Predicting Chemical Reactions: Insights and Methodologies
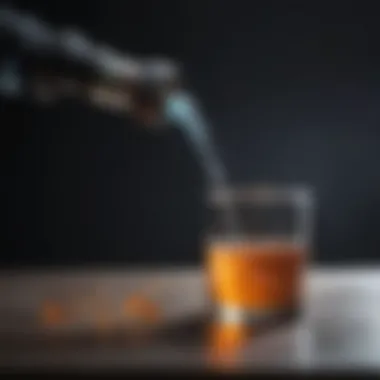
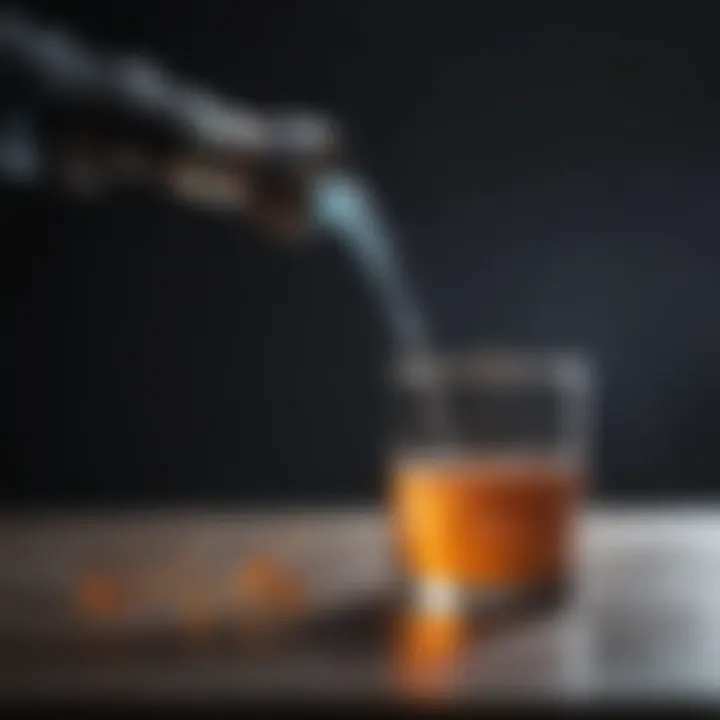
Intro
Predicting chemical reactions is an ever-evolving area of study in the fields of chemistry and material science. With various methodologies available, researchers and professionals strive to enhance their understanding of chemical behavior. Accurate predictions allow scientists to anticipate outcomes and design experiments more effectively. This in-depth examination will touch on essential principles, methodologies, current research trends, and practical applications in real-world scenarios.
Key Concepts
Definition of the Main Idea
Predicting chemical reactions involves using systematic methods to forecast the products and pathways of chemical processes. This can be expressed quantitatively or qualitatively through computational models or experimental observations. Essentially, the aim is to grasp how different chemicals interact under various conditions.
Overview of Scientific Principles
The predictability of chemical reactions is grounded in several scientific principles:
- Thermodynamics: This branch studies energy changes throughout reactions, helping to determine spontaneity.
- Kinetics: Understanding reaction rates offers insight into how quickly and efficiently reactions occur.
- Quantum Mechanics: Models based on quantum principles provide a detailed understanding of molecular interactions.
Each of these principles plays a critical role in developing accurate predictive methodologies and frameworks.
Current Research Trends
Recent Studies and Findings
Recent research has focused on improving computational models. Innovations such as machine learning algorithms have begun to revolutionize the accuracy of predictions. For instance, studies have shown that deep learning frameworks can analyze vast data sets of reaction outcomes and identify correlations that human researchers might overlook.
Significant Breakthroughs in the Field
Several notable breakthroughs in predictive chemistry include:
- Development of Software Tools: Programs like Gaussian and ORCA have made it easier to model complex chemical systems.
- Integration of AI: Artificial intelligence has enhanced pace and accuracy in predicting reaction pathways.
"Understanding the principles of chemical behavior not only informs theoretical research but also fosters practical applications in fields like pharmaceuticals and materials development."
"Understanding the principles of chemical behavior not only informs theoretical research but also fosters practical applications in fields like pharmaceuticals and materials development."
