledge of genes, RNA-Seq provides unbiased data on all expressed genes.
- Data Depth: The depth of sequencing can identify lowly expressed genes, which is crucial in diverse biological processes.
- Novel Transcripts: RNA-Seq helps in discovering novel transcripts that may be vital in various biological functions.
Overall, its capabilities have advanced fields such as cancer biology, developmental biology, and evolutionary biology by facilitating deeper understandings of complex biological systems.
RNA-Seq not only enhances our understanding of gene expression but also introduces new opportunities for therapeutic interventions by identifying potential targets for drug development.
RNA-Seq not only enhances our understanding of gene expression but also introduces new opportunities for therapeutic interventions by identifying potential targets for drug development.
Intro to the DAVID Database
The Database for Annotation, Visualization, and Integrated Discovery, commonly known as DAVID, serves as a pivotal tool in the realm of bioinformatics. This section aims to elucidate its functionality and importance, particularly in the context of RNA sequencing data analysis. Researchers dealing with vast sets of data will find that DAVID streamlines the process of gene annotation and functional enrichment analysis. It acts as a bridge, translating raw data into meaningful biological insights, thus playing a fundamental role in the interpretation of RNA-Seq results.
Utilizing DAVID efficiently can enhance the understanding of gene functions and their associations with biological pathways. It's crucial to grasp how this resource can transform complex genomic information into usable forms, making it indispensable for students, researchers, and professionals alike.
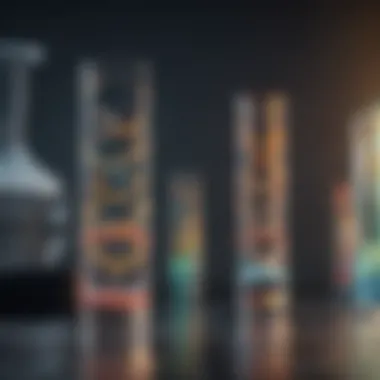
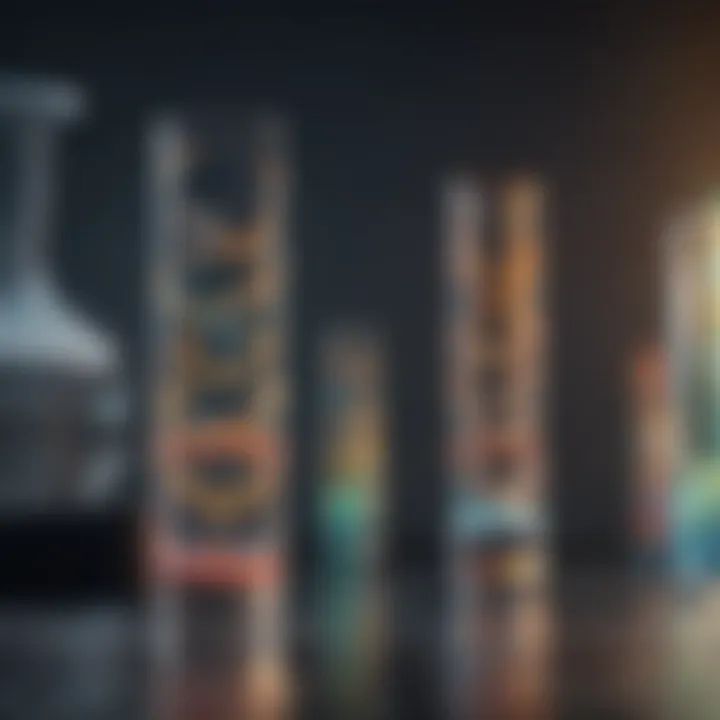
Overview of DAVID Functionality
DAVID provides an array of tools designed to facilitate data analysis. The platform specializes in functional annotation, meaning it offers users the ability to systematically categorize gene lists based on their functions. Users can upload gene identifiers, and DAVID matches them with a vast database of biological knowledge. This helps in identifying enriched biological themes or pathways in their data.
