Top AI Systems: In-Depth Analysis and Future Insights
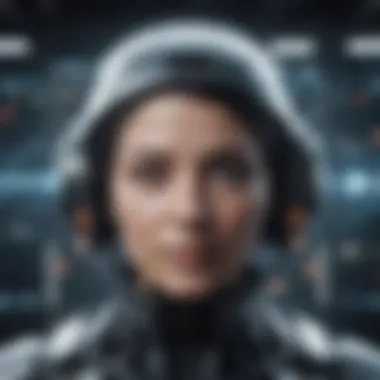
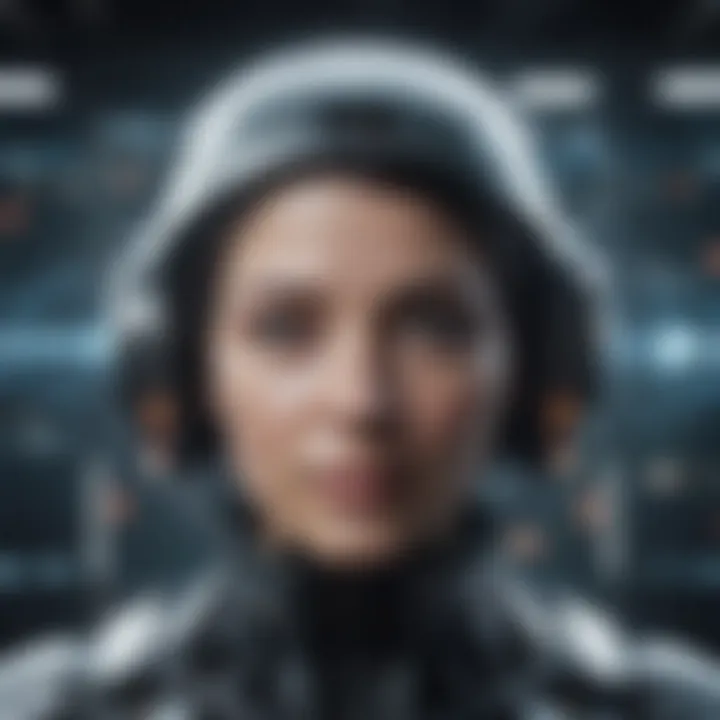
Intro
The rapidly evolving domain of artificial intelligence has become a focal point for various sectors, from healthcare to finance and beyond. As we stand on the brink of an intelligent revolution, understanding the best AI systems currently available is not just beneficial; it’s essential. This analysis embarks on a journey through the intricate world of AI, scrutinizing different systems and their implications on our society.
We live in a time when the interplay between technology and human capability is increasingly profound. Knowing what constitutes an effective AI system is critical for anyone—whether you're a student diving into this field, a researcher seeking to contribute novel insights, or a professional striving to leverage AI in their work. Furthermore, an informed perspective on the ethical dimensions and potential impacts of these technologies aids in constructive dialogues about their future trajectory.
Thus, this article will dissect the very fabric of artificial intelligence, highlighting its core principles while addressing the current trends, ethical considerations, and anticipated developments. The objective is to furnish readers with a thorough understanding, guiding them in navigating this complex yet exhilarating landscape.
Prolusion to Artificial Intelligence
Artificial Intelligence (AI) is a multifaceted field that continues to transform how we interact with technology and one another. Understanding the foundational aspects of AI is crucial, especially as it increasingly integrates into our daily lives. From personal assistants on smartphones to sophisticated systems driving autonomous vehicles, AI technologies are becoming an integral part of various sectors.
The exploration of AI highlights its vast potential but also uncovers the challenges it brings. One major consideration is how AI can sometimes make decisions that feel opaque to users; thus, digging into this aspect is vital for a deeper comprehension of AI systems. This article concentrates on a comprehensive examination of AI, unraveling the different types and systems it comprises, and what makes some solutions more impactful than others.
Moreover, as we dissect AI systems, it becomes evident that several underlying principles define their evolution, application, and limitation. The significance of AI carries far beyond mere curiosity; it raises profound questions about ethics, privacy, and reliability in technology. Navigating these waters can provide insights that are beneficial for educators, students, researchers, and professionals alike.
