Exploring Medical AI: Course Overview and Impact
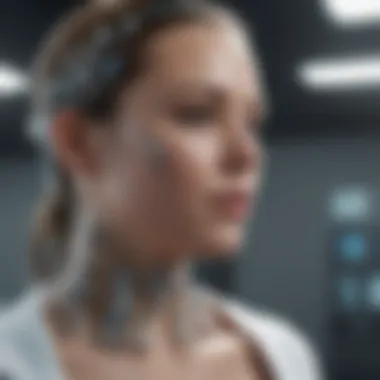
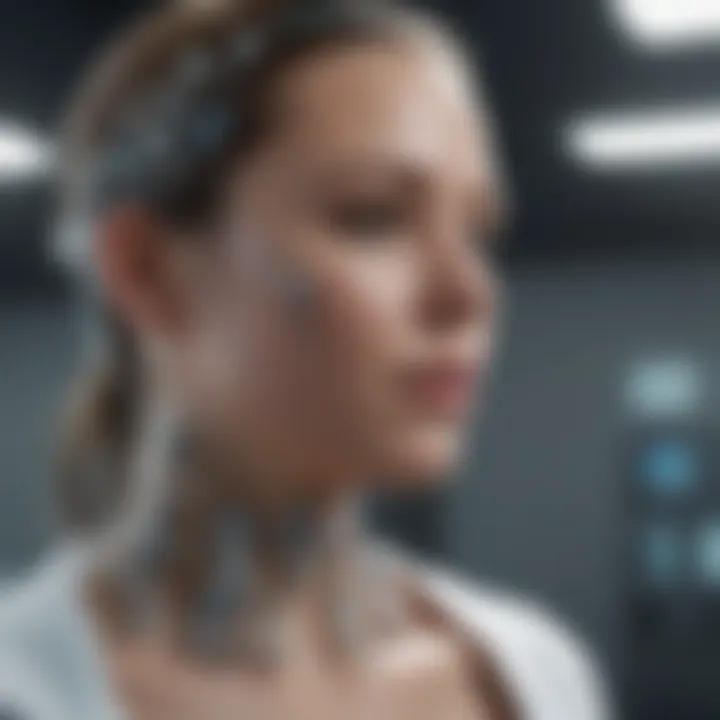
Intro
In today's evolving healthcare landscape, the integration of medical artificial intelligence (AI) is more crucial than ever. As technology progresses, so too does the need for professionals who understand how to harness AI in medical contexts. This article is designed to provide a comprehensive overview of the medical AI educational framework, examining the course structure, content, and relevance in the health sector.
Understanding medical AI requires grasping key concepts and staying up-to-date with current research trends. As we delve into this subject, we will explore definitions, scientific principles, recent studies, and significant breakthroughs. This structured overview enables students, researchers, educators, and professionals to appreciate the expansive role that education plays in fostering innovation and improving healthcare delivery.
The notion of AI in healthcare encompasses a variety of tools and methodologies. From machine learning algorithms analyzing large sets of patient data to natural language processing systems assisting in patient interaction, the potential applications are vast. By analyzing these aspects, we hope to illuminate the value of focused education in this niche field.
Prologue to Medical Artificial Intelligence
Medical artificial intelligence (AI) sits at the forefront of modern healthcare innovation. Understanding this technology is critical for professionals engaged in various aspects of medicine, from practitioners to healthcare administrators. The emergence of AI has reshaped the way healthcare can be delivered, highlighting the necessity of integrating technology into medical practices. This section examines the fundamental aspects of medical AI and its significance in improving patient outcomes and healthcare efficiency.
Defining Medical Artificial Intelligence
Medical artificial intelligence refers to the application of AI techniques and algorithms to assist in diagnosing, analyzing, and treating medical conditions. This domain of AI encompasses a range of subfields, including machine learning, natural language processing, and computer vision. By processing vast amounts of data, medical AI can offer valuable insights that enhance clinical decision-making. The definition remains broad, as the technology is continually evolving, but its core lies in making healthcare smarter and more responsive to patient needs.
The main components of medical AI can include:
- Clinical Decision Support Systems: Tools that help clinicians make better decisions by providing data-driven insights.