A key functionality includes the interactive web interface that allows for seamless navigation through different analytical modules. Users can perform annotation clustering, in which related functions are grouped together, simplifying the interpretation of large datasets. The functionality of DAVID hence provides clarity to researchers trying to decode complex biological information.
Key Features of DAVID
DAVID boasts several key features that enhance its utility for RNA-Seq data analysis:
- Comprehensive Annotation: Users benefit from an extensive database incorporating multiple species, allowing for broad applicability in research.
- Gene Set Enrichment Analysis: This feature enables the identification of biological pathways significantly associated with gene alterations, providing insights into their functional implications.
- Visualization Tools: DAVID facilitates graphical representations of analysis results, including charts and graphs, which help in quick assimilation of complex data.
- User-Friendly Interface: The straightforward design of the tool allows users of varying expertise to navigate through the resources effortlessly.
- Community Support and Documentation: A wealth of tutorials and community forums are available for users to seek assistance and learn through shared experiences.
These features make DAVID an essential resource for anyone working with RNA-Seq data, aiding both novice and experienced researchers in their analytical journeys.
Preparing RNA-Seq Data for DAVID Analysis
In the analysis of RNA sequencing data, preparing the data correctly is a foundational step that can influence the outcomes of subsequent analyses. Proper preparation ensures that the data integrity is maintained and optimizes the usefulness of tools like the DAVID database. With the ever-increasing complexity of biological datasets, the emphasis on meticulous preparation cannot be overstated. Key elements such as quality control, normalization techniques, and appropriate formatting must be systematically considered to facilitate robust and meaningful analysis.
Quality Control of RNA-Seq Data
Quality control (QC) is the first crucial stride in ensuring that RNA-Seq data is reliable. Poor-quality data can lead to incorrect conclusions, which may affect the validity of research findings. Making use of QC tools, researchers can evaluate data by checking for several factors including sequencing depth, read quality, and contamination.
It is common to visualize quality metrics using software like FastQC. Here are some essential aspects of QC:
- Read Quality: Assessing the base quality scores to identify potential errors in the sequences.
- Adapter Content: Checking for the presence of adapters, which can introduce biases in the data.
- Duplication Levels: Evaluating duplicated reads, which may skew the analysis of gene expression levels.
In summary, rigorous QC is a gateway to assessing and confirming the reliability of RNA-Seq datasets, laying the groundwork for accurate downstream analysis, including DAVID’s enrichment features.
Normalization Techniques
Normalization addresses the variability that can occur in RNA-Seq data due to technical factors, rather than actual biological differences. Several normalization methods exist, each suited to different types of datasets. Implementing proper normalization can vastly improve the interpretability of the results.
Some common normalization techniques include:
- Total Count Normalization: Adjusts the counts to reflect the library size.
- Relative Log Expression: This method emphasizes log transformation, allowing for a more balanced comparison between samples.
- Quantile Normalization: Ensures that the distribution of gene expression levels is the same across all samples, which is particularly effective in high-throughput datasets.
Utilizing these techniques not only assures that the data is comparable across samples but also enhances the quality of results derived from DAVID's functional analysis tools.
Data Formatting for DAVID
Once the RNA-Seq data is subjected to quality control and normalized, formatting the data accurately is crucial for seamless input into the DAVID platform. DAVID requires specific formats to process data efficiently. Missing or incorrectly formatted data can lead to errors that prevent a meaningful analysis from being performed.
The key considerations for data formatting include:
- Data Type: Ensure that the gene identifiers used are compatible with DAVID (for example, using Entrez Gene IDs).
- Input File Type: Usually, a plain text file or Excel format is recommended for ease of use.
- Organized Layout: Structuring the file to have clear headers for gene IDs and associated expression levels helps in proper mapping within DAVID.
Proper formatting not only facilitates a smooth data submission but also helps in retrieving precise and informative results from the DAVID database, ultimately leading to successful downstream gene enrichment and functional annotation.