As the landscape of predictive chemistry advances, ongoing developments will continue to shape the trajectory of scientific research and its applications.
Prelude to Predicting Chemical Reactions
Predicting chemical reactions is an essential aspect of chemistry that has far-reaching implications across various scientific disciplines. The ability to anticipate how substances interact allows researchers and practitioners to develop new materials, pharmaceuticals, and sustainable practices. This article aims to explore the methodologies and principles involved in predicting chemical reactions, offering insights into both theoretical foundations and practical applications.
Importance of Reaction Predictions
The importance of predicting chemical reactions cannot be overstated. Accurate predictions can lead to significant advancements in multiple fields. For instance, in pharmaceuticals, understanding potential reaction pathways can expedite drug development, ensuring safety and efficacy. Enhanced reaction predictions can also support materials science by enabling the design of innovative materials with desired properties. Furthermore, environmental chemistry relies on reaction predictions to evaluate the effects of various compounds, aiding in regulatory compliance and pollution control.
- Reaction predictions influence:
- Drug discovery timelines and success rates.
- Material properties and performance optimization.
- Environmental impact and regulatory assessments.
In summary, the capacity to predict reactions effectively is a driving force for innovation and progress. This capability enhances scientific understanding and leads to technological breakthroughs that address real-world challenges.
Historical Context
The journey of predicting chemical reactions spans several centuries, reflecting the evolving nature of chemistry itself. Early efforts were primarily qualitative, relying on empirical observations. For example, alchemists made extensive observations but lacked a robust theoretical framework. The transition to modern chemistry began with figures like Antoine Lavoisier, who established the law of conservation of mass, laying foundational principles for chemical equations.
The development of the periodic table by Dmitri Mendeleev introduced a systematic approach that helped predict the properties of unknown elements. As the field progressed, the advent of thermodynamics in the 19th century allowed scientists to understand the energy changes during reactions, further enhancing predictive capabilities.
In the 20th century, quantum chemistry emerged, bringing with it advanced mathematical models that provided more accurate predictions of molecular behavior. As computational power increased, models became increasingly complex and precise, paving the way for current methodologies that integrate machine learning and high-throughput screening.
This historical perspective underscores the dynamic nature of predicting chemical reactions—a field that continuously evolves in response to new scientific insights and technological advancements.
Fundamental Concepts in Chemistry
Understanding the fundamental concepts in chemistry is essential for predicting chemical reactions. These concepts form the backbone of chemical interactions and reactions. A solid grounding in these principles equips researchers and students with the knowledge to approach complex chemical predictions systematically and effectively.
The significance of fundamental concepts can be seen in their wide applications, from academia to industry. They influence everything from the design of new materials to the development of drugs.
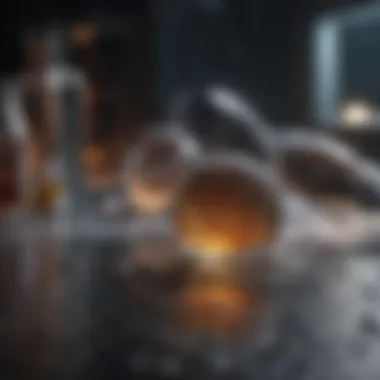
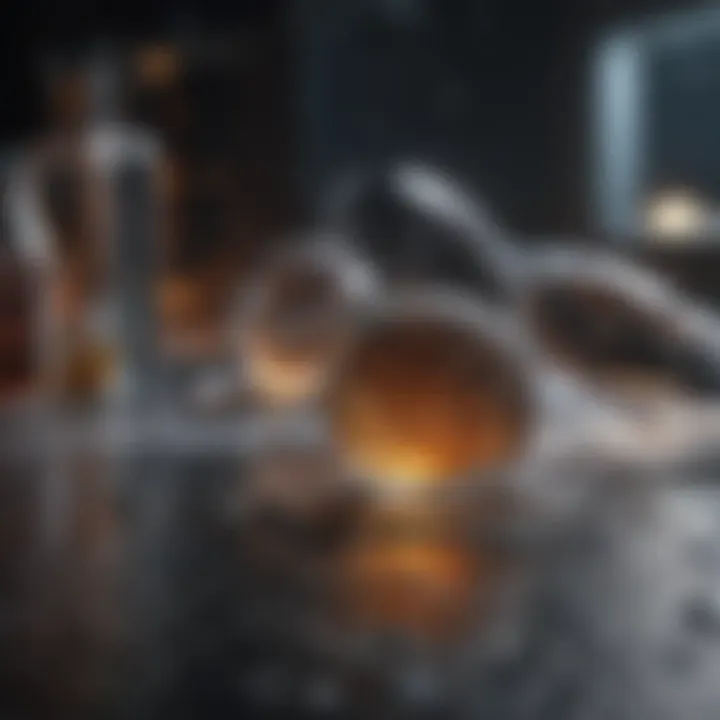
Chemical Bonds and Interactions
Chemical bonds are the forces that hold atoms together in a molecule. They can be classified broadly into ionic, covalent, and metallic bonds. Each bond type has distinct properties, affecting the stability and reactivity of the molecules formed. Understanding these interactions is crucial when assessing reaction pathways and outcomes.