- Medical Imaging Analysis: Use of AI to analyze radiology images for faster and more accurate diagnosis.
- Predictive Analytics: Applications that forecast patient outcomes based on historical data, aiding in preventive care strategies.
Understanding these components helps delineate what medical AI can achieve.
The Importance of AI in Healthcare
The integration of AI into healthcare brings considerable benefits. Most notably, AI enhances the ability to provide personalized treatment options based on individual patient data. This development is essential, as each patient may respond differently to treatment. AI's capacity to analyze data from diverse sources allows practitioners to tailor interventions specifically to each individual.
Furthermore, AI can significantly reduce the time necessary for diagnoses by swiftly processing imaging results or lab tests. This efficiency not only improves patient care but also alleviates some burdens on healthcare professionals, enabling them to focus on direct patient interaction and complex decision-making.
To summarize, the importance of AI in healthcare is underscored by its potential to:
- Improve diagnostic accuracy and speed.
- Facilitate personalized medicine.
- Optimize operational efficiencies within healthcare facilities.
Course Structure and Curriculum
The structure and curriculum of medical artificial intelligence courses are fundamental to the overall learning experience. A well-organized curriculum ensures that students cover essential topics in a logical order. It equips them with the necessary knowledge and skills to navigate the complexities of AI in healthcare. This structure typically balances theoretical understanding with practical application, promoting a comprehensive grasp of the subject.
The benefits of a well-defined curriculum include clarity in learning objectives and the ability to track student progress effectively. Each course component contributes to a cohesive understanding of AI technologies and their implications in medical settings. Moreover, the structure must remain adaptable, reflecting the rapid advancements in the field. Thus, educators have the responsibility to update content continually to include the latest research and tools.
Core Topics Covered
Core topics in medical artificial intelligence courses usually encompass a variety of fields. These can include:
- Machine Learning Algorithms: Students learn about supervised and unsupervised learning techniques. Understanding algorithms such as neural networks, decision trees, and support vector machines is crucial.
- Data Science Principles: Data cleaning, transformation, and analysis are essential skills. Students engage with datasets common in healthcare to grasp the data lifecycle from collection to decision-making.
- Healthcare Informatics: This aspect covers the application of AI in electronic health records, telemedicine, and patient management systems. Knowing how AI integrates into existing healthcare frameworks is valuable.
These core topics form the backbone of the curriculum. They encourage students to develop a multifaceted view of how AI can enhance diagnosis, treatment, and overall patient care.
Practical Applications and Projects
Practical applications in medical AI courses allow students to apply theoretical knowledge to real-world problems. Many programs incorporate project-based learning, which encourages creativity and innovation. Projects might involve:
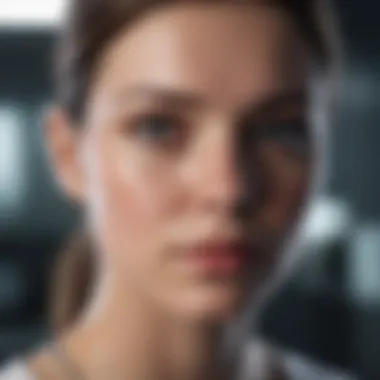
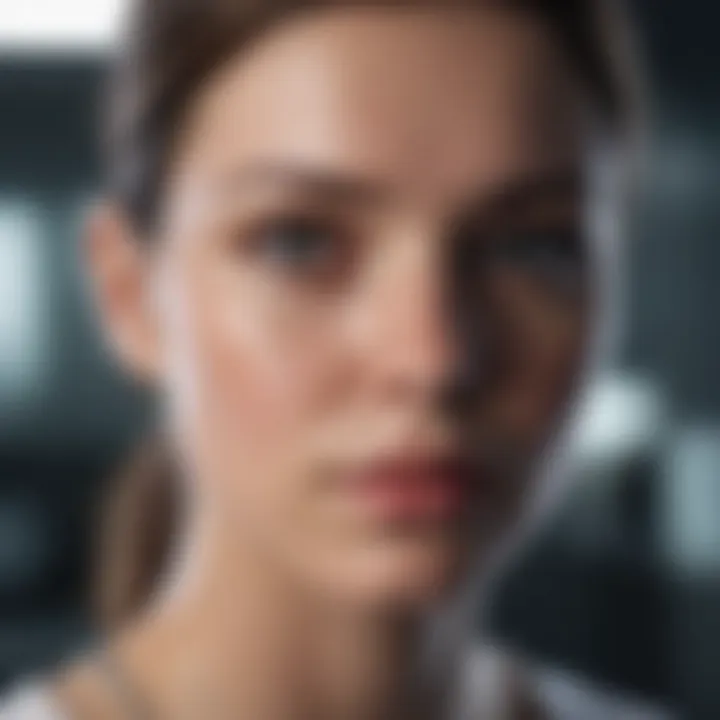
- Developing Predictive Models: Students could create models to predict patient outcomes based on historical data.