Conducting Enrichment Analysis Using DAVID
Conducting enrichment analysis using the Database for Annotation, Visualization, and Integrated Discovery (DAVID) is a fundamental step in RNA-Seq data analysis. This process allows researchers to identify which biological functions, pathways, or diseases are represented in their data. By employing DAVID, scientists can extract meaningful biological insights from gene lists generated through RNA sequencing.
The enrichment analysis essentially tests the over-representation of gene sets among the inputted gene list compared to a background population. This helps identify not just which pathways are involved, but also reveals the biological relevance behind the observed changes in gene expression.
Steps to Perform Gene Enrichment
To initiate the enrichment analysis, follow these steps:
- Prepare your gene list: Start with a list of differentially expressed genes from your RNA-Seq analysis. Ensure that the gene identifiers are compatible with DAVID’s database, which primarily supports Entrez Gene IDs.
- Access the DAVID interface: Navigate to the DAVID website. Log in or create an account, if needed, to access the full features of the platform.
- Upload your gene list: Select the option to upload the gene list. You can paste the list directly or upload a text file, depending on your preference.
- Select the appropriate gene identifier: Make sure to choose the correct identifier type to match your data. This step reduces any errors in mapping your genes to the DAVID database.
- Choose the analysis type: Opt for a functional annotation or a gene enrichment analysis, based on your research objectives.
- Review and submit: After configuring your analysis parameters, review your selections and submit the analysis.
This methodical approach ensures that you accurately conduct the enrichment analysis, laying a solid foundation for meaningful interpretations.
Choosing Appropriate Background Genes
When conducting enrichment analysis, specifying the appropriate background gene set is crucial. The background set serves as a reference from which to judge the significance of the observed enrichment. Here are important considerations:
- Select a relevant background: The background genes should be chosen based on the experimental context. For instance, for studies focusing on specific tissues or conditions, using the transcriptomes for those tissues can provide a clearer perspective on enrichment results.
- Use a global background if necessary: If specific background data are not available, a global background that includes all expressed genes can be utilized. However, this might lead to less precise results compared to tissue-specific gene sets.
- Avoid bias: A well-chosen background should not bias the analysis. Ensure that the background gene list reflects the same experimental conditions as the gene list being analyzed to avoid skewed results.
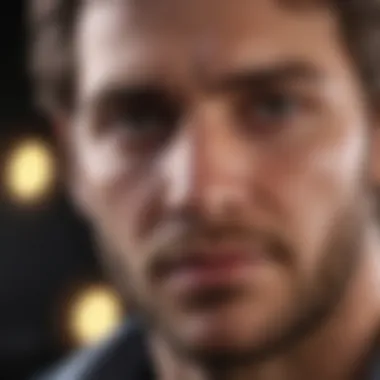
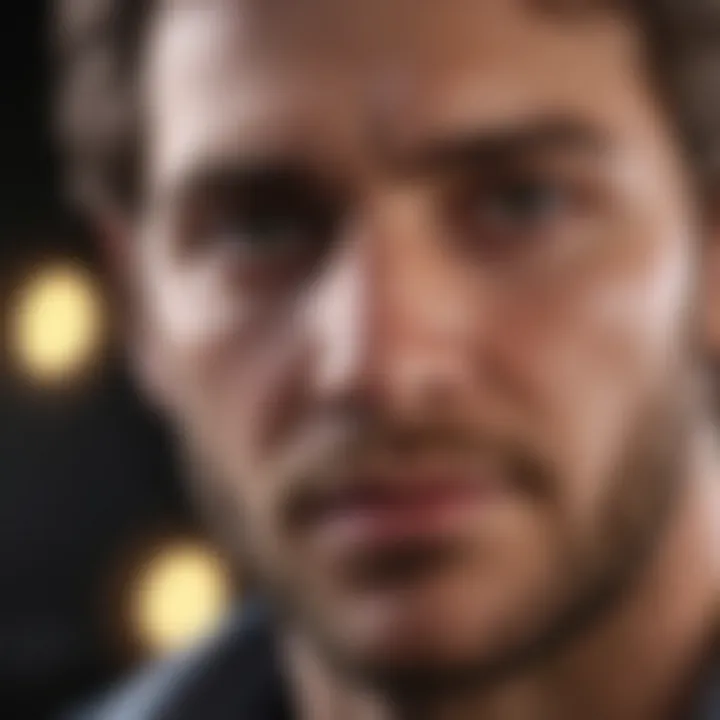
Interpreting Enrichment Results
Once the enrichment analysis is complete, interpreting the results is essential for drawing meaningful conclusions. Acceptable methods for analysis should include:
- Review significant terms: Focus on the top enriched Gene Ontology (GO) terms and pathways. Look at the p-values adjusted for multiple testing, such as the Benjamini-Hochberg method, to determine significance.