Defining Artificial Intelligence
At its core, Artificial Intelligence refers to the ability of machines to perform tasks that would normally require human intelligence. These tasks can include reasoning, learning, problem solving, perception, and even communication. Rather than merely executing pre-programmed instructions, AI systems analyze data, adapt to new information, and optimize their processes. A common example is how recommendation systems on platforms like Netflix or Amazon not only suggest products based on past behavior but continually refine their approach based on collective user interactions.
"AI is not about replacing humans; it's about augmenting our capabilities to make better decisions."
"AI is not about replacing humans; it's about augmenting our capabilities to make better decisions."
The definition of AI often encompasses various subfields such as machine learning and natural language processing, which further illustrate its capabilities. Machine learning, for instance, allows computers to learn from data without explicit programming, thus enabling them to adapt and improve automatically. This flexibility is a significant benefit, but it also brings concerns regarding bias, as algorithms may unintentionally replicate human prejudices embedded in training data.
The Evolution of AI Technologies
The journey of AI technologies can resemble a rollercoaster ride, with its share of highs and lows since the inception of the term in the mid-20th century. Initially, early systems were limited in scope, often requiring obsolete rules and rigid parameters. Over the decades, we witnessed breakthroughs, such as the development of expert systems in the 1980s and advancements in neural networks as we approached the new millennium.
One notable change in recent years is the advent of deep learning. With powerful algorithms capable of performing complex data analysis, deep learning has paved the way for innovations like image and speech recognition. For instance, the recognition software in smartphones has vastly improved due to these technologies. Alongside this, the availability of large datasets and enhanced computational power has fostered further breakthroughs, propelling AI into various sectors including healthcare, finance, and even creative industries.
In summary, the evolution of AI reflects an ongoing dance of innovation and adaptation. As models and technologies mature, our ability to integrate AI into everyday challenges becomes ever more sophisticated, promising exciting prospects for the future.
Criteria for Evaluating AI Systems
To discern the effectiveness of various artificial intelligence systems, one must dive deep into a detailed set of criteria. The landscape of AI is vast and varied, encompassing everything from simple algorithms to complex neural networks. Each system brings its own strengths and weaknesses, which can significantly influence its output and applicability in real-world scenarios. Thus, a well-defined framework for evaluation is crucial. This framework not only helps in identifying the best systems but also in understanding how these systems can fit into existing processes or innovate new ones.
Performance Metrics
Performance metrics form the backbone of any AI evaluation. They are the numerical outputs or criteria that indicate how well an AI system performs its designated tasks. These metrics can vary drastically based on the type of AI system and its intended use.
- Accuracy: This is often the first metric that comes to mind. It simply measures the proportion of correct predictions made by the model compared to the actual outcomes. While a high accuracy is desirable, it should be viewed alongside other metrics to paint a complete picture.
- Precision and Recall: Particularly important in cases where the quality of results matters more than quantity. Precision measures the number of true positive results divided by the number of all positive predictions. Recall, on the other hand, gauges how many actual positive cases were captured.
- F1 Score: A harmonic mean of precision and recall, the F1 Score provides a single metric that balances both considerations and is especially useful in imbalanced class distributions.
Using these metrics collaboratively offers a nuanced insight into how well an AI system is performing under real-world conditions. Each metric can reveal different limitations and strengths, leading to informed decisions about modifications or applications.
Scalability and Flexibility
In the world of AI, scalability refers to the capability of a system to handle increasing amounts of work or its potential to be enlarged to accommodate that growth. The technology landscape is continuously changing, and businesses must adapt swiftly. An inflexible model could mean losing out on opportunities or failing to meet evolving demands.
- Adaptability: The best AI systems are built to adapt, evolving with both user needs and advancements in technology. This keeps them relevant over time. For instance, models that can learn from new data without extensive retraining are considered highly flexible.
- Deployment Across Environments: Various systems might need to be utilized in different settings, from cloud to on-premises. A system that can seamlessly transition between these environments will greatly benefit organizations.
- Resource Management: Efficient use of computational resources is vital. An AI model that requires fewer resources while still delivering top-notch performance is ideal for companies looking to optimize costs.
By ensuring scalability and flexibility, stakeholders guarantee that their AI investments can grow and adapt in line with business needs and technological advancements.