- AI-Based Diagnostic Tools: Groups might build simple algorithms that assist in interpreting diagnostic imaging or lab results.
These hands-on experiences not only enhance students’ technical skills but also foster collaboration and critical thinking.
Assessment and Evaluation Methods
Assessment in these courses encompasses a variety of methods to ensure comprehensive evaluation of student competencies. Traditional exams may gauge theoretical knowledge, while practical assessments might include:
- Project Presentations: Students showcase their projects, demonstrating understanding and integration of course material.
- Peer Reviews: Peer evaluation promotes critical thought about team dynamics and project outcomes.
Feedback is crucial in the learning process. Rigorous assessment standards help maintain course integrity and ensure that students are adequately prepared for real-world challenges.
"A well-structured curriculum not only fosters knowledge but also prepares students to face the dynamic challenges in medical AI."
"A well-structured curriculum not only fosters knowledge but also prepares students to face the dynamic challenges in medical AI."
Key Learning Outcomes
The section on key learning outcomes underscores the fundamental importance of gaining a clear understanding of what students can expect to achieve upon completing a course in medical artificial intelligence. These outcomes serve not only as guideposts for learners but also as benchmarks for educational institutions to measure the effectiveness of their curricula.
Understanding AI Technologies
In today's healthcare landscape, it is critical to grasp the underlying technologies that drive artificial intelligence. Students learn about various AI frameworks such as machine learning, neural networks, and natural language processing. This understanding enables them to analyze the capabilities and limitations of these technologies in a medical context.
AI technologies can automate tasks, enhance decision-making, and improve patient outcomes. For instance, students gain insights into systems like IBM Watson Health, which utilizes AI to sift through vast amounts of medical literature and data to assist healthcare providers in making informed clinical decisions. Such knowledge is essential for developing a future-ready mindset, which is invaluable in the continual evolution of healthcare practices.
Interpreting Healthcare Data
The ability to interpret healthcare data is another essential outcome of medical artificial intelligence education. The healthcare domain generates a vast scope of data—from patient records to medical imaging. Students are trained to analyze this data to derive meaningful insights.
Learning how to utilize statistical tools and software programs enhances their competence in evaluating data trends and making data-driven decisions. This skill is crucial for predicting disease outbreaks and understanding patient behavior, further contributing to improved patient care quality. Developing these capabilities fosters critical thinking and adaptability, necessary traits as they enter a rapidly evolving medical field.
Developing AI Solutions for Medical Problems
Finally, the course aims to equip students with the skills required to develop practical AI solutions that address real-world medical challenges. This includes designing algorithms that can predict patient outcomes or deploying chatbots to triage patient queries effectively.
Engaging in hands-on projects helps solidify students' understanding of how to create and modify AI applications relevant to healthcare settings. They learn to approach problems methodically, from identifying a clinical issue to proposing an AI-driven resolution.
"AI has the potential to transcend traditional healthcare methods, but only if the next generation of professionals is well-versed in these emerging technologies."
"AI has the potential to transcend traditional healthcare methods, but only if the next generation of professionals is well-versed in these emerging technologies."