- Explore biological relevance: It is crucial to analyze which biological processes and pathways are most relevant to your study. Make connections between these pathways and the diseases or biological systems of interest.
- Visualize findings: Utilize DAVID’s built-in visualization tools, such as the bar charts and dendrograms, to grasp the relationships between enriched terms. This can help identify key pathways that may warrant further investigation.
By thoroughly understanding these stages of enrichment analysis within DAVID, researchers can gain deeper biological insights from their RNA-Seq data. These analyses not only highlight important pathways but also facilitate further research into their roles in various biological contexts.
Effective enrichment analysis with DAVID opens doors to new hypotheses and potential therapeutic targets, paving the way for advancements in biomedical research.
Effective enrichment analysis with DAVID opens doors to new hypotheses and potential therapeutic targets, paving the way for advancements in biomedical research.
Functional Annotation and Biological Interpretation
Functional annotation and biological interpretation are crucial components of RNA-Seq data analysis. This process allows researchers to assign biological meaning to the multitude of genes identified in RNA sequencing experiments. The effectiveness of gene expression studies hinges on the ability to translate raw data into insights that can inform biological understanding and experimental design.
Functional annotation provides a means to categorize genes based on their known or predicted biological roles. This categorization encompasses aspects such as protein function, cellular localization, and participation in specific biological pathways. By understanding these roles, researchers can identify which genes are overexpressed or underexpressed relative to a control, which can shed light on the underlying mechanisms of diseases or developmental processes.
Moreover, biological interpretation goes beyond mere categorization; it integrates annotations with experimental data to derive meaningful conclusions. This interpretative phase is essential for contextualizing findings within broader biological systems and enables hypotheses generation about cellular behavior or pathological states. The implications are far-reaching, influencing study designs and further experimental validations.
Using DAVID for Functional Annotation
The Database for Annotation, Visualization, and Integrated Discovery (DAVID) is an invaluable tool for functional annotation. It allows users to upload gene lists and retrieve extensive information about the genes’ functions and related biological traits. The platform simplifies the annotation process by employing various databases, including Gene Ontology, KEGG pathways, and Uniprot, thus creating a comprehensive view of gene function.
When using DAVID, researchers follow a basic workflow:
- Upload the list of genes of interest.
- Select appropriate background genes.
- Choose the correct annotation category.
- Analyze the results to identify significant annotations.
This process ensures that the analysis is grounded in reliable data sources, enhancing the validity of the results.
Identifying Biological Pathways
Identifying biological pathways is another key application of DAVID. By mapping genes to known pathways, researchers can discover how their genes of interest integrate into biological networks. Understanding these networks is pivotal for unraveling the complexities of biological processes and can direct future experimental inquiries.
In DAVID, pathway analysis can be approached through:
- Pathway enrichment analysis: This technique identifies pathways that are significantly overrepresented in the gene list compared to a background set.
- Functional clusters: This feature groups similar pathways or annotations, offering insights into interconnected biological functions and mechanisms.
By utilizing these analyses, researchers can draw connections between gene expression profiles and pathway activity, providing a clearer picture of biological systems at play.
Visualizing Results in DAVID
Visualizing results is essential for interpreting complex data effectively. DAVID provides various visualization options that can enhance understanding and communication of findings. Visualization tools in DAVID include bar charts, pie charts, and dendrograms, which can illustrate the distribution of gene annotations, pathway involvement, and relationship among different biological processes.