User Experience
User experience plays a critical role in determining the success of an AI system. An innovative model may boast state-of-the-art capabilities, but if the end-user finds it challenging to interact with or comprehend, its potential can be severely hamstrung.
- Interface Design: An intuitive interface can significantly enhance user interaction. Whether it’s a chatbot handling customer inquiries or a diagnostic tool for healthcare professionals, ease of use can determine whether users adopt the technology.
- Feedback Mechanism: Collecting user feedback and incorporating it into the system can help refine performance and usability. Systems should have mechanisms in place to learn from user interactions continually.
- Training and Support: For many AI systems, especially more complex ones, user education is essential. Providing adequate training resources and responsive support can greatly influence user confidence and satisfaction.
Types of AI Systems
Understanding the various types of AI systems is crucial for grasping the landscape of artificial intelligence and its applications. Each type brings unique characteristics that cater to different needs and challenges in society. This exploration sheds light on how these differences can influence effectiveness in real-world applications, ultimately shaping our future.
Machine Learning Models
Machine learning is at the heart of modern AI, transforming how systems learn from data. These models can be broadly categorized into three primary types. Each has its own strengths and shortcomings, ultimately guiding their applications across various industries.
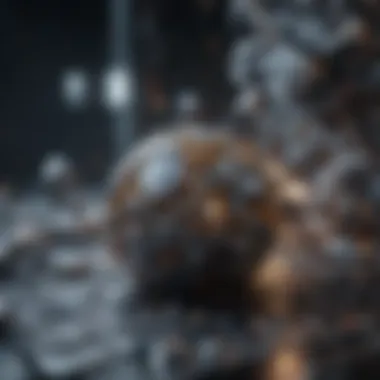
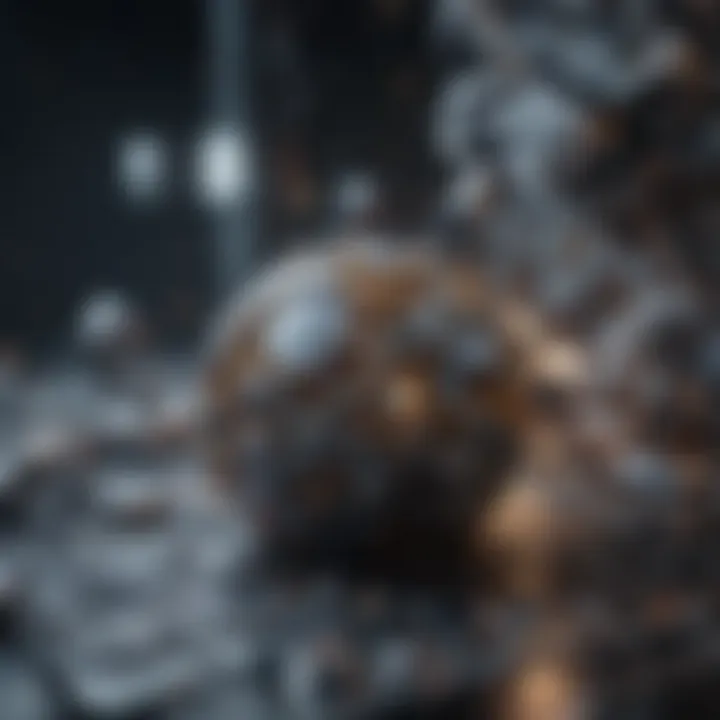
Supervised Learning
Supervised Learning is one of the most widely used machine learning techniques. It deals with learning from a labeled dataset, where the model is trained on input-output pairs. The key characteristic here is the reliance on labels, which guide the learning process.
This approach is beneficial because it allows the model to make accurate predictions based on past data, thus enjoying a strong track record in various applications like fraud detection and image classification. One unique aspect of Supervised Learning is its ability to quantify performance through metrics like accuracy and precision. However, it can suffer from overfitting, where the model performs well on training data but poorly on unseen data.
Unsupervised Learning
Contrastingly, Unsupervised Learning does not utilize labeled data. Instead, it looks for patterns and structures within the data itself. A key feature of this method is its exploratory nature, making it a valuable tool for discovering hidden insights.