Areas of Application in Medicine
The exploration of medical artificial intelligence intersects rich areas of application within healthcare. These applications not only enhance diagnostic processes but also refine treatment methods and improve patient outcomes. Understanding these areas is critical for students, educators, and professionals seeking to harness the power of artificial intelligence in medicine.
Diagnostic Imaging
Diagnostic imaging stands out as a prominent field leveraging AI technologies. It encompasses various imaging modalities like X-rays, MRI, and CT scans. AI algorithms improve image analysis by detecting anomalies more precisely than traditional methods. For instance, deep learning models can spot early signs of diseases such as cancer, often at stages when human detection may falter. These advancements reduce the burden on radiologists, allowing for more efficient case prioritization. However, the integration of AI requires consideration of data quality and algorithm transparency to ensure reliability in clinical decision-making.
Personalized Medicine
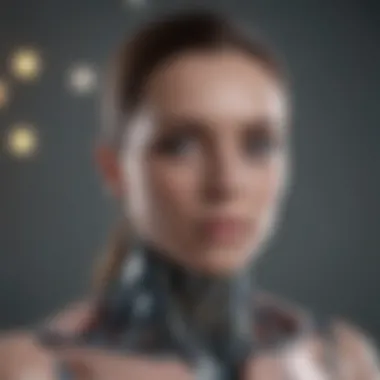
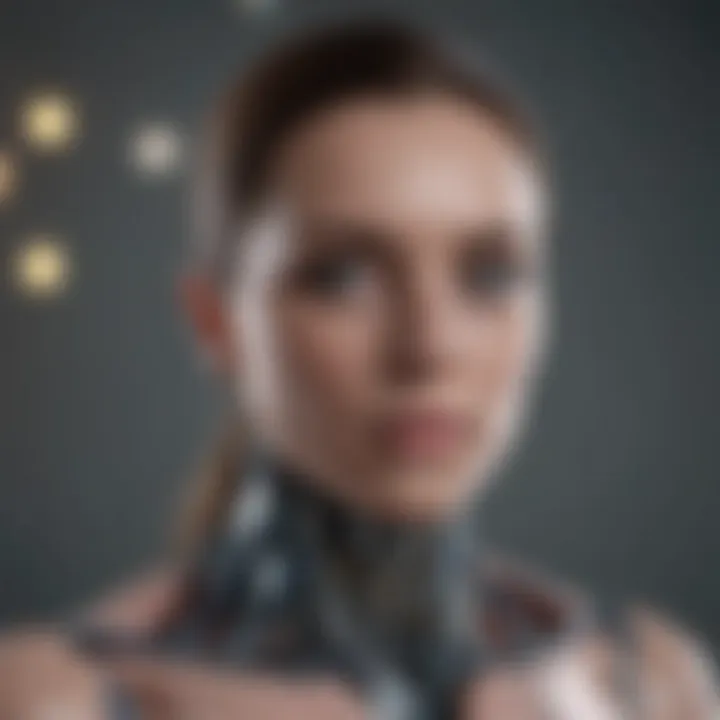
Personalized medicine aims to customize healthcare based on the individual patient characteristics, including genetics and lifestyle. AI plays a pivotal role in this area by analyzing vast datasets to identify relevant patterns. For example, machine learning algorithms can help tailor medication dosages or suggest specific treatment plans based on a patient’s unique profile. The potential benefits include improved efficacy of treatments and reduced adverse reactions. Nonetheless, ethical concerns, such as data privacy and the potential for biased algorithms, must be addressed for the safe implementation of personalized approaches.
Predictive Analytics in Patient Care
Predictive analytics harnesses AI to forecast patient outcomes and streamline healthcare services. By analyzing historical patient data, AI models can predict disease progression, readmission risks, and treatment responses. This proactive approach enables healthcare providers to intervene early, improving patient management and reducing costs. For instance, predictive analytics can identify high-risk patients for chronic conditions like diabetes or heart disease, allowing targeted preventive strategies. However, healthcare professionals must remain vigilant regarding the accuracy of predictive models and the ethical implications of using AI in patient care.
"AI technologies are transforming healthcare by providing deeper insights and improving efficacy in patient treatment strategies."
"AI technologies are transforming healthcare by providing deeper insights and improving efficacy in patient treatment strategies."