For instance, a simple bar chart can display the number of enriched functional annotations, making it easier for users to grasp which functions are represented most prominently. Similarly, dendrograms can help visualize clusters of related biological pathways, allowing researchers to identify overarching themes in their data.
Challenges in RNA-Seq Data Analysis
In the field of bioinformatics, analyzing RNA sequencing data presents various challenges that researchers must navigate. This section emphasizes the importance of understanding these challenges, particularly when using tools such as the Database for Annotation, Visualization, and Integrated Discovery (DAVID). A thorough grasp of these challenges can enhance the validity of the analysis and improve the quality of the insights drawn from the data.
Limitations of DAVID
While DAVID is a powerful tool for gene enrichment analysis, it has certain limitations that researchers need to be aware of:
- Data Applicability: DAVID is mainly optimized for human and mouse data. Other species may not have comprehensive annotation, limiting the analysis.
- Statistical Rigor: The statistical methods employed by DAVID may not account for every variable in complex datasets. This means that some results may not reflect true biological significance.
- Gene Annotation Variability: Changes in gene annotations can lead to inconsistencies in results over time. Researchers should verify the gene IDs and their annotations in their datasets to ensure accuracy.
- User Experience: New users may find the interface overwhelming, making it difficult to extract the desired information effectively. A learning curve exists, which can deter efficient use of the tool.
Common Pitfalls in Enrichment Analysis
Enrichment analysis is a critical step in interpreting RNA-Seq data, but there are some common pitfalls:
- Background Selection: Often, researchers choose inappropriate background gene lists, which can skew enrichment results. Selecting a relevant background is crucial for accurate interpretation.
- Multiple Testing Correction: Failing to account for multiple hypothesis testing can lead to false positives. Proper correction methods should be applied to control for Type I errors.
- Biological Interpretation: Over-interpreting results or drawing hasty conclusions from enrichment analysis can mislead further research. It is important to complement findings with biological context and prior knowledge.
In summary, understanding the limitations of DAVID and avoiding common pitfalls in enrichment analysis are essential for producing reliable and meaningful results in RNA-Seq studies.
In summary, understanding the limitations of DAVID and avoiding common pitfalls in enrichment analysis are essential for producing reliable and meaningful results in RNA-Seq studies.
Researchers must approach RNA-Seq data analysis thoughtfully. By recognizing challenges and constraints, they can employ tools more effectively and interpret results with greater accuracy.
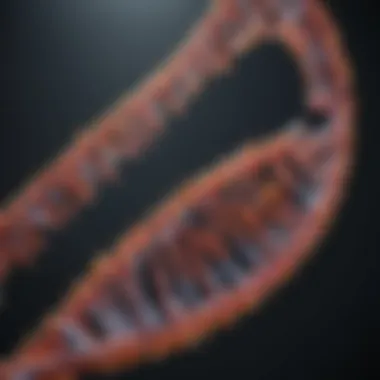
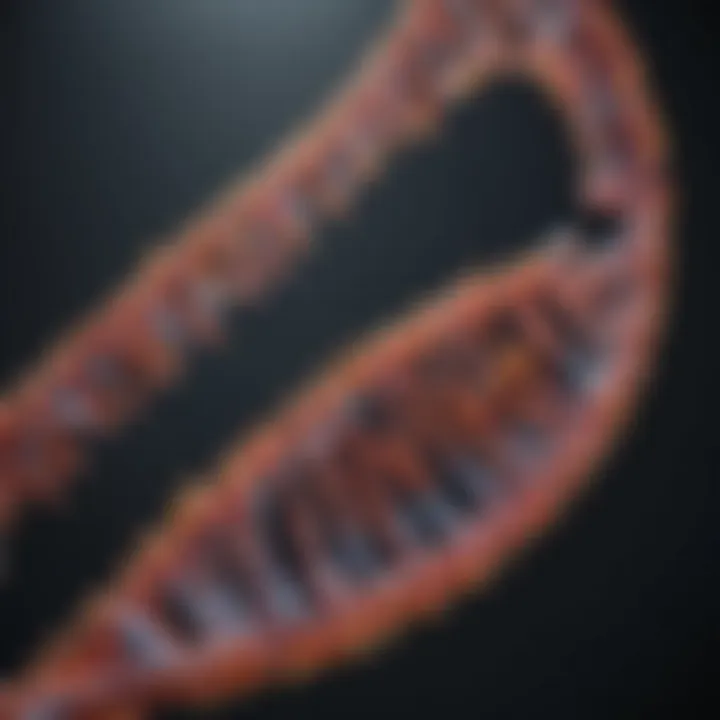
Case Studies in RNA-Seq Analysis Using DAVID
In the realm of RNA-Seq data analysis, case studies serve as an essential bridge between theoretical knowledge and practical application. They offer concrete examples that demonstrate how to utilize the DAVID database effectively. This section emphasizes the relevance of case studies in providing insights into real-world scenarios where DAVID has made significant contributions. By examining specific instances, researchers can grasp the complexity of RNA-Seq analysis while understanding the practical implications and outcomes of using DAVID.