This type of learning is often considered ideal for tasks such as customer segmentation or anomaly detection, where the goal is to identify natural groupings. Its uniqueness lies in the ability to learn from unlabeled data, potentially uncovering information that might be missed in supervised frameworks. However, its downside is the challenge of validating the model, as no clear answers exist to determine its success.
Reinforcement Learning
Next up is Reinforcement Learning, a paradigm where an agent learns to make decisions through trial and error. The model interacts with an environment and receives feedback in the form of rewards or penalties. The key characteristic of this approach is its focus on action sequences, not just static inputs.
What makes Reinforcement Learning particularly appealing is its capability to handle complex decision-making tasks, such as training autonomous vehicles or playing games like chess and Go. The unique aspect here is the learning process, which mimics natural learning through experiences—good decisions lead to rewards while poor ones result in penalties. However, it can face challenges such as requiring significant computational resources and time to converge to optimal solutions.
Neural Networks and Deep Learning
Neural Networks form the backbone of deep learning, allowing computers to recognize patterns and make predictions. This segment focuses on two popular types of neural networks: Convolutional Neural Networks and Recurrent Neural Networks.
Convolutional Neural Networks
Convolutional Neural Networks (CNN) are particularly effective for processing grid-like data such as images. Their architecture is designed to automatically and adaptively learn spatial hierarchies of features. This capability makes them invaluable in fields such as computer vision, where understanding visual content is key.
The main advantage of CNNs is their ability to extract complex features without human intervention, allowing for higher accuracy in tasks such as image recognition and classification. However, they do require large datasets for training, which can be a barrier to entry for some applications.
Recurrent Neural Networks
On the other hand, Recurrent Neural Networks (RNN) excel in tasks where context is important, such as language processing or time-series prediction. A key characteristic of RNNs is their ability to maintain a 'memory' of previous inputs, enabling them to account for context in their predictions.
RNNs are beneficial in scenarios where temporality matters, like translating sentences or generating text. However, they can suffer from issues such as vanishing gradients, making them unstable during training. This particular trait underscores their complexity, balancing potential with challenges that can arise during use.
The exploration of these types of AI systems lays the groundwork for understanding their real-world applications and implications on society and industry.
The exploration of these types of AI systems lays the groundwork for understanding their real-world applications and implications on society and industry.
Top AI Systems in Practice
As artificial intelligence continues to permeate various sectors, understanding the best AI systems in practice becomes crucial. These systems not only demonstrate the capabilities of current technology but also set benchmarks for future developments. The significance of this section lies in highlighting how AI systems are applied in real-world scenarios, providing insights into their functionality and impact on daily life. By showcasing practical applications, this segment aims to clarify the transformative potential of AI across multiple industries.
Chatbots and Virtual Assistants
Chatbots and virtual assistants have redefined customer service and personal assistance. Companies, big and small, employ these AI systems to interact with users in a conversational manner. The functionality of a chatbot goes beyond simple queries; it can handle complex transactions, guide users through processes, and even provide personalized suggestions.
For instance, Slackbot in Slack helps users navigate through the app and answers common questions, streamlining workflow within teams. Similarly, Siri on Apple devices can perform a range of tasks from setting reminders to controlling smart home devices.
The benefits are manifold:
- Cost Efficiency: Businesses save on customer service costs by automating responses.
- 24/7 Availability: Unlike human agents, chatbots are available around the clock.
- Scalability: As demand spikes, chatbots can easily handle numerous queries.
However, one must consider limitations such as language processing errors and inability to grasp nuanced human emotions fully. It's a double-edged sword, offering efficiency while sometimes compromising personal touch.
AI in Healthcare
The healthcare sector has embraced AI like never before, enhancing diagnostics, treatment protocols, and patient management. AI systems can analyze vast amounts of medical data swiftly, allowing for more accurate patient outcomes. Consider IBM Watson Health, which leverages AI algorithms to assist doctors in making informed decisions based on patient history and clinical data.
The impact is profound:
- Early Diagnosis: AI can detect anomalies in imaging faster than many clinicians.
- Personalized Medicine: Algorithms can tailor healthcare plans based on individual genetic information.
- Operational Efficiency: Hospitals can optimize resource allocation patterns through predictive analytics.