In summary, the areas of application in medical AI, including diagnostic imaging, personalized medicine, and predictive analytics, showcase the transformative potential of these technologies in healthcare. Each application presents unique benefits and challenges, reinforcing the critical need for education and ethical considerations in deploying AI solutions.
Ethical Considerations and Challenges
The integration of artificial intelligence into healthcare is not solely a matter of technological advancement. Ethical considerations and challenges must framework this progress. Questions around data privacy, algorithmic fairness, and compliance with regulatory standards occupy an essential role in the development and deployment of AI in medicine. Addressing these concerns can significantly affect how technologies are received and used by various stakeholders, including patients, healthcare providers, and policymakers.
Data Privacy and Security
Data privacy plays a critical role when it comes to patients' personal information. The healthcare sector is already under scrutiny due to sensitive data handling. Artificial intelligence implementations often require vast amounts of health data to train models effectively. Unfortunately, this data can be vulnerable to breaches, leading to severe consequences for individuals and institutions alike.
To combat this, organizations must employ encryption, secure databases, and rigorous access controls. Regular audits can also enhance security, ensuring compliance with data protection laws such as GDPR.
In the context of AI, developing systems that anonymize data can be beneficial, allowing researchers access to necessary datasets without compromising patient identities.
Bias and Fairness in AI Models
Bias is an intrinsic concern in developing AI systems. If training datasets reflect any existing disparities, AI models can inadvertently perpetuate or even exacerbate these inequities. For instance, medical AI tools trained on predominantly male populations may not perform well on female patients, leading to misdiagnoses.
To promote fairness in AI, developers need to prioritize diverse datasets that incorporate a wide range of demographic variables. Continuous evaluation of algorithms can identify areas of bias, allowing for adjustments to improve overall fairness. Efforts must also involve multidisciplinary teams that understand the healthcare landscape to ensure models serve all populations equitably.
Regulatory Considerations
Regulation in the field of AI in healthcare is still a developing area. The challenge comes from ensuring that these technologies adhere to existing medical standards while also being innovative. Regulatory bodies must navigate the delicate balance of facilitating innovation and ensuring patient safety.
For example, regulatory frameworks such as the FDA in the United States or EMA in Europe currently assess AI-based medical devices. Utilizing clear guidelines that define the scope and expectations for these products can facilitate smoother approval processes. Additionally, continuous monitoring post-deployment can help identify issues that may arise in real-world applications.
Ethical considerations are paramount to ensuring the successful integration of AI systems in healthcare, affecting both trust and clinical efficacy.
Ethical considerations are paramount to ensuring the successful integration of AI systems in healthcare, affecting both trust and clinical efficacy.
By addressing these ethical realms systematically, the responsible development of artificial intelligence in medicine can significantly improve trust and acceptance. Balancing the advantages of AI with these ethical considerations will ultimately dictate the path forward.
Case Studies Demonstrating AI in Healthcare
The examination of case studies demonstrating AI in healthcare serves as a fundamental element of this article. Such studies illustrate not only the success but also the challenges encountered when implementing artificial intelligence in clinical settings. Real-world case studies showcase practical applications and results of AI technologies in various medical fields. They provide insights into how AI can deepen our understanding of medical processes, enhance patient care, and optimize healthcare delivery within institutions.
Successful Implementations
Successful implementations of AI in healthcare highlight the transformative potential of technology in improving patient outcomes and operational efficiency. For example, the collaboration between Google Health and healthcare systems has led to the development of innovative diagnostic tools. One notable project involves the application of deep learning algorithms to detect diabetic retinopathy in ophthalmic images. The accuracy achieved in these diagnostics is comparable to that of experienced radiologists, demonstrating how AI can augment human expertise.
Another example is IBM's Watson, which has shown promise in oncology. By analyzing vast amounts of clinical data and medical literature, Watson helps oncologists identify personalized treatment plans based on individual patient characteristics. The positive outcomes in various hospitals adopting this technology reveal its potential to enhance decision-making processes.
The benefits of successful AI implementations include:
- Increased diagnostic accuracy: AI systems can analyze data rapidly and with precision.