- Ionic bonds arise from the electrostatic attraction between charged ions. They are usually found in solid salts and have high melting and boiling points.
- Covalent bonds involve the sharing of electrons between atoms. These bonds can form strong, stable molecules found in organic compounds. Their complexity greatly influences the possible reactions.
- Metallic bonds lead to the formation of metals characterized by their malleability and electrical conductivity.
The interaction between bonds also informs reaction predictions. For instance, covalent bonds may break during a reaction, allowing for the rearrangement of atoms. Understanding the nature of these bonds helps chemists foresee what may occur during chemical processes.
Reaction Mechanisms
Reaction mechanisms explain how reactants transform into products at a molecular level. This area of study delves into the steps involved in a reaction and how energy changes factor into these processes. Each step in a mechanism can involve breaking and forming bonds and can be influenced by catalysts.
Key components of reaction mechanisms include:
- Transition states: These are high-energy states that occur during the transformation of reactants to products.
- Rate-determining steps: This is the slowest step in the mechanism that dictates the overall rate of reaction.
- Intermediates: These are transient species formed during the reaction but not consumed in the final products.
By thoroughly understanding reaction mechanisms, chemists can make informed predictions about reaction rates and product yields. This knowledge is imperative for reaching accurate predictions in complex reaction scenarios.
Theoretical Approaches to Prediction
The theoretical approaches to predicting chemical reactions form the backbone of modern chemistry. Understanding these methodologies is essential for chemists seeking to forecast reaction behavior accurately. These approaches rely on principles from various disciplines, including physics, mathematics, and computational science. Their importance lies in the ability to simplify complex reactions into manageable frameworks, allowing for more accurate predictions.
Adopting theoretical approaches equips researchers with the tools to understand various aspects of a reaction, such as thermodynamics, kinetics, and quantum mechanics. Each of these elements provides insight into different phases of a chemical reaction, revealing how reactants transform into products and the rates at which these transformations occur. Key benefits include the enhancement of experimental designs and the optimization of reaction conditions, ultimately leading to more efficient and economical chemical processes.
Thermodynamics in Reaction Prediction
Thermodynamics plays a crucial role in predicting which chemical reactions are feasible. This field examines the energy changes that occur during a reaction, specifically focusing on concepts like enthalpy, entropy, and Gibbs free energy. By evaluating these factors, chemists can determine whether a reaction will proceed spontaneously or require external energy input.
- Enthalpy: It represents the total heat content of a system. A reaction with a negative change in enthalpy is considered exothermic, while a positive change indicates an endothermic reaction.
- Entropy: This quantifies the disorder within a system. An increase in entropy suggests that a reaction is likely to occur, as systems favor greater disorder over time.
- Gibbs Free Energy: This is the ultimate indicator of spontaneity. A reaction is favorable when the change in Gibbs free energy is negative.
By applying these principles, chemists can create predictive models that guide experimental work. Thermodynamics also allows researchers to span a wide range of conditions, assisting in understanding not just if, but how reactions occur.