Nonetheless, ethical considerations, such as data privacy and the reliability of AI recommendations, remain areas of concern. Balancing technological advancements with patient trust is essential for long-term success.
Autonomous Systems
Autonomous systems represent a leap towards a future with reduced human intervention. From self-driving cars to drones delivering packages, the applications of these AI systems are expanding rapidly. Waymo and Tesla are at the forefront of this technology, pushing for smarter and safer vehicles.
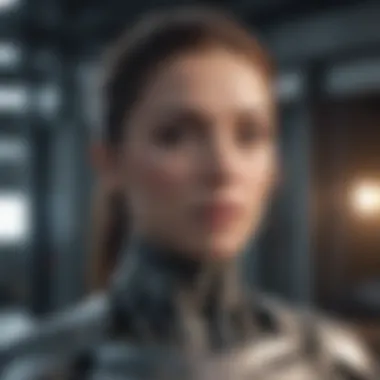
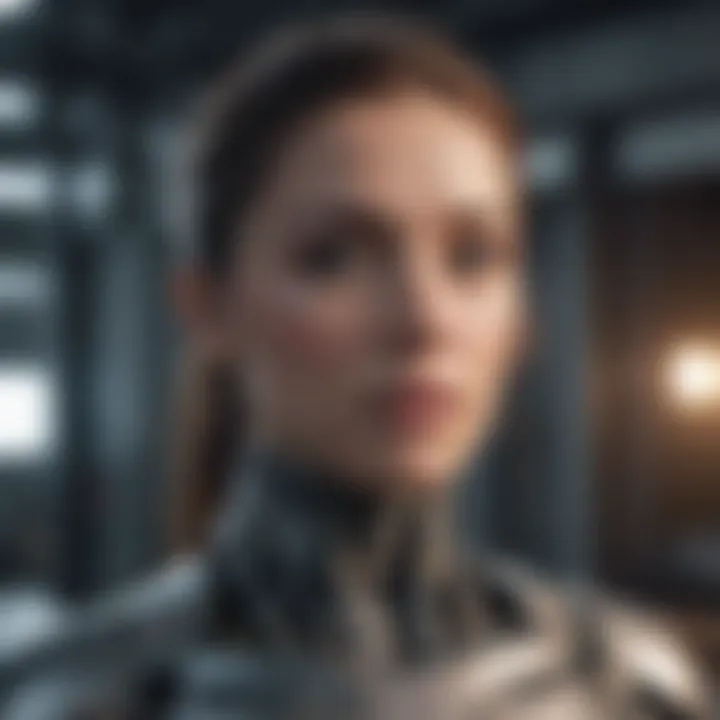
Key benefits of autonomous systems include:
- Safety Improvements: Reducing human error can significantly lower accident rates.
- Efficiency Gains: Autonomous applications can optimize routes and schedules.
However, the road ahead isn't entirely clear. Regulatory frameworks need to catch up, and societal acceptance is paramount. Concerns about job displacement and operational failures are valid and warrant careful consideration as we progress.
Applications in Finance
The finance industry has seen a remarkable transformation due to AI adoption. Banks and financial institutions use AI systems to analyze market trends, assess risks, and automate trading. Companies like Goldman Sachs employ AI-driven systems for algorithmic trading, where decisions are made within milliseconds.
The benefits here include:
- Fraud Detection: AI can learn to identify unusual transaction patterns, greatly improving security.
- Risk Management: Machine learning models can evaluate potential risks better and faster than traditional methods.
- Enhanced Customer Experiences: Personalized investment advice is increasingly driven by AI systems that analyze client portfolios.
As with other sectors, challenges exist, especially regarding regulatory compliance and data integrity. The balance between innovation and secure practices is essential to maintain trust in financial systems.
"The future of AI will unfold against a backdrop of ethical challenges and societal expectations that must be managed carefully."
"The future of AI will unfold against a backdrop of ethical challenges and societal expectations that must be managed carefully."
With each application of AI systems, we unveil not only their potential but also the considerations and challenges that come with it. Understanding these applications is vital for students, researchers, educators, and professionals who aim to navigate the rapidly evolving field of AI.