- Improved operational efficiency: Automation of routine tasks allows healthcare professionals to focus on patient care.
- Enhanced personalized treatment: AI can tailor therapies based on genetic and clinical information.
"AI's integration into healthcare can lead to significant improvements in how we diagnose and treat diseases."
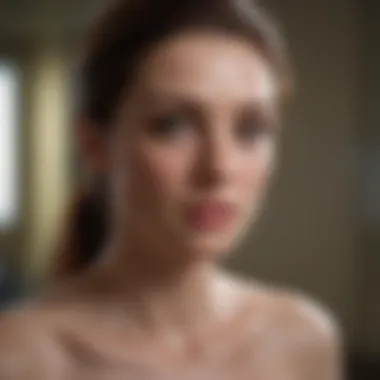
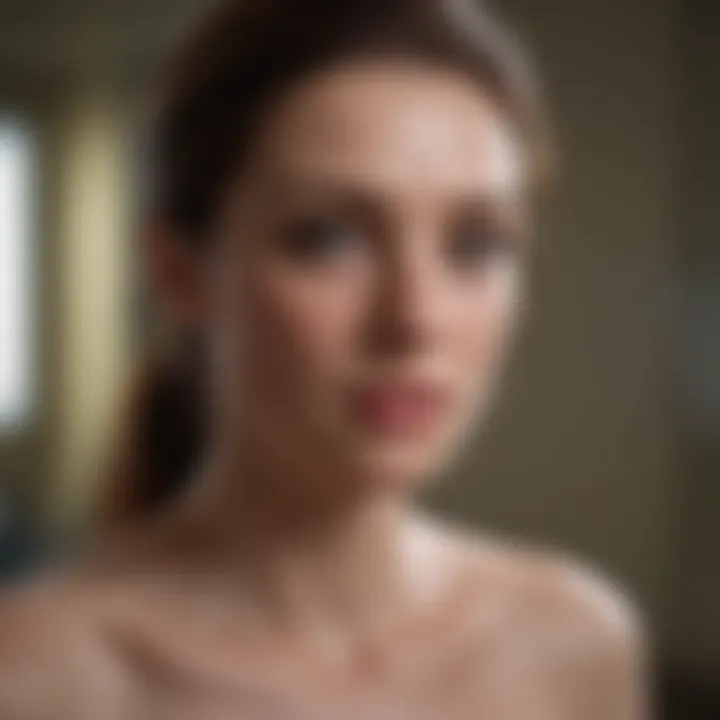
"AI's integration into healthcare can lead to significant improvements in how we diagnose and treat diseases."
Lessons Learned from Failures
While successes are often celebrated, it is equally important to examine lessons learned from failed AI projects. Such cases reveal the complexities of deploying AI in healthcare and the need for a careful approach. One significant example is the early implementation of AI-assisted chatbots in patient triage systems. Many systems faced challenges regarding accuracy and user trust, leading to misdiagnoses and frustrated patients. This highlights the necessity for thorough testing and validation before real-world deployment.
Failures can result from various factors including:
- Data quality issues: Poor quality data can lead to misleading outcomes. Having diverse and comprehensive datasets is crucial for AI training.
- Lack of clinician involvement: AI tools must fit seamlessly into the workflow of healthcare professionals, requiring their input during design and implementation phases.
- Underestimating regulatory hurdles: Navigating the landscape of healthcare regulations is complex and can impede AI project timelines.
Through reflecting on these lessons, future AI projects can be better structured to address potential pitfalls, ultimately leading to more successful outcomes in healthcare settings.
Future Trends in Medical Artificial Intelligence
The field of medical artificial intelligence (AI) continues to evolve rapidly, influencing how healthcare systems operate and deliver services. Understanding the future trends in this area is crucial for stakeholders in education, research, and practice. Not only do these trends provide insights into potential advancements, but they also highlight areas where there may be challenges and opportunities for innovation. By keeping abreast of these trends, students, researchers, and professionals can better prepare for the changes that will shape the future of healthcare.