Implementing DAVID in research can yield profound benefits. It not only enhances the analysis process but also facilitates a deeper understanding of biological context. The insights gained from these case studies can guide future research endeavors, offering a template for methodological approaches and highlighting important considerations when using DAVID.
Case Study One: Cancer Research
Cancer research benefits greatly from RNA-Seq analysis, as it allows for a detailed exploration of gene expression profiles in tumor samples. By employing DAVID, researchers can perform gene enrichment analysis, identifying pathways and functional categories that are significantly related to various cancers.
One notable case study involved the analysis of gene expression data from breast cancer tissues. Researchers used DAVID to categorize differentially expressed genes and to identify crucial pathways involved in cancer progression. The findings revealed that several genes were associated with cell proliferation and apoptosis, two critical processes in cancer biology.
The utilization of DAVID provided multiple layers of understanding:
- Functional Insights: Researchers could link specific genes with biological functions.
- Pathway Identification: Key cancer-related pathways were highlighted, facilitating future studies on targeted therapies.
- Network Analysis: Connections between genes were visualized, allowing for a comprehensive view of the molecular landscape in cancer.
By analyzing cancer RNA-Seq data through DAVID, researchers established a blueprint for future studies. The combination of gene expression profiling and enrichment analysis proved invaluable in advancing cancer research methodologies.
Case Study Two: Developmental Biology
In developmental biology, understanding gene expression changes during various stages is crucial. RNA-Seq analysis using DAVID can unveil the intricate networks involved in organismal development. A prominent case study explored the gene expression profiles during the embryonic stages of a model organism, Xenopus laevis.
Researchers employed DAVID to analyze the differentially expressed genes at specific developmental time points. The insights gained from this analysis were remarkable:
- Temporal Expression Patterns: Identifying genes that are upregulated or downregulated at particular stages of development.
- Functional Categorization: Using DAVID's functional annotation tools, researchers categorized these genes into developmental pathways, enhancing the understanding of embryogenesis.
- Comparative Analysis: DAVID allowed for the comparison with existing data, providing context to the findings and suggesting evolutionary relationships.
The comprehensive insights from this study underscore the value of integrating DAVID into developmental biology research. The ability to access and analyze multifunctional data facilitates a deeper comprehension of the genetic underpinnings of development.
Case studies illuminate how DAVID serves as a pivotal resource in translating RNA-Seq data into meaningful biological interpretations, enhancing both cancer and developmental research.
Case studies illuminate how DAVID serves as a pivotal resource in translating RNA-Seq data into meaningful biological interpretations, enhancing both cancer and developmental research.
Future Directions in RNA-Seq Analysis
In the evolving field of genomics, the analysis of RNA sequencing data is continually progressing. This section focuses on the future directions in RNA-Seq analysis, highlighting emerging technologies and the enhancements that can benefit tools like DAVID. As researchers enhance their abilities to interpret complex RNA-Seq data, considering these advancements becomes critical in ensuring accuracy and relevance in biological research.