Kinetics and Rate Laws
Kinetics concerns the rate at which chemical reactions proceed. Understanding the factors influencing reaction speed can profoundly affect how scientists design experiments and industrial processes. The rate of a chemical reaction can be expressed through rate laws, which relate the reaction rate to the concentrations of reactants.
Key aspects of kinetics include:
- Order of Reaction: This refers to the power to which the concentration of a reactant is raised in the rate equation. It provides insights into the reaction mechanism.
- Activation Energy: This is the minimum energy required for a reaction to occur. Lowering activation energy can lead to faster reactions and is vital in catalysis.
- Temperature: An increase in temperature generally increases reaction rates, which follows the Arrhenius equation.
Kinetic studies are invaluable for optimizing conditions in laboratory and industrial settings. These studies can also provide insights into potential pathways of a reaction, which is critical when predicting product formation.
Quantum Chemistry Applications
Quantum chemistry serves as a powerful tool in the predictive modeling of chemical reactions. It applies quantum mechanics principles to understand the behavior of electrons in atoms and molecules. By utilizing quantum calculations, researchers can gain deep insights into molecular structures, reaction pathways, and energy barriers.
Common applications include:
- Molecular Orbitals: Understanding how atomic orbitals combine to form molecular orbitals helps predict molecular stability and reactivity.
- Energy Calculations: Quantum chemistry enables accurate energy calculations for various reaction intermediates and transition states using methods like Hartree-Fock and Density Functional Theory (DFT).
- Simulations: Computational simulations can model complex reactions over time, allowing researchers to visualize dynamic processes that are otherwise difficult to observe experimentally.
The integration of quantum mechanics into chemical predictions enhances the accuracy of models and bridges theoretical and empirical knowledge, providing a comprehensive understanding of molecular interactions.
By combining thermodynamic principles, kinetic data, and quantum chemical insights, researchers can formulate a cohesive strategy for predicting chemical behavior effectively. Understanding these theoretical approaches paves the way for informed experimental practices and innovative applications in various chemical fields.
By combining thermodynamic principles, kinetic data, and quantum chemical insights, researchers can formulate a cohesive strategy for predicting chemical behavior effectively. Understanding these theoretical approaches paves the way for informed experimental practices and innovative applications in various chemical fields.
Computational Methods in Predictive Chemistry
Computational methods play a critical role in predictive chemistry, particularly because they allow scientists to model and understand complex chemical reactions without needing to conduct extensive experiments. These methodologies integrate theoretical principles with advanced computational power to simulate chemical behavior, making predictions about reaction outcomes more efficient.
One key benefit of computational methods is their ability to analyze large datasets, which is increasingly important in the realm of big data and complex systems. They help in assessing various possible pathways for reactions, providing insights that may not be evident through experimental methods alone. Furthermore, computational approaches are often more cost-effective and time-efficient compared to traditional laboratory experiments.
Molecular Modeling Techniques
Molecular modeling techniques are foundational in predictive chemistry. They involve creating computer-based representations of molecules and systems to study their behavior under different conditions. Software tools such as Gaussian and CHARMM enable researchers to simulate molecular interactions and predict reaction mechanisms with considerable accuracy.
Among the diverse methodologies, ab initio and density functional theory (DFT) are notable. These methods enable scientists to calculate the electronic structure of molecules, providing insights into how chemical bonds form and break. Through molecular dynamics simulations, researchers can explore how molecules evolve over time, revealing the dynamics of reaction processes in detail. The understanding gained through these techniques consequently enhances the accuracy of predictions made about reaction outcomes.