Advancements in Machine Learning Techniques
Machine learning (ML) serves as the backbone of many medical AI applications. As technology progresses, various ML techniques are gaining traction. One significant advancement is the development of deep learning algorithms. These algorithms process vast amounts of data, allowing for better accuracy in diagnostic tasks. Improved neural networks are making it possible to analyze medical imaging with unprecedented precision.
Furthermore, transfer learning is becoming common. This technique uses pre-trained models on one dataset and applies it to another. For instance, models trained on large image datasets can be fine-tuned for specific medical imaging tasks more efficiently. The future will likely see wider adoption of these methods, leading to more personalized and precise diagnostics in various medical fields.
While these advancements are promising, they require careful implementation. There are ongoing discussions about best practices and guidelines for model validation. Accurate assessment can prevent issues that arise from overfitting or bias. Institutions should prioritize continuous evaluation of these techniques to ensure they are effective in real-world scenarios.
Integration with Telemedicine
Telemedicine is the delivery of healthcare services remotely using technology. Its rise has been particularly evident during health crises, such as the COVID-19 pandemic. Integrating medical AI with telemedicine presents significant opportunities. For example, AI-based chatbots can offer preliminary assessments to patients before they consult medical professionals. These tools can analyze symptoms and suggest possible next steps, streamlining the healthcare process.
Additionally, AI can assist in monitoring chronic conditions through remote sensors and software applications. By consistently analyzing data from wearables, healthcare providers can receive alerts about patients’ conditions and intervene as necessary. This level of proactive care can significantly enhance patient outcomes.
However, integrating AI with telemedicine also raises concerns regarding data privacy and patient consent. The ethical implications must be evaluated, and safeguarding patient information should be a top priority. Future initiatives must focus on addressing these challenges while embracing the new possibilities presented by medical AI in telemedicine.
The intersection of artificial intelligence and telemedicine reflects a critical evolution in healthcare delivery.
The intersection of artificial intelligence and telemedicine reflects a critical evolution in healthcare delivery.
End
The conclusion plays a crucial role in crystallizing the knowledge presented throughout this article. It serves as the final note that not only synthesizes the content but also emphasizes the significance of medical artificial intelligence education. The relevance of this subject is rooted in several elements.
Summarizing the Impact of Education in AI
Education in medical artificial intelligence equips individuals with the necessary skills to navigate the complexities of this evolving field. The diverse curriculum covered in courses allows students to understand AI technologies, interpret healthcare data effectively, and develop innovative solutions for medical challenges. The impact of well-designed educational programs extends beyond individual growth; it ultimately contributes to advancements in healthcare delivery and patient outcomes.
Moreover, these educational experiences underscore the importance of interdisciplinary collaboration. As AI continues to integrate into various medical sectors, a solid educational foundation fosters better coordination between technologists and healthcare providers. This synergy is vital for implementing AI solutions that are informed by real-world medical needs, ensuring that advancements not only serve technical interests but also enhance healthcare practices and patient care.
Encouraging Continuous Learning
In a field as dynamic as medical artificial intelligence, continuous learning is not just beneficial; it is essential. The rapid evolution of technology necessitates that professionals remain abreast of new developments and methodologies. Thus, educational programs should instill a mindset of lifelong learning, encouraging learners to engage with ongoing advancements in AI technologies.
By embracing continuous learning, professionals can adapt to emerging challenges and opportunities in healthcare. This proactive approach enhances one's ability to leverage AI solutions, thereby improving efficiency and precision in medical practice. Continuous education also promotes an ethical understanding of AI applications, ensuring that practitioners use these technologies responsibly.
"Continuous learning in medical AI is an investment in the future of healthcare. It allows professionals to apply the latest techniques to improve patient outcomes while addressing ethical considerations."
"Continuous learning in medical AI is an investment in the future of healthcare. It allows professionals to apply the latest techniques to improve patient outcomes while addressing ethical considerations."
In summary, the conclusions drawn from this article highlight the importance of education in shaping the future of medical artificial intelligence. The integration of AI into healthcare is a complex endeavor that demands well-prepared professionals. Therefore, fostering an environment that promotes both education and continuous learning is imperative for those aspiring to make meaningful contributions in this field.