Comparative Analysis of Leading AI Models
The world of artificial intelligence is bustling with innovations, and analyzing the leading AI models is an essential task to grasp where the technology is headed. Comparative analysis not only sheds light on the strengths and weaknesses of these models but also their applicability across various fields. This section will highlight the core models in AI, namely OpenAI's GPT and Google's BERT, by examining their unique capabilities and the contexts in which they excel. We need to understand that no model is one-size-fits-all; each has its quirks that make it suited for specific applications.
OpenAI's GPT Models
Strengths and Limitations
OpenAI's GPT models are renowned for their impressive language generation capabilities. One of their standout strengths is their ability to produce coherent and contextually relevant text. This is largely due to the models' large training datasets and sophisticated architecture. Users are often amazed by its conversational abilities, making it a popular choice for applications requiring human-like interactions.
However, these models aren’t without limitations. For instance, they can sometimes generate content that is factually incorrect or mislabeled. This inconsistency raises concerns about reliability in critical areas such as healthcare or legal advice. Users must tread carefully and consider the implications of relying solely on a model that might occasionally miss the mark.
Real-World Applications
The real-world applications of OpenAI's GPT models are extensive. From customer service chatbots to complex content creation, the model’s versatility allows for wide adoption across industries. One of the key characteristics of GPT is its ability to handle multilingual content, making it beneficial for global companies seeking to engage diverse markets.
On the downside, the computational resources required to fine-tune and deploy these models can often be a barrier to entry for smaller organizations. Still, their impact on automating repetitive tasks frees up human talent for more complex decision-making processes.
Google's BERT and Transformer Models
Natural Language Processing Capabilities
Google's BERT (Bidirectional Encoder Representations from Transformers) has revolutionized natural language processing. Its key strength lies in its ability to understand context better than its predecessors by considering the full context of a word based on surrounding words. This means it can interpret and generate language that aligns more closely with human understanding.
This enhanced understanding makes BERT a strong candidate for applications in search engines, where context is everything. However, the model's reliance on extensive text input can lead to challenges in terms of speed, particularly in applications where time is of the essence.
Impact on Search Engines
In the realm of search engines, BERT's deployment has demonstrated significant improvements in query interpretation, helping users to find more relevant results. This presents a transformative element in how individuals search for information online. The model's robustness applies particularly well in processing nuanced queries — something traditional keyword-based searches struggle with.
However, it's important to note that such a shift also requires businesses to adapt their SEO strategies. As BERT alters the landscape of search engine algorithms, it necessitates content creators to pivot and focus on more holistic, user-oriented writing that reflects natural language use.
"The continual adaptation of AI models must align with ethical considerations and user needs, ensuring technologies evolve with responsibility in mind."
"The continual adaptation of AI models must align with ethical considerations and user needs, ensuring technologies evolve with responsibility in mind."
Ethical Considerations in AI Development
Understanding the ethical considerations in AI development is critical, especially as these systems gain increasing influence over societal functions. The implications of these technologies do not just affect businesses and efficiency; they touch on fundamental human rights and societal norms. As AI applications proliferate across sectors such as healthcare, finance, and transportation, the ethical framework guiding their development must ensure not just optimized performance but also the welfare of individuals and communities.
Addressing ethical concerns in AI is like laying down the tracks before the train rolls out of the station. If we get it wrong, the potential for harm is substantial. These considerations help steer the conversation towards fairness, accountability, and transparency in AI models. What’s at stake here is not merely the functionality of these systems but also public trust in them. Ethical AI promotes a balance between innovation and responsibility, allowing for advancements while safeguarding the interests of all stakeholders involved.
"An ethical approach to AI is not just a nice-to-have; it's a necessity for any sustainable integration into society."
"An ethical approach to AI is not just a nice-to-have; it's a necessity for any sustainable integration into society."