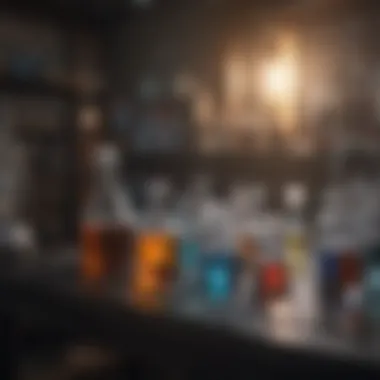
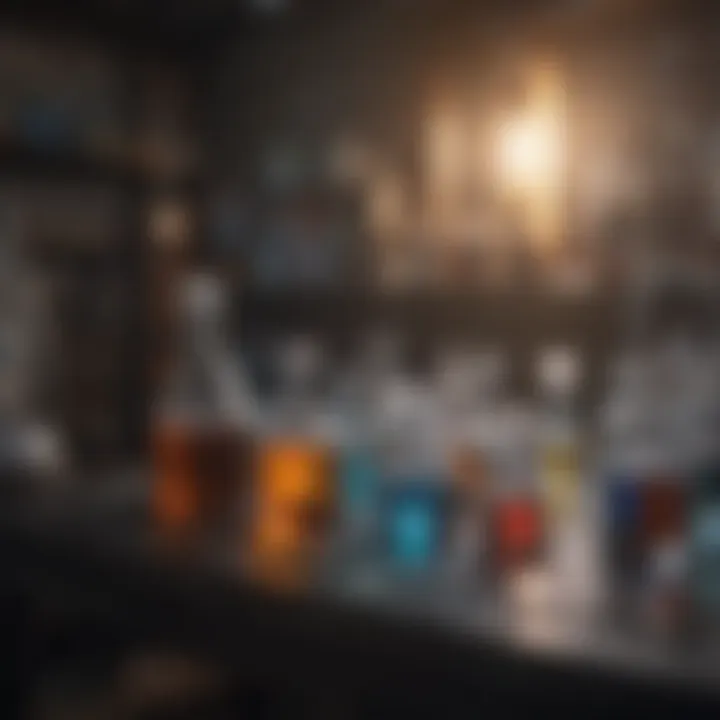
Machine Learning Applications
Machine learning applications are becoming increasingly significant in the field of predictive chemistry. Leveraging algorithms to analyze chemical data allows for improved predictions that are driven by patterns identified in large datasets. Programs like ChemProp and DeepChem illustrate how machine learning can predict molecular properties based on previous information.
One of the main advantages of using machine learning is its capacity to continuously improve predictions as new data becomes available. As the algorithms learn from more examples, they can refine their predictive capabilities, which is essential for dealing with complex reaction networks. Moreover, machine learning enables the integration of various types of data, including experimental results and theoretical calculations, creating a more holistic view of chemical behavior.
"The intersection of machine learning and chemistry is paving the way for unprecedented predictive capabilities in molecular design and reaction outcomes."
"The intersection of machine learning and chemistry is paving the way for unprecedented predictive capabilities in molecular design and reaction outcomes."
In summary, computational methods, particularly molecular modeling techniques and machine learning applications, significantly enhance our understanding of chemical reactions. They not only streamline the prediction process but also open avenues for innovative solutions in fields such as drug discovery and materials science.
Experimental Techniques for Reaction Prediction
Experimental techniques play a vital role in the predictive landscape of chemical reactions. These methods provide empirical data that enhances theoretical models and strengthens predictions about reaction mechanisms and outcomes. Researchers can observe real-time changes in a reaction, allowing for an understanding that purely computational methods may not reveal. The integration of experimental techniques with computational predictions leads to a more holistic view of chemical behavior.
High-Throughput Screening
High-throughput screening is a powerful method that enables researchers to quickly assess large libraries of chemical compounds. This technique is particularly useful in drug discovery, wherein numerous candidates need to be evaluated against specific biological targets. By automating the testing process, high-throughput screening reduces the time and resources required for developing potential new drugs.
Key benefits of high-throughput screening include:
- Efficiency: It allows for thousands of experiments to be conducted simultaneously.
- Data Richness: Generates extensive data sets, facilitating diverse analyses.
- Speed: Accelerates the identification of potential leads in pharmaceutical research.
However, there are considerations to keep in mind. The quality of the initial data is crucial for success. Misleading or inaccurate results from early screenings can lead to wasted resources in later stages. Furthermore, while high-throughput screening can reveal patterns and trends, it often requires follow-up experiments to understand the underlying mechanisms fully.
In Situ Monitoring Methods
In situ monitoring methods involve observing chemical reactions as they happen. These techniques allow scientists to gather real-time data on various parameters, such as temperature, pressure, and concentration of reactants and products. By applying methods such as spectroscopic analysis or thermogravimetry, researchers can achieve a deeper understanding of reaction kinetics and mechanisms.
The advantages of in situ monitoring include:
- Real-time insights: Provides immediate feedback on reaction progress.
- Detailed Mechanism Clarification: Helps elucidate complex reaction pathways.
- Adaptability: Can be adapted to study a wide range of reactions across different settings.
Yet, the use of in situ methods comes with challenges. Equipment sensitivity is critical, as many reactions occur under conditions that can easily lead to noise or interference in measurements. Additionally, interpreting the data requires expertise to avoid conclusions that are speculative and unsupported by evidence.
"The ability to monitor reactions in situ reflects a shift towards more nuanced understanding of chemical processes. This approach significantly shapes the future of predictive chemistry."
"The ability to monitor reactions in situ reflects a shift towards more nuanced understanding of chemical processes. This approach significantly shapes the future of predictive chemistry."
Challenges in Predicting Chemical Reactions
Predicting chemical reactions presents several challenges that need to be understood and addressed. These challenges can significantly influence the accuracy of reaction predictions, which ultimately impacts various scientific disciplines and industries. The nature of chemical reactions is inherently complex due to the numerous variables involved, including the types of reactants, environmental conditions, and pathways through which reactions can proceed. Understanding these challenges is critical for improving methodologies in predictive chemistry.
Complex Reaction Networks
In chemistry, reaction networks can be thought of as a web of interconnected reactions that evolve over time. These networks can become exceedingly complex, particularly in systems with numerous reactants and products. Each reaction path can produce a multitude of possible products, and depending on the initial conditions, some pathways might be favored over others. This complexity complicates attempts to predict outcomes based on simplified models. For example, the reaction between hydrocarbons can lead to countless possible isomers, each with distinct properties and reactivity.
Beyond just chemical structures, factors like temperature, pressure, and solvent can further influence reaction kinetics and thermodynamics. The challenge lies in modeling this web effectively, as traditional methods often fail to account for every variable and interaction involved. The result can be inaccurate predictions, leading to wasted resources in research or industrial applications.
"In reaction networks, accuracy in prediction often hinges on the integration of various parameters that influence each step of the process."
"In reaction networks, accuracy in prediction often hinges on the integration of various parameters that influence each step of the process."
Limitations of Current Models
Current predictive models face several limitations that hinder their effectiveness. Classical models, such as those based on thermodynamics, often rely on assumptions that do not hold in all situations. For instance, many traditional models assume equilibrium conditions, which may not be present in rapid or irreversible reactions. This can result in predictions that do not align with experimental results.
Moreover, models utilizing quantum chemistry can be computationally intensive and require significant resources. Though they provide detailed insights into molecular interactions, they may not be feasible for large systems or high-throughput screening.
Furthermore, existing artificial intelligence and machine learning approaches, while promising, still grapple with the need for high-quality training data. If the data is incomplete or biased, the generated models can yield misleading predictions. Thus, the advancement of predictive chemistry is often stymied by these limitations, pointing to the need for further research and improved methodologies.
In summary, the challenges associated with predicting chemical reactions are diverse and multifaceted. Addressing the complexities of reaction networks and the limitations of current models is essential for enhancing the credibility and applicability of reaction predictions across various scientific disciplines.
Practical Applications of Reaction Predictions
Predicting chemical reactions holds significant importance across various scientific domains. Each application of reaction predictions showcases the potential for enhancing processes, improving product outcomes, and mitigating adverse effects on the environment. In this section, we will explore three critical areas where these predictions are indispensable: pharmaceutical development, materials science innovations, and environmental impact assessments.
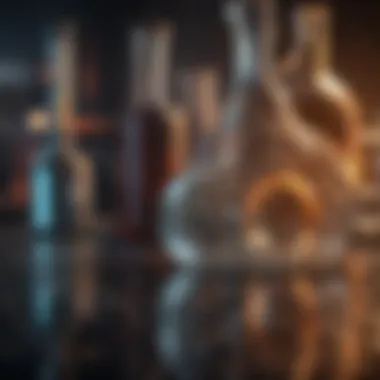
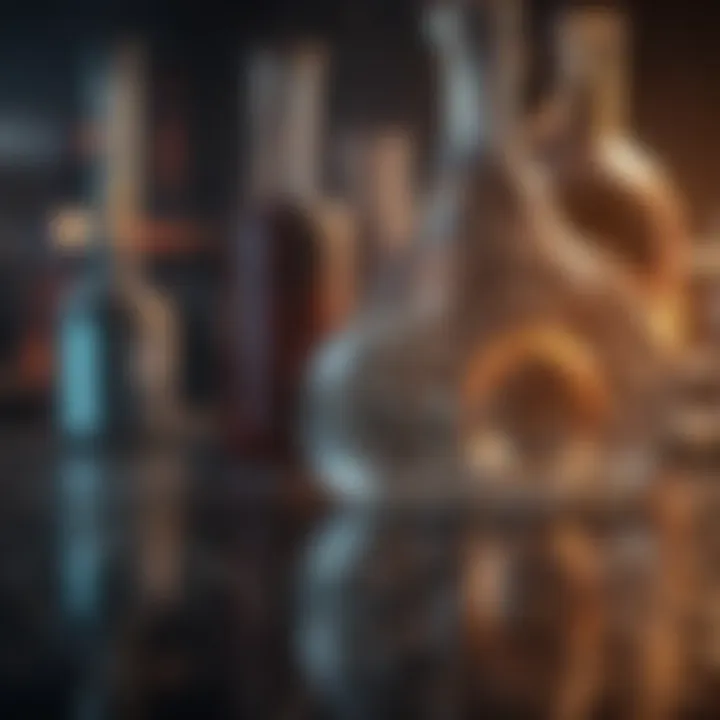
Pharmaceutical Development
In the field of pharmaceuticals, the prediction of chemical reactions plays a vital role in drug discovery and development. Understanding how different compounds will react allows researchers to efficiently synthesize potential medications.
Benefits include:
- Reduced Time and Costs: By accurately predicting which compounds will yield effective drugs, developers can streamline experiments. This reduction in trial and error not only saves time but also lowers costs associated with resource-intensive research.
- Enhanced Safety Profiles: Predictive models can help foresee adverse reactions between drugs and biological systems. Identifying potential toxicity early in the development process leads to safer drug profiles, minimizing risks associated with clinical trials.
- Targeted Drug Design: Utilizing computational methods enables the design of molecules tailored for specific biological targets. This means more effective treatments for diseases, improving patient outcomes.
Materials Science Innovations
Advancements in materials science heavily depend on the ability to predict chemical reactions. When developing new materials, understanding how substances interact enables scientists to innovate more effectively.
Key areas of impact include:
- Creating Advanced Materials: Predicting reactions with high precision aids in designing materials with desired properties, such as increased strength or enhanced conductivity. This can lead to breakthroughs in electronics, manufacturing, and nanotechnology.
- Sustainability Considerations: As awareness of environmental concerns grows, there is a push for materials that are not only effective but also environmentally friendly. Predictive models help in assessing the lifecycle of materials and their ecological impact before they are synthesized.
- Recycling and Reusability: Understanding chemical behaviors can enhance recycling processes, making it easier to recover valuable components from used products.
Environmental Impact Assessments
Reaction predictions extend beyond laboratories and into environmental contexts. Accurate predictions of chemical behavior help assess the impact of various compounds on ecosystems.
Considerations include:
- Pollution Risk Assessment: Predictive chemistry helps to forecast the potential hazardous outcomes of chemical releases into the environment. This allows for proactive measures to be taken to prevent adverse effects.
- Ecotoxicology Studies: Understanding how different chemicals interact with biological organisms is essential for evaluating toxicity levels. Predictive models afford critical insights into the potential impact of chemicals on wildlife and natural resources.
- Regulatory Frameworks: Data gleaned from predictive models supports regulatory agencies in decision-making. Ensuring that new chemical substances meet safety standards hinges upon accurate predictions of their behaviors.
In summary, the practical applications of reaction predictions span multiple fields, benefiting society by fostering innovation while prioritizing safety and sustainability. Commitment to refining these methodologies is crucial for future advancements.
In summary, the practical applications of reaction predictions span multiple fields, benefiting society by fostering innovation while prioritizing safety and sustainability. Commitment to refining these methodologies is crucial for future advancements.
Through careful development in these domains, we can look forward to a future where predictive chemistry significantly contributes to science and industry.
Future Directions in Predictive Chemistry
The landscape of predictive chemistry is undergoing profound transformations, fueled by advancements in computational techniques and experimental methodologies. As we look ahead, it becomes crucial to explore future directions that will shape this field. These directions not only reflect current trends but also highlight significant opportunities for enhancing the accuracy and applicability of reaction predictions across various domains.
Integrating AI with Chemistry
The integration of artificial intelligence in chemistry marks a pivotal advancement in predictive methodologies. This union brings various benefits, primarily the capacity to analyze vast datasets quickly and draw insights that traditionally would take researchers much longer to uncover.
AI techniques, such as machine learning and neural networks, can be harnessed to develop predictive models that can outperform classical approaches. For instance, the use of deep learning has shown promise in predicting molecular properties and reactivity patterns with greater precision. By continuously learning from new data, these models can adapt and improve, making them invaluable in dynamic research settings.
Key considerations for integrating AI into chemistry include the need for high-quality data and transparent algorithms. Without reliable data, the models may yield inaccurate predictions, leading to erroneous conclusions. Moreover, understanding how these models make decisions is essential to foster trust among chemists.
Enhancing Computational Models
Future advancements in computational models will focus on refining the methodologies that predict chemical reactions. The enhancement of models entails leveraging more sophisticated algorithms that can process complex data structures. More specifically, the development of hybrid models that amalgamate quantum mechanical principles with machine learning approaches may provide notable improvements.
These hybrid systems allow for a more comprehensive understanding of molecular interactions, as they can account for the nuances that traditional models might overlook. Moreover, increasing computational power will facilitate the simulation of more complex systems, enabling researchers to predict reactions that occur in environments closer to real-world conditions.
The implications of enhanced computational models are vast. They can hasten drug discovery, optimize material properties, and simulate environmental reactions with higher fidelity. By prioritizing improvements in model accuracy and computational efficiency, the field can move closer to achieving reliable predictions for a broader range of chemical systems.
"The future of predictive chemistry lies in the successful integration of AI and advanced computational models, fostering a new era of scientific discovery."
"The future of predictive chemistry lies in the successful integration of AI and advanced computational models, fostering a new era of scientific discovery."
Ending
The conclusion of this article underscores the critical nature of predicting chemical reactions in various domains of science and technology. It encapsulates the methodologies, theoretical insights, and practical applications discussed throughout the text. A comprehensive understanding of these methodologies not only enhances our capacity to anticipate chemical behavior but also broadens the horizons for innovation in multiple fields, such as pharmaceuticals and materials science.
Summary of Key Insights
In summary, this article provides an in-depth exploration of different facets of predicting chemical reactions. The insights discussed include:
- Fundamental Principles: Chemistry's foundational concepts, including chemical bonds and reaction mechanisms, are vital in forecasting reaction outcomes.
- Theoretical Frameworks: Thermodynamics and kinetics play essential roles in shaping our predictions and understanding reaction dynamics.
- Computational and Experimental Techniques: Advanced computer modeling, machine learning, high-throughput screening, and in situ monitoring are revolutionizing how reactions are predicted and studied.
- Emerging Challenges: The complexities of reaction networks and limitations of current models pose ongoing challenges that require attention and innovation.
"The accurate prediction of chemical reactions is not merely an academic exercise; it is vital for advancing numerous scientific fields."
"The accurate prediction of chemical reactions is not merely an academic exercise; it is vital for advancing numerous scientific fields."
Implications for Future Research
The exploration of predictive chemistry has significant implications for future research. Key areas to consider include:
- Integration of Artificial Intelligence: The potential to enhance predictive accuracy through machine learning and AI models will be transformative. This integration offers a pathway to deciphering complex chemical interactions more effectively.
- Refinement of Computational Models: There is a continuous need to refine existing models. Research should focus on improving the accuracy and reliability of predictions to accommodate increasingly complex systems.
- Cross-Disciplinary Approaches: Future studies should embrace interdisciplinary collaborations to merge concepts from physics, computer science, and biology, leading to richer predictive frameworks.