Emerging Technologies in RNA-Seq
Recent advancements in RNA sequencing technologies have significantly changed the landscape of genomics. Techniques that were once limited due to cost or complexity are becoming more accessible and efficient.
Key innovations include:
- Single-Cell RNA Sequencing (scRNA-Seq): This technology allows for the analysis of gene expression at the single-cell level. It provides a detailed view of cellular heterogeneity, which is essential in understanding complex tissues and diseases like cancer.
- Long-Read Sequencing: Technologies like Oxford Nanopore and PacBio enable longer reads compared to traditional methods. They allow for better resolution of transcript isoforms and structural variations, which are often overlooked in short-read analyses.
- RNA Editing Detection: New methodologies are emerging that can detect RNA editing events with high sensitivity. This is important for understanding post-transcriptional modifications that can affect gene regulation and function.
These technologies collectively enhance the depth and quality of RNA-Seq data, leading to more precise biological interpretations. The integration of these emerging methods into existing workflows like DAVID will allow researchers to generate richer datasets and more actionable insights.
Enhancing Tools like DAVID
As RNA-Seq technologies evolve, so too must the analytical tools that support them. There is a need to enhance platforms like DAVID for better data integration and analysis capabilities.
Considerations for enhancing tools include:
- User Interface Improvements: Simplifying the user interface can make it easier for researchers with varying levels of expertise to use DAVID effectively. Streamlined workflows and clear instructions can reduce the learning curve.
- Integration with Other Databases: Enhancing the ability to integrate data from other databases will improve the context and relevance of the analyses. For example, linking DAVID with databases like Gene Ontology or KEGG pathways would provide richer annotation features.
- Automation and Machine Learning: Incorporating machine learning algorithms can help automate the analysis of RNA-Seq data. This would enable faster processing, identification of patterns, and ultimately more effective enrichment analyses.
End
The conclusion of this article emphasizes the pivotal role of DAVID in the landscape of RNA sequencing analysis. This section synthesizes the insights gathered throughout the article, underscoring how varied methods and interpretations enhance the usefulness of DAVID. By engaging with the nuances of RNA-Seq data, researchers can tap into a rich reservoir of biological understanding. Thus, a detailed comprehension of the analysis process not only enriches individual studies but also contributes significantly to the collective knowledge in genomics.
Summarization of Key Insights
In review, several key points arise from our exploration of DAVID and RNA-Seq data analysis:
- Functionality of DAVID: The tools provided by DAVID allow for comprehensive gene enrichment and functional annotation, which are crucial for understanding biological phenomena.
- Data Preparation: Quality control and normalization steps are integral to refining raw RNA-Seq data. Preparing data correctly is the foundation of effective analysis.
- Enrichment Analysis: The methodologies for conducting enrichment analysis are vital. This step helps reveal significant biological pathways, providing insights that raw data alone cannot offer.
- Visualization Techniques: Effective visualization tools within DAVID aid in interpreting complex data sets, allowing for a clearer understanding of research findings.
Each of these insights contributes to a more nuanced grasp of both the capabilities of the DAVID database and the implications of RNA-Seq research.
Implications for Future Research
Reflecting on the insights discussed, the implications for future research are profound:
- Continued Development of Tools: As technologies evolve, enhancements in tools like DAVID will likely emerge. Researchers will benefit from increased functionalities, which may allow for deeper insights in various biological contexts.
- Interdisciplinary Approaches: Future studies should aim to integrate findings from RNA-Seq data with other genomic technologies. This interdisciplinary approach can lead to a more comprehensive understanding of gene function and regulation.
- Enhanced Data Sharing: Encouraging open access to RNA-Seq datasets will promote collaboration and knowledge sharing. This openness can lead to novel findings and further enhance the understanding of complex biological systems.
In summary, the conclusion encapsulates the importance of following robust methodologies in RNA-Seq data analysis with DAVID. Considerations for ample research methodologies and future advancements stand to propel the field forward, enriching both academic inquiry and practical applications in biology.