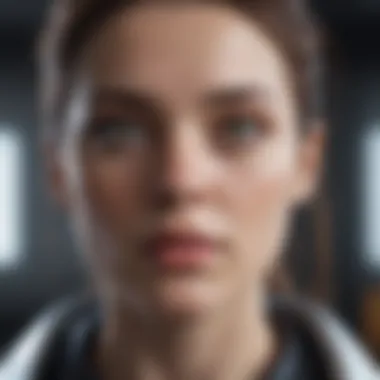
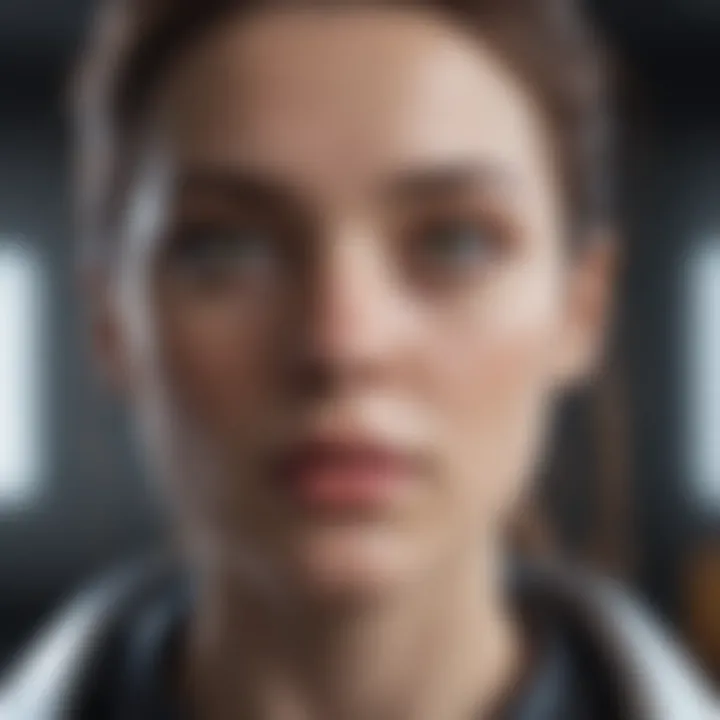
Bias and Fairness in AI Systems
Bias in AI systems can manifest in many forms, often influenced by the data used to train these systems. Models trained on unrepresentative datasets might lead to outcomes that reflect existing prejudices, resulting in significant real-world consequences. A classic example can be found within facial recognition technology, where numerous studies have shown a higher error rate when identifying individuals from certain demographics. This can lead to unfair treatment, discrimination, and erosion of trust.
Developing fair AI systems calls for systematic approaches that involve diverse datasets, constant evaluation, and modification of algorithms. Moreover, incorporating fairness into AI requires a broad definition that not only considers statistical measures but also takes into account social implications. Initiatives like the Fairness, Accountability, and Transparency (FAT) in Machine Learning conference aim to address these challenges head-on.
Key aspects of ensuring fairness include:
- Acknowledging biases in data
- Ensuring diverse representation in training sets
- Regular audits of AI algorithms for bias detection
- Implementing fairness frameworks in development processes
Privacy and Data Security
Privacy and data security are intertwined with the ethical usage of AI. With AI systems accessing vast amounts of personal information, safeguarding this data becomes paramount. Users often remain unaware of how their data is collected, processed, and stored, raising significant ethical questions. A breach of this data not only violates privacy but can also lead to severe financial and emotional repercussions.
Regulations such as GDPR in Europe emphasize the importance of transparency in data handling, allowing users to know what to expect in terms of data use. However, adhering to regulations is just one part of responsible AI practice. Organizations need to implement robust security measures, conduct regular audits, and ensure proper encryption of sensitive data.
Future Trends in AI Technology
The realm of artificial intelligence is constantly shifting and evolving, making it imperative to keep a keen eye on the upcoming trends that may redefine its landscape. Understanding these trends is crucial for both practitioners and policymakers, as they carry implications that can shape industries and influence daily life. Here, we explore the potential benefits and challenges that may arise as AI technology continues to integrate deeper into various sectors.
The Role of AI in Society
AI systems are rapidly embedding themselves into the fabric of society, transforming how people interact with technology and each other. The impacts are vast, ranging from improving productivity in workplaces to enhancing customer experiences in shopping and healthcare. For example, consider the way algorithms personalize user experiences across platforms, suggesting products tailored to individual preferences. This custom touch can ultimately lead to higher satisfaction and engagement.
Moreover, AI is increasingly seen as a tool for social good, helping to address pressing global issues like climate change, public health crises, and education gaps. Nevertheless, the societal integration of AI isn't without challenges. The risk of dependence on technology raises questions about job displacement and the loss of human touch in crucial services. Therefore, balancing benefits with ethical considerations and potential drawbacks is vital for sustained integration.
Integrating AI with Other Technologies
The future of AI is not standalone; it intertwines with emerging technologies to create even more powerful applications. Distinguished integrations include AI with the Internet of Things and quantum computing, showcasing how collaborative advancements can reshape industries.
AI and Internet of Things
One of the most transformative integrations is definitely that of AI with the Internet of Things (IoT). The combination allows smart devices to communicate and analyze data autonomously, leading to improved decision-making processes. For instance, in smart homes, AI can learn residents’ patterns and preferences, optimizing energy consumption without manual input.
The key characteristic of AI in IoT is its ability to process vast amounts of data in real-time. This is beneficial since it enables quick, informed actions based on current conditions. A unique feature of combining AI with IoT is predictive maintenance. This technology minimizes operational downtime by anticipating equipment failures before they occur.
Yet, while the advantages abound, integrating AI with IoT raises concerns about data security. Devices connected online can become susceptible to cyberattacks, making privacy a legitimate issue of contention in AI discussions.
AI in Quantum Computing
Meanwhile, the introduction of AI into quantum computing presents a revolutionary approach to data processing. Quantum computers can handle computations at speeds unattainable by classical computers, giving rise to profound implications for machine learning and data analysis. The noteworthy aspect is the ability to solve complex problems, such as drug discovery or financial modelling, significantly faster.
AI in quantum computing stands out for its potential to unlock solutions for problems currently beyond reach. For example, optimizing logistics on a large scale could lead to reductions in both time and expenses, thus benefiting industries like transportation and supply chain management. Yet, despite its potential, there are hurdles. The technology is still in its infancy, and practical applications are limited.
Ending
As AI continues to evolve and integrate with other technologies, the trajectory it takes will be dictated by innovation, ethical considerations, and awareness of societal impacts. Awareness and adaptability will be key to harnessing these trends effectively.
In summary, engaging with future trends in AI technology isn't just about understanding advancements; it's also about exploring how these trends may redefine our world and our daily interactions with technology.
In summary, engaging with future trends in AI technology isn't just about understanding advancements; it's also about exploring how these trends may redefine our world and our daily interactions with technology.
Culmination
The conclusion of this analysis serves a pivotal role in synthesizing the myriad insights drawn from the exploration of the best AI systems available today. Here, we wrap up the intricate threads of discussion surrounding the capabilities and implications of these technologies, ensuring that readers grasp not only the concepts but their far-reaching consequences.
Summary of Insights
The exploration has highlighted several significant findings:
- Diverse Applications: AI is not a one-trick pony. From chatbots aiding customer support to autonomous vehicles revolutionizing transportation, the systems discussed exhibit remarkable versatility across various sectors. This broad scope showcases how integrated AI technologies can enhance efficiency and drive innovation.
- Ethical Nuances: With great power comes great responsibility. The article has delved deeply into the ethical considerations surrounding AI. Issues of bias, data security, and privacy are deliberated, stressing the need for responsible AI development practices that prioritize fairness and transparency.
- Future Directions: The future trends we've examined underscore the inexorable march of technology.
"AI has the capability to reshape industries, but that power must be matched with integrity and foresight."
"AI has the capability to reshape industries, but that power must be matched with integrity and foresight."
Implications for Future Research
Looking ahead, several implications emerge from our analysis that can guide future research endeavors:
- Interdisciplinary Approaches: AI technology doesn’t exist in a vacuum. As we further our understanding, interdisciplinary approaches that meld computer science with social sciences will be crucial. This perspective will allow for a more holistic analysis of AI impacts on society.
- Focus on Ethics: Given the complexities of integrating AI into society, ongoing research into ethical frameworks is essential. This includes developing guidelines for fairness and accountability that can adapt to emerging technologies.
- Advancements in Algorithms: Continuous improvement in algorithmic efficiency and transparency paves the way for more robust AI systems. Researchers should investigate novel methodologies that enhance the interpretability of AI models, which remains a significant challenge in the field.
In sum, while the current capabilities of AI systems are impressive, recognizing their limitations and ethical implications is equally vital. The discourse on AI is only just beginning, and future inquiries into these cutting-edge systems promise not only to deepen our understanding but also refine their practical applications in a just and equitable